SeqDTW: A Segmentation Based Distance Measure for Time Series Data
Transactions of the Indian National Academy of Engineering(2021)
摘要
Computing the similarity between time series data is one of the core challenges frequently occurring in time series analysis across various disciplines. Particularly, in building energy management, there are many applications that need to cluster the time series with simultaneous rise, peak, similar patterns, pattern-average allowing small amplitude shift and small phase shift as one group. Commonly used distance measures like Euclidean and DTW being sensitive to phase shift or amplitude shift, are unable to identify similarity between two time series with simultaneous rise, peak and similar patterns. To address this problem, we propose a novel time series similarity measure, sub-sequence based DTW, SeqDTW based on time series segmentation approach. SeqDTW attempts to find best match of each subsequence in the segmented time series. Overall distance is the weighted aggregate of distance between the matching subsequences. In addition to existing monotonicity in DTW warping path, SeqDTW reduces the number of computations across the distance matrix and avoids singularity. An exhaustive experiment shows the superiority of the proposed method in finding time series having simultaneous rise, peak and similar patterns.
更多查看译文
关键词
Smart grids,Time series,Distance measures,Segmentation,Clustering
AI 理解论文
溯源树
样例
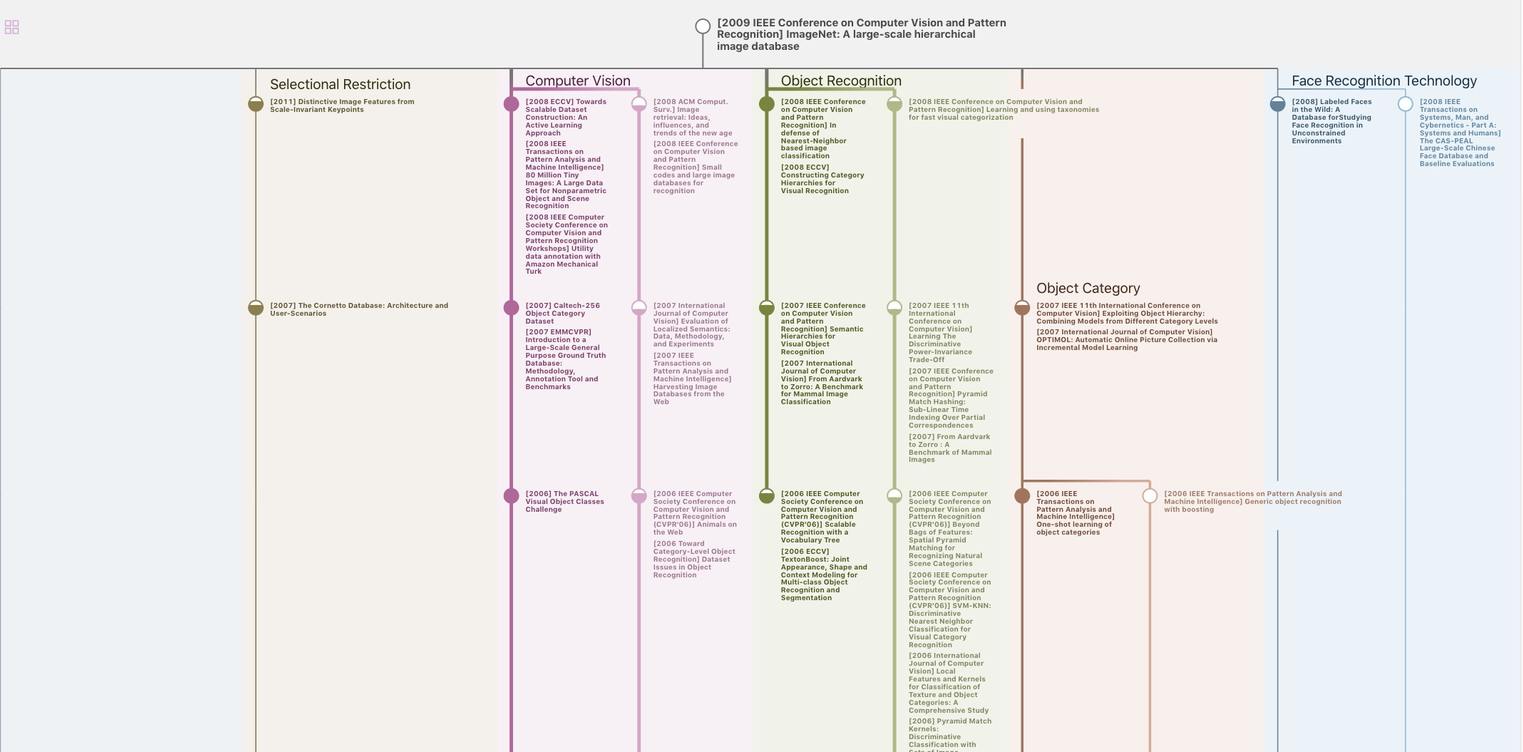
生成溯源树,研究论文发展脉络
Chat Paper
正在生成论文摘要