Detecting time lag between a pair of time series using visibility graph algorithm.
Communications in statistics. Case studies, data analysis and applications(2021)
摘要
Estimating the time lag between a pair of time series is of significance in many practical applications. In this article, we introduce a method to quantify such lags by adapting the visibility graph algorithm, which converts time series into a mathematical graph. Currently widely used method to detect such lags is based on cross-correlations, which has certain limitations. We present simulated examples where the new method identifies the lag correctly and unambiguously while as the cross-correlation method does not. The article includes results from an extensive simulation study conducted to better understand the scenarios where the new method performed better or worse than the existing approach. We also present a likelihood based parametric modeling framework and consider frameworks for quantifying uncertainty and hypothesis testing for the new approach. We apply the current and new methods to two case studies, one from neuroscience and the other from environmental epidemiology, to illustrate the methods further.
更多查看译文
关键词
Time series,correlogram,cross correlation,environmental epidemiology,local field potentials,neuroscience,ozone levels,time lag,transfer function,visibility graph algorithm
AI 理解论文
溯源树
样例
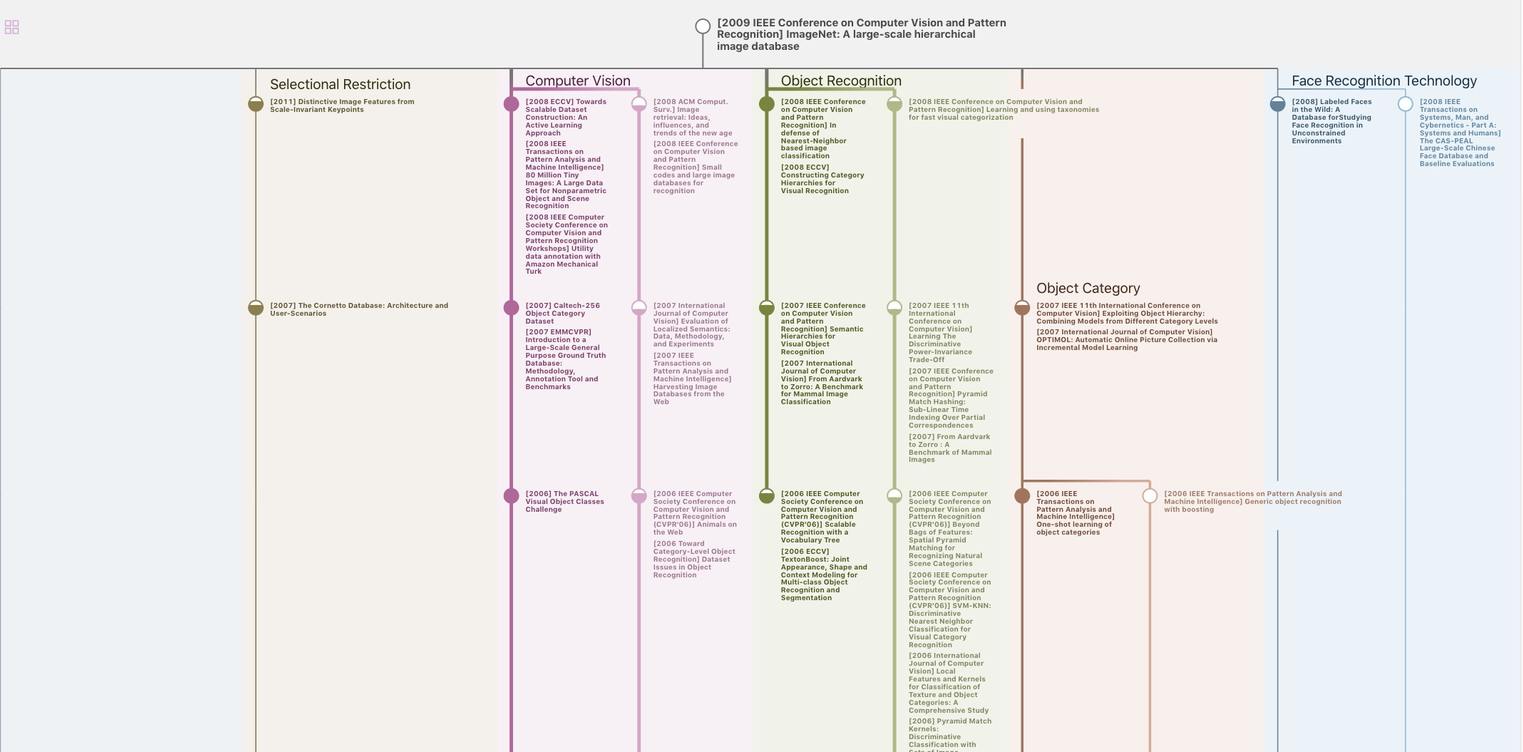
生成溯源树,研究论文发展脉络
Chat Paper
正在生成论文摘要