Estimating uniaxial compressive strength of peridotites from simple tests using neural networks
Arabian Journal of Geosciences(2021)
摘要
In mechanical rock projects, it is often required to determine the uniaxial compressive strength (UCS). For the direct estimation of this parameter, destructive tests of high-quality core specimens of proper geometry are needed. However, these tests are demanding, and sometimes, it is difficult to obtain suitable specimens from highly porous, highly fractured, weathered, or thinly bedded rocks. Therefore, a model predicting UCS through intact rock properties has become an attractive alternative method. Artificial neural networks (ANNs) are applied to estimate UCS through the physical, dynamic, and mechanical characteristics of various materials but not specifically for peridotites. In this study, the dry unit weight ( γ d ), effective porosity ( n e ), P-wave ultrasonic velocity ( V p ), the Schmidt rebound number (Srn), and the point load strength index (Is 50 ) are selected as input parameters, and by using neural networks techniques, some equations are developed and presented in order to estimate the UCS. The data was gathered from earlier experimental work, which has taken place in central Greece. The results obtained from neural network modeling are compared with those from previous studies on peridotites, and it is shown that the proposed ANN is a better predictive tool than the earlier multiple regression models. Due to their high prediction performance; ANN can, therefore, be used during the preliminary stages of rock engineering assessments and help to reduce the requirements for more expensive and difficult or destructive tests.
更多查看译文
关键词
Rock mechanic, Peridotites, Uniaxial compressive strength, Physicomechanical property, Artificial neural networks
AI 理解论文
溯源树
样例
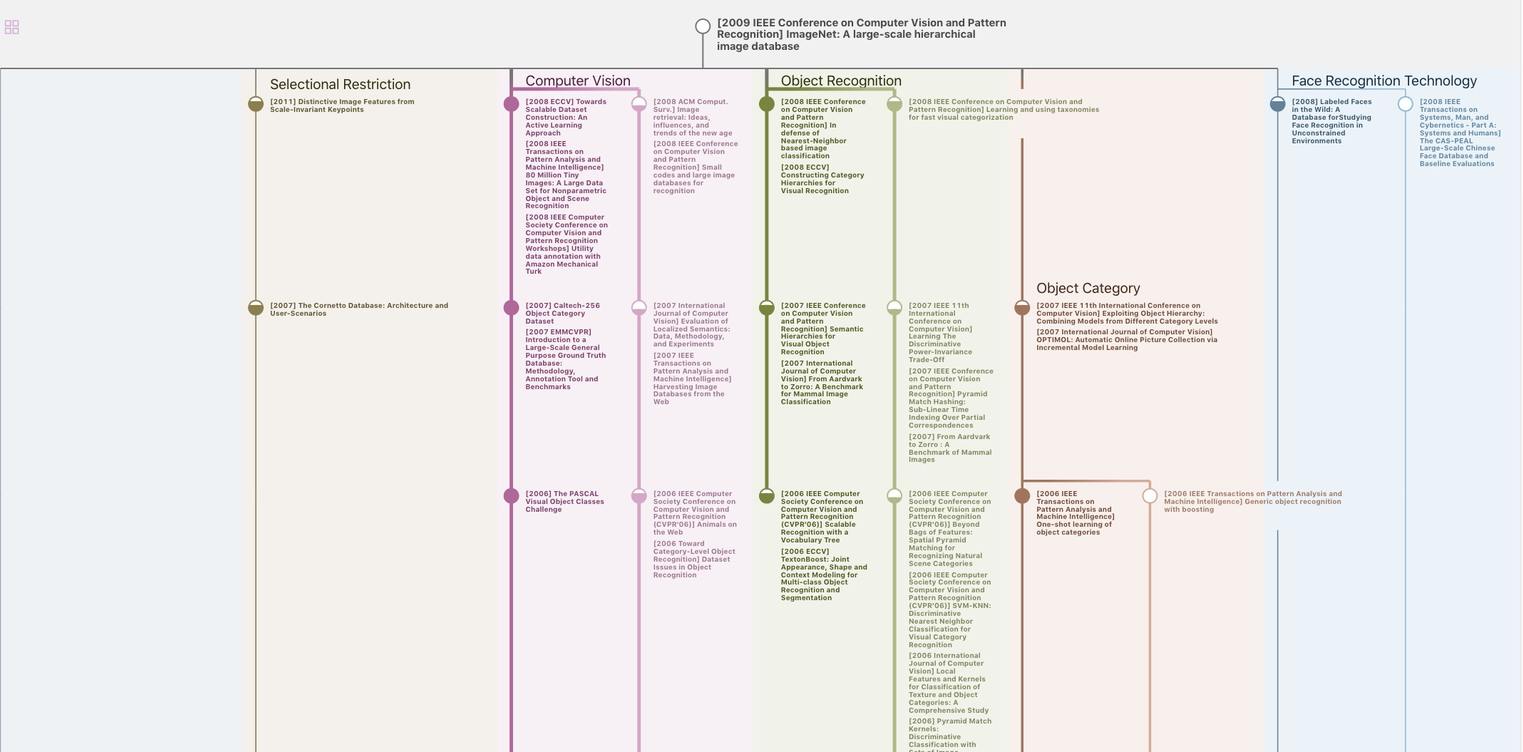
生成溯源树,研究论文发展脉络
Chat Paper
正在生成论文摘要