Dynamic Programming Through the Lens of Semismooth Newton-Type Methods
IEEE control systems letters(2022)
摘要
Policy iteration and value iteration are at the core of many (approximate) dynamic programming methods. For Markov Decision Processes with finite state and action spaces, we show that they are instances of semismooth Newton-type methods for solving the Bellman equation. In particular, we prove that policy iteration is equivalent to the exact semismooth Newton method and enjoys a local quadratic convergence rate. This finding is corroborated by extensive numerical evidence in the fields of control and operations research, which confirms that policy iteration generally requires relatively few iterations to achieve convergence even in presence of a large number of admissible policies. We then show that value iteration is an instance of the fixed-point iteration method and develop a locally accelerated version of value iteration with global convergence guarantees and negligible extra computational costs.
更多查看译文
关键词
Costs,Convergence,Jacobian matrices,Newton method,Dynamic programming,Markov processes,Cost function,Dynamic programming,semismooth Newton-type methods
AI 理解论文
溯源树
样例
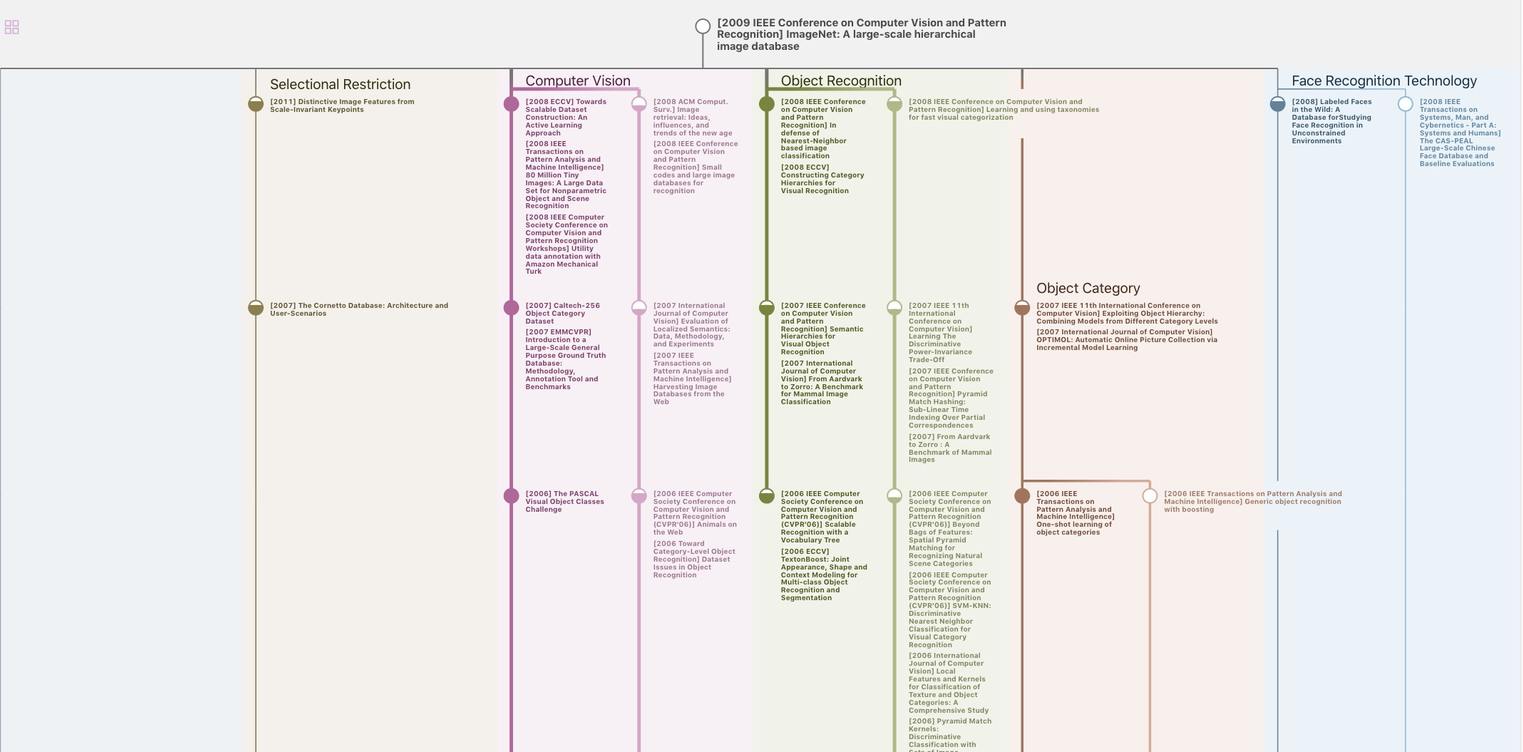
生成溯源树,研究论文发展脉络
Chat Paper
正在生成论文摘要