dialogi: Utilising NLP With Chemical and Disease Similarities to Drive the Identification of Drug-Induced Liver Injury Literature
FRONTIERS IN GENETICS(2022)
摘要
Drug-Induced Liver Injury (DILI), despite its low occurrence rate, can cause severe side effects or even lead to death. Thus, it is one of the leading causes for terminating the development of new, and restricting the use of already-circulating, drugs. Moreover, its multifactorial nature, combined with a clinical presentation that often mimics other liver diseases, complicate the identification of DILI-related (or "positive") literature, which remains the main medium for sourcing results from the clinical practice and experimental studies. This work-contributing to the "Literature AI for DILI Challenge" of the Critical Assessment of Massive Data Analysis (CAMDA) 2021- presents an automated pipeline for distinguishing between DILI-positive and negative publications. We used Natural Language Processing (NLP) to filter out the uninformative parts of a text, and identify and extract mentions of chemicals and diseases. We combined that information with small-molecule and disease embeddings, which are capable of capturing chemical and disease similarities, to improve classification performance. The former were directly sourced from the Chemical Checker (CC). For the latter, we collected data that encode different aspects of disease similarity from the National Library of Medicine's (NLM) Medical Subject Headings (MeSH) thesaurus and the Comparative Toxicogenomics Database (CTD). Following a similar procedure as the one used in the CC, vector representations for diseases were learnt and evaluated. Two Neural Network (NN) classifiers were developed: a baseline model that accepts texts as input and an augmented, extended, model that also utilises chemical and disease embeddings. We trained, validated, and tested the classifiers through a Nested Cross-Validation (NCV) scheme with 10 outer and 5 inner folds. During this, the baseline and extended models performed virtually identically, with F-1-scores of 95.04 +/- 0.61% and 94.80 +/- 0.41%, respectively. Upon validation on an external, withheld, dataset that is meant to assess classifier generalisability, the extended model achieved an F-1-score of 91.14 +/- 1.62%, outperforming its baseline counterpart which received a lower score of 88.30 +/- 2.44%. We make further comparisons between the classifiers and discuss future improvements and directions, including utilising chemical and disease embeddings for visualisation and exploratory analysis of the DILI-positive literature.
更多查看译文
关键词
drug-induced liver injury (DILI, ), natural language processing (NLP, neural network, disease similarity, drug similarity
AI 理解论文
溯源树
样例
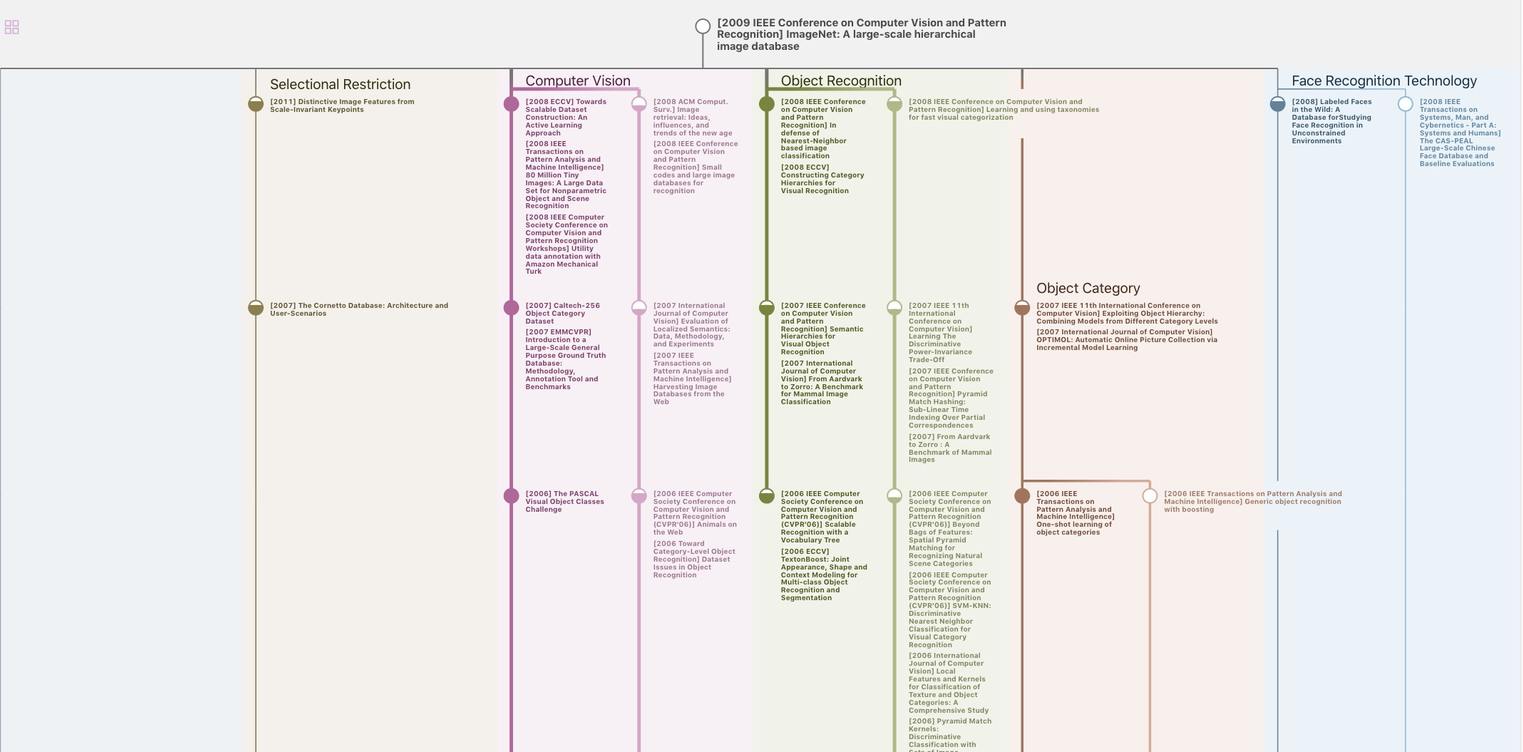
生成溯源树,研究论文发展脉络
Chat Paper
正在生成论文摘要