Speeding up the Topography Imaging of Atomic Force Microscopy by Convolutional Neural Network
ANALYTICAL CHEMISTRY(2022)
摘要
Atomic force microscopy (AFM) provides unprecedented insight into surface topography research with ultrahigh spatial resolution at the subnanometer level. However, a slow scanning rate has to be employed to ensure the image quality, which will largely increase the accumulated sample drift, thereby, resulting in the low fidelity of the AFM image. In this paper, we propose a fast imaging method which performs a complete fast Raster scanning and a slow mu-path subsampling together with a deep learning algorithm to rapidly produce an AFM image with high quality andsmall drift. A supervised convolutional neural network (CNN) model is trained withthe slow mu-path subsampled data and its counterpart acquired with fast Raster scan.The fast speed acquired AFM image is then inputted to the well-trained CNN modelto output the high quality one. We validate the reliability of this method using a silicongrids sample and further apply it to the fast imaging of a vanadium dioxide thinfilm.The results demonstrate that this method can largely improve the imaging speed up to 10.3 times with state-of-the-art imagingquality, and reduce the sample drift by 8.9 times in the multiframe AFM imaging of the same area. Furthermore, we prove that thismethod is also applicable to other scanning imaging techniques such as scanning electrochemical microscopy
更多查看译文
AI 理解论文
溯源树
样例
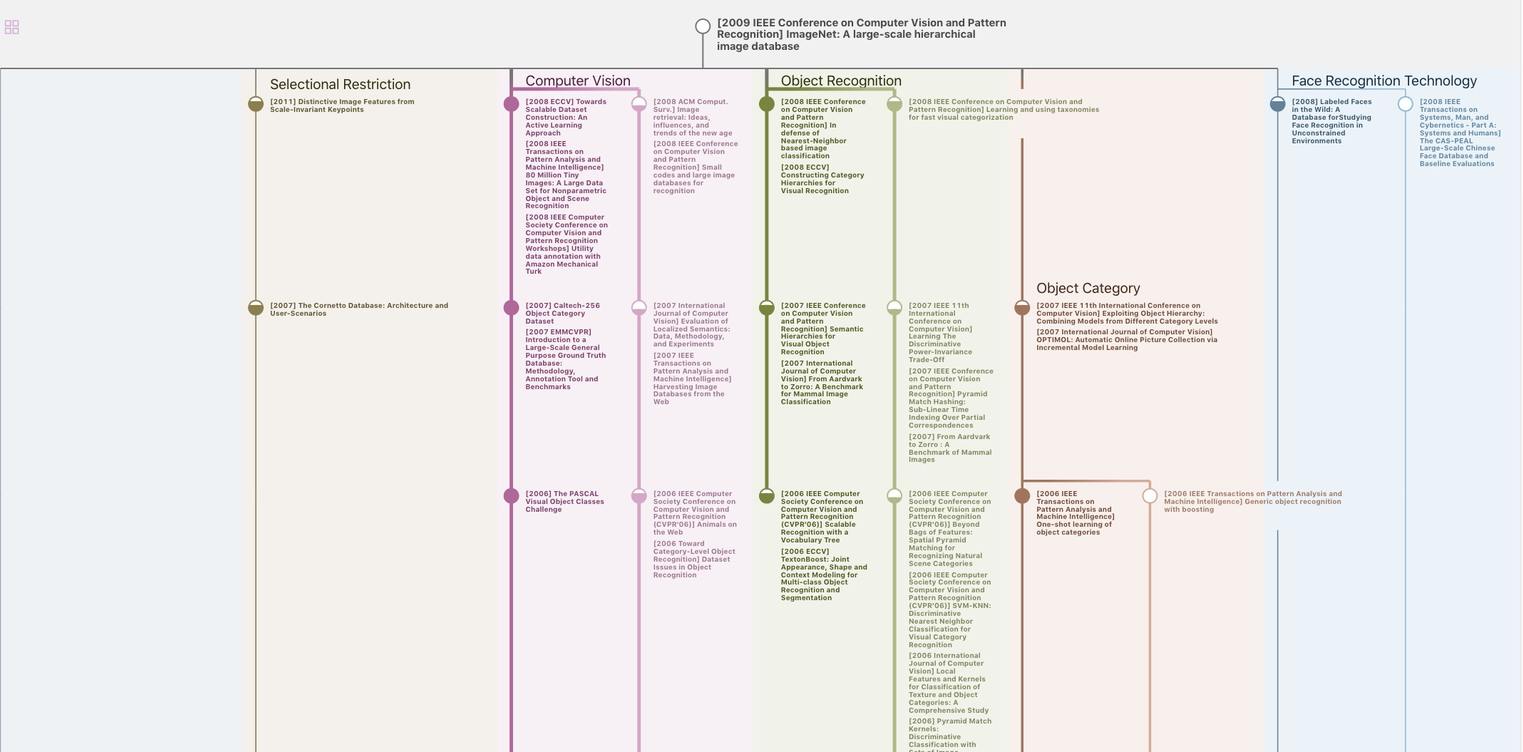
生成溯源树,研究论文发展脉络
Chat Paper
正在生成论文摘要