D2 dopamine receptor expression, sensitivity to rewards, and reinforcement learning in a complex value-based decision-making task
bioRxiv(2022)
摘要
In the basal ganglia, different dopamine subtypes have opposing dynamics at post-synaptic receptors, with the ratio of D1 to D2 receptors determining the relative sensitivity to gains and losses, respectively, during value-based learning. This effective sensitivity to reward feedback interacts with phasic dopamine levels to determine the effectiveness of learning, particularly in dynamic feedback situations where frequency and magnitude of rewards need to be integrated over time to make optimal decisions. Using both simulations and behavioral data in humans, we evaluated how reduced sensitivity to losses, relative to gains, leads to suboptimal learning in the Iowa Gambling Task (IGT), a complex value-learning task. In the behavioral data, we tested individuals with a variant of the human dopamine receptor D2 (DRD2; -141C Ins/Del and Del/Del) gene that associates with lower levels of D2 receptor expression (N=119) and compared their performance to non-carrier controls (N=319). The magnitude of the reward response was measured by looking at ventral striatal (VS) reactivity to rewards in the Cards task using fMRI. DRD2 variant carriers had generally lower performance in the IGT than non-carriers, consistent with reduced sensitivity to losses. There was also a positive association between VS reactivity and performance in the IGT, however, we found no statistically significant difference in this effect between DRD2 carriers and non-carriers. Thus, while reduced D2 receptor expression was associated with less efficient learning in the IGT, we did not find evidence for the moderation of this effect by the magnitude of the reward response.
### Competing Interest Statement
The authors have declared no competing interest.
更多查看译文
关键词
d2 dopamine receptor expression,reinforcement learning,decision-making decision-making,rewards,value-based
AI 理解论文
溯源树
样例
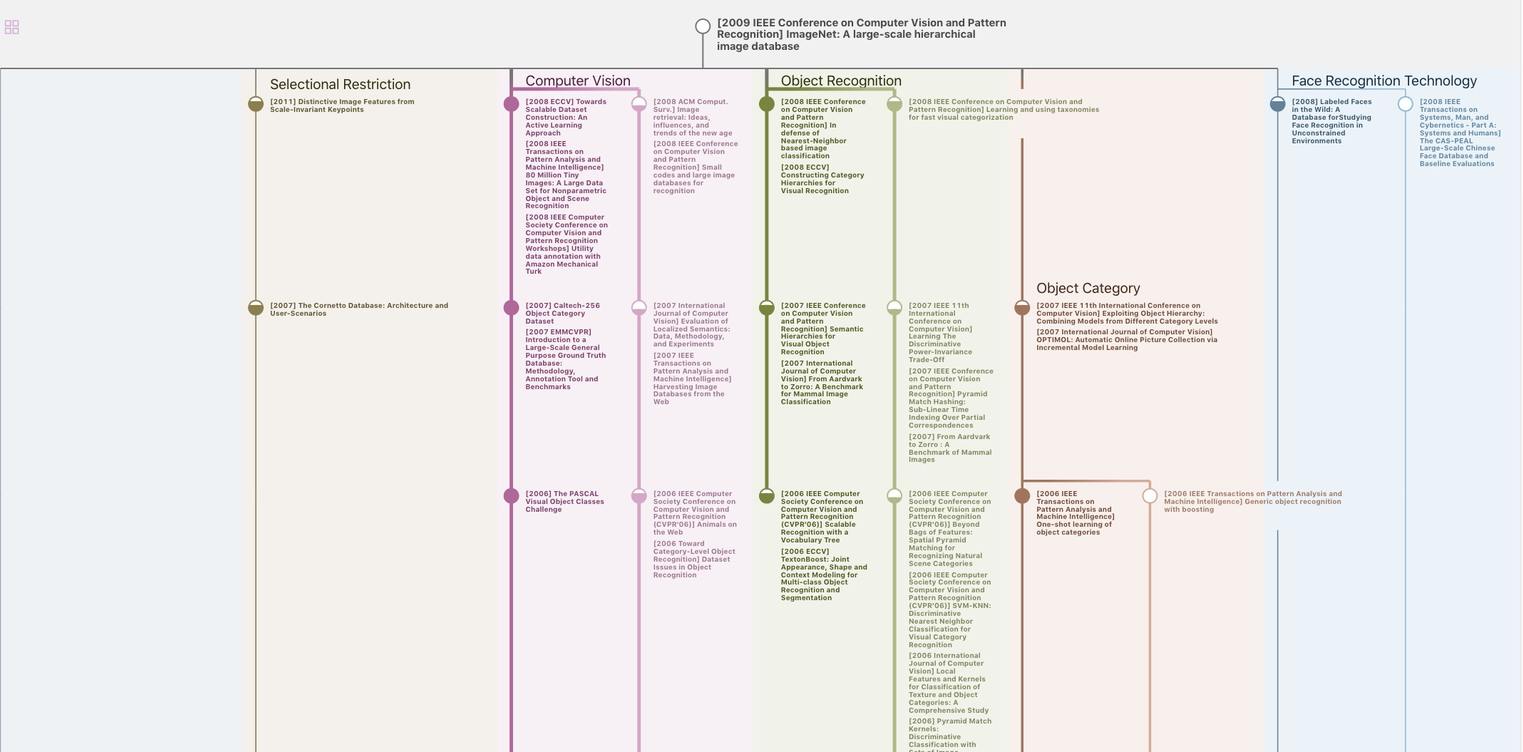
生成溯源树,研究论文发展脉络
Chat Paper
正在生成论文摘要