PLANET: Dynamic Content Planning in Autoregressive Transformers for Long-form Text Generation
PROCEEDINGS OF THE 60TH ANNUAL MEETING OF THE ASSOCIATION FOR COMPUTATIONAL LINGUISTICS (ACL 2022), VOL 1: (LONG PAPERS)(2022)
摘要
Despite recent progress of pre-trained language models on generating fluent text, existing methods still suffer from incoherence problems in long-form text generation tasks that require proper content control and planning to form a coherent high-level logical flow. In this work, we propose PLANET, a novel generation framework leveraging autoregressive self-attention mechanism to conduct content planning and surface realization dynamically. To guide the generation of output sentences, our framework enriches the Transformer decoder with latent representations to maintain sentence-level semantic plans grounded by bag-of-words. Moreover, we introduce a new coherence-based contrastive learning objective to further improve the coherence of output. Extensive experiments are conducted on two challenging longform text generation tasks including counter-argument generation and opinion article generation. Both automatic and human evaluations show that our method significantly outperforms strong baselines and generates more coherent texts with richer contents.
更多查看译文
关键词
dynamic content planning,autoregressive transformers,text,long-form
AI 理解论文
溯源树
样例
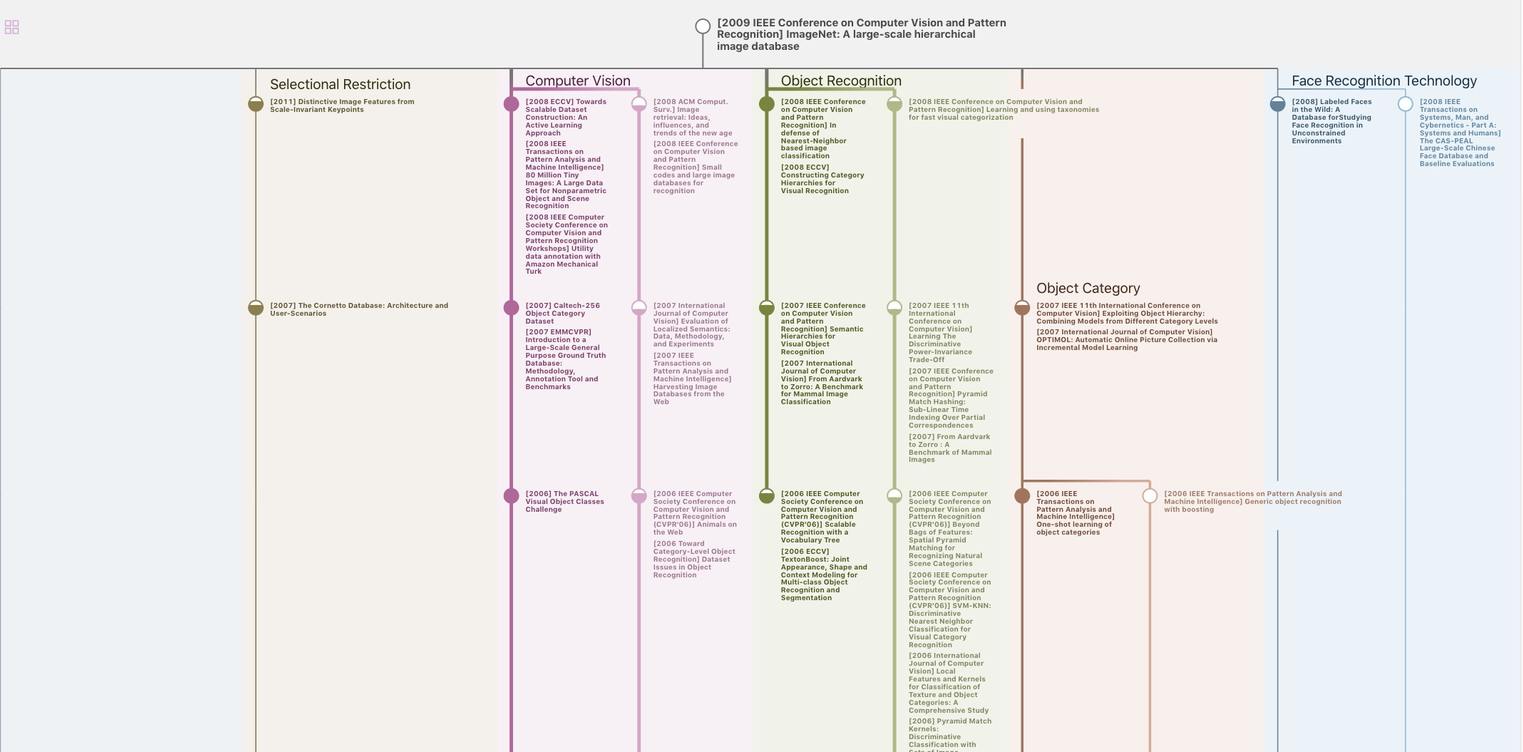
生成溯源树,研究论文发展脉络
Chat Paper
正在生成论文摘要