Oversampling based on WGAN for Network Threat Detection
2021 IEEE INTL CONF ON DEPENDABLE, AUTONOMIC AND SECURE COMPUTING, INTL CONF ON PERVASIVE INTELLIGENCE AND COMPUTING, INTL CONF ON CLOUD AND BIG DATA COMPUTING, INTL CONF ON CYBER SCIENCE AND TECHNOLOGY CONGRESS DASC/PICOM/CBDCOM/CYBERSCITECH 2021(2021)
Abstract
Class imbalance is a common problem on network threat detection. The data of network threat usually are few and viewed as the minority class. Wasserstein GAN (WGAN) as a generative method can solve the imbalanced problem through oversampling. In this work, we use the shallow learning and the deep learning methods to build a network threat detection model on the imbalanced data. First, the imbalanced data are divided into the training data set and testing data set. Second, WGAN is used to generate the new minority samples for the training data. Then the generated data are fused to the original training data to form a balanced training data set. Third, the balanced training data set is input to the shallow learning methods to train the network threat detection model. Next, the imbalanced testing data set is input to the trained model to distinguish the network threat. The experimental results show that our network threat detection model based on WGAN for oversampling achieves a good performance for network threat detection.
MoreTranslated text
Key words
network threat detection,Wasserstein Generative adversarial networks,shallow Learning,deep learning
AI Read Science
Must-Reading Tree
Example
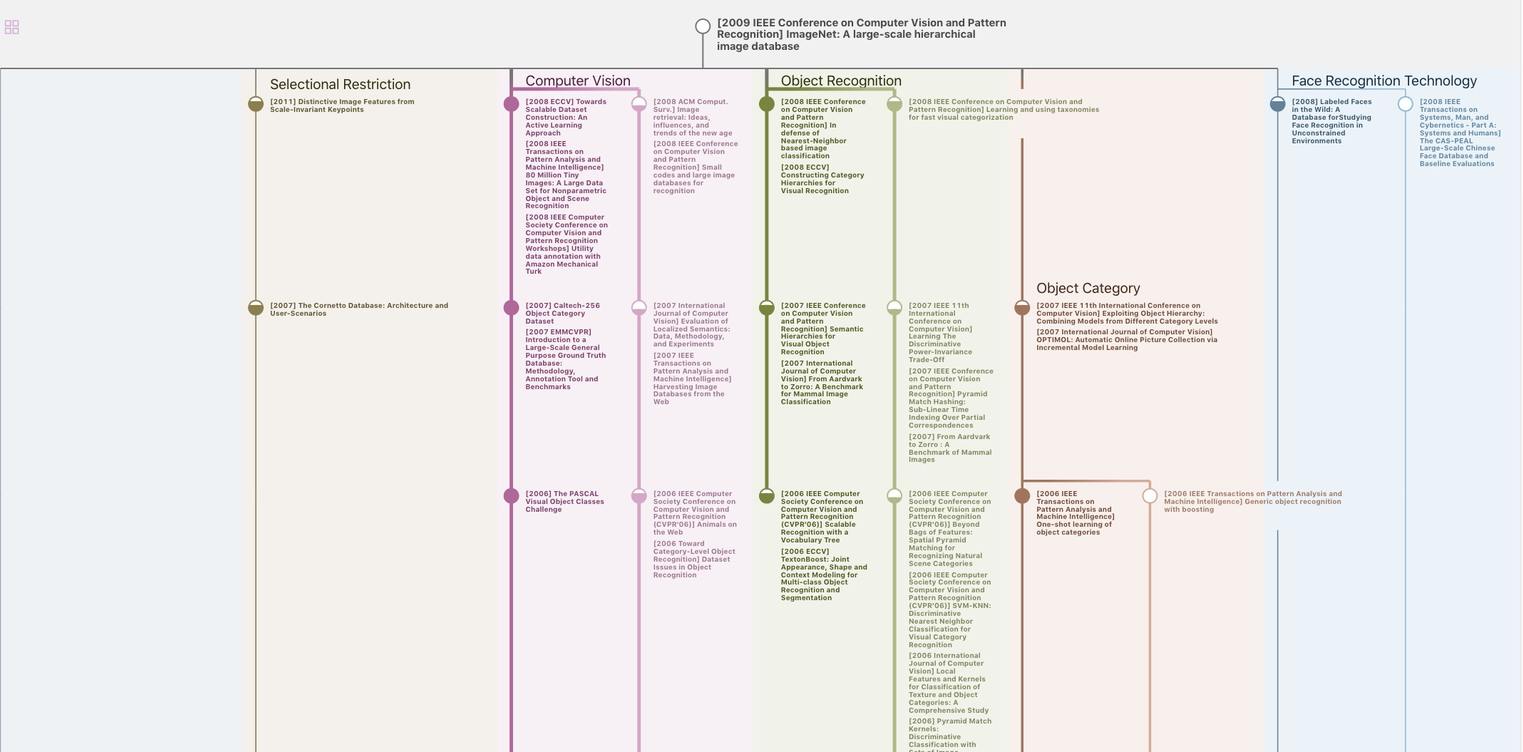
Generate MRT to find the research sequence of this paper
Chat Paper
Summary is being generated by the instructions you defined