ShapeNet: Shape constraint for galaxy image deconvolution
ASTRONOMY & ASTROPHYSICS(2022)
摘要
Deep learning (DL) has shown remarkable results in solving inverse problems in various domains. In particular, the Tikhonet approach is very powerful in deconvolving optical astronomical images. However, this approach only uses the l(2) loss, which does not guarantee the preservation of physical information (e.g., flux and shape) of the object that is reconstructed in the image. A new loss function has been proposed in the framework of sparse deconvolution that better preserves the shape of galaxies and reduces the pixel error. In this paper, we extend the Tikhonet approach to take this shape constraint into account and apply our new DL method, called ShapeNet, to a simulated optical and radio-interferometry dataset. The originality of the paper relies on i) the shape constraint we use in the neural network framework, ii) the application of DL to radio-interferometry image deconvolution for the first time, and iii) the generation of a simulated radio dataset that we make available for the community. A range of examples illustrates the results.
更多查看译文
关键词
miscellaneous, radio continuum, galaxies, techniques, image processing, methods, data analysis, methods, numerical
AI 理解论文
溯源树
样例
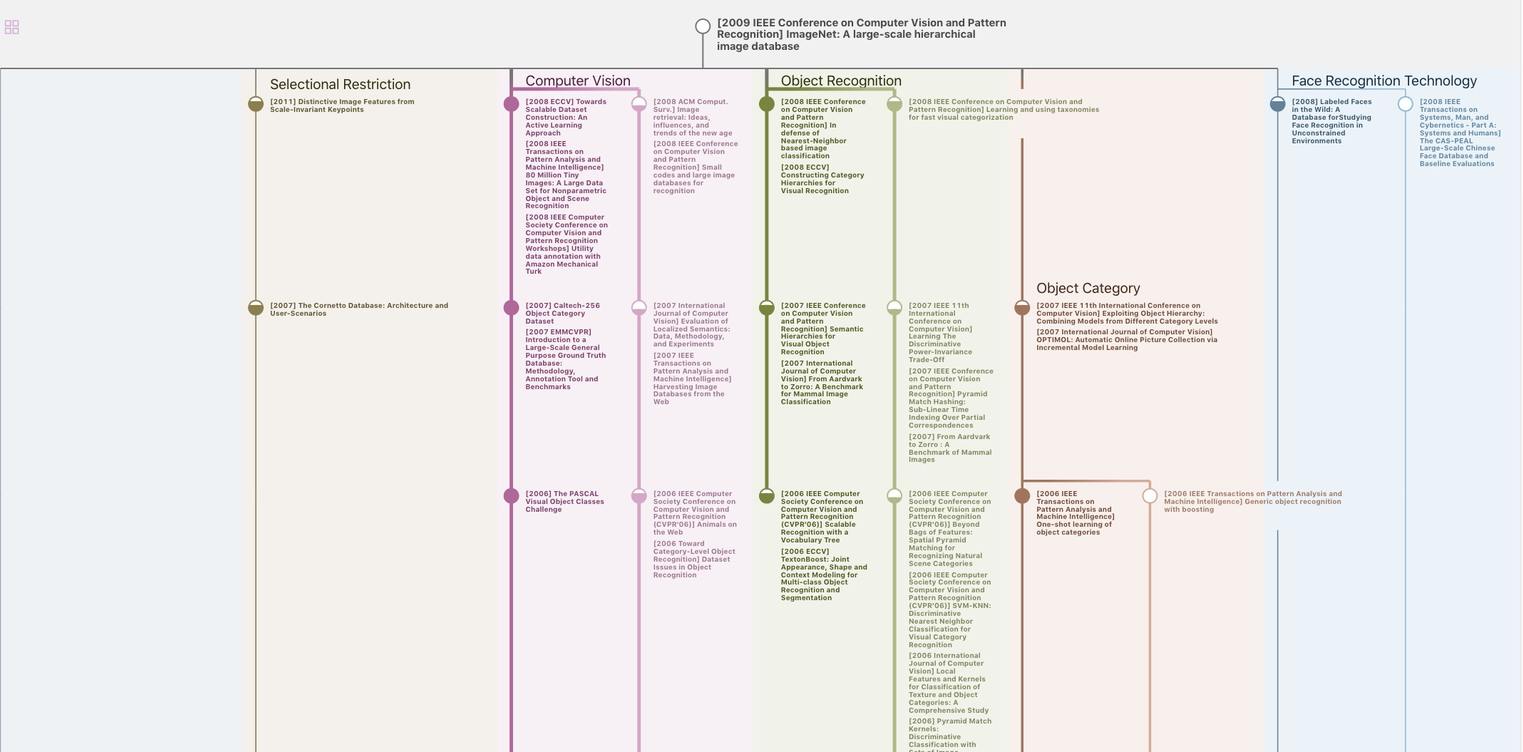
生成溯源树,研究论文发展脉络
Chat Paper
正在生成论文摘要