Probabilistic Brain Extraction in MR Images via Conditional Generative Adversarial Networks
IEEE TRANSACTIONS ON MEDICAL IMAGING(2024)
摘要
Brain extraction, or the task of segmenting the brain in MR images, forms an essential step for many neuroimaging applications. These include quantifying brain tissue volumes, monitoring neurological diseases, and estimating brain atrophy. Several algorithms have been proposed for brain extraction, including image-to-image deep learning methods that have demonstrated significant gains in accuracy. However, none of them account for the inherent uncertainty in brain extraction. Motivated by this, we propose a novel, probabilistic deep learning algorithm for brain extraction that recasts this task as a Bayesian inference problem and utilizes a conditional generative adversarial network (cGAN) to solve it. The input to the cGAN's generator is an MR image of the head, and the output is a collection of likely brain images drawn from a probability density conditioned on the input. These images are used to generate a pixel-wise mean image, serving as the estimate for the extracted brain, and a standard deviation image, which quantifies the uncertainty in the prediction. We test our algorithm on head MR images from five datasets: NFBS, CC359, LPBA, IBSR, and their combination. Our datasets are heterogeneous regarding multiple factors, including subjects (with and without symptoms), magnetic field strengths, and manufacturers. Our experiments demonstrate that the proposed approach is more accurate and robust than a widely used brain extraction tool and at least as accurate as the other deep learning methods. They also highlight the utility of quantifying uncertainty in downstream applications.
更多查看译文
关键词
Biomedical imaging,Task analysis,Brain modeling,Image segmentation,Head,Brain,Uncertainty,Bayesian inference,brain extraction,conditional generative adversarial networks,medical imaging,neuroimaging,skull stripping,uncertainty quantification
AI 理解论文
溯源树
样例
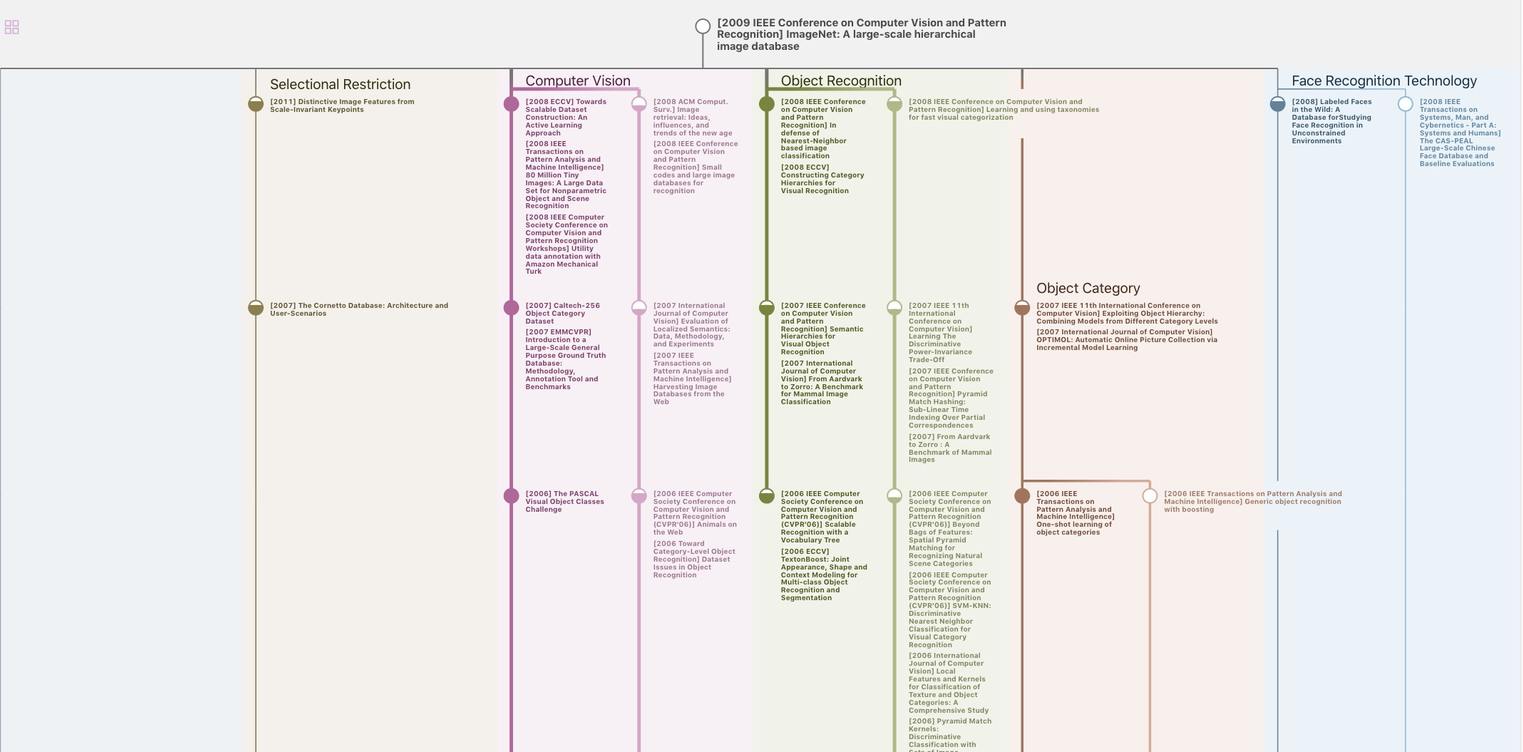
生成溯源树,研究论文发展脉络
Chat Paper
正在生成论文摘要