Comparison and evaluation of data-driven protein stability prediction models
biorxiv(2022)
摘要
Predicting protein stability is important to protein engineering yet poses unsolved challenges. Computational costs associated with physics-based models, and the limited amount of data available to support data-driven models, have left stability prediction behind the prediction of structure. New data and advancements in modeling approaches now afford greater opportunities to solve this challenge. We evaluate a set of data-driven prediction models using a large, newly published dataset of various synthetic proteins and their experimental stability data. We test the models in two separate tasks, exercising extrapolation to new protein classes and prediction of the effects on stability of small mutations. Results: Small convolutional neural networks trained from scratch on stability data and large protein embedding models passed through simple downstream models trained on stability data are both able to predict stability comparably well. The largest of the embedding models yields the best performance in all tasks and metrics. We also explored the marginal performance gains seen with two ensemble models.
### Competing Interest Statement
The authors have declared no competing interest.
更多查看译文
关键词
protein,stability,prediction,data-driven
AI 理解论文
溯源树
样例
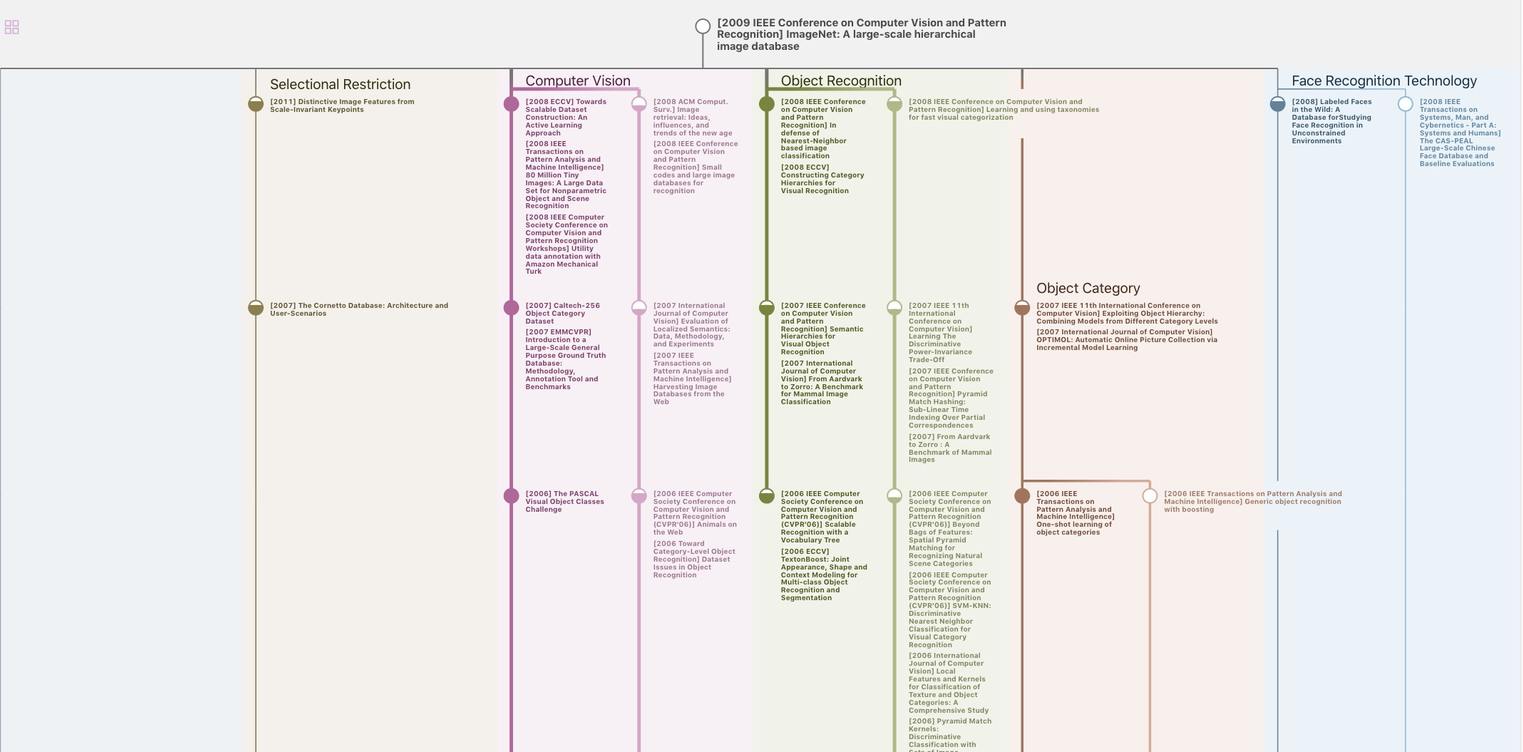
生成溯源树,研究论文发展脉络
Chat Paper
正在生成论文摘要