Deep Transferring Quantization
European Conference on Computer Vision(2020)
摘要
Network quantization is an effective method for network compression. Existing methods train a low-precision network by fine-tuning from a pre-trained model. However, training a low-precision network often requires large-scale labeled data to achieve superior performance. In many real-world scenarios, only limited labeled data are available due to expensive labeling costs or privacy protection. With limited training data, fine-tuning methods may suffer from the overfitting issue and substantial accuracy loss. To alleviate these issues, we introduce transfer learning into network quantization to obtain an accurate low-precision model. Specifically, we propose a method named deep transferring quantization (DTQ) to effectively exploit the knowledge in a pre-trained full-precision model. To this end, we propose a learnable attentive transfer module to identify the informative channels for alignment. In addition, we introduce the Kullback-Leibler (KL) divergence to further help train a low-precision model. Extensive experiments on both image classification and face recognition demonstrate the effectiveness of DTQ.
更多查看译文
关键词
Quantization, Deep transfer, Knowledge distillation
AI 理解论文
溯源树
样例
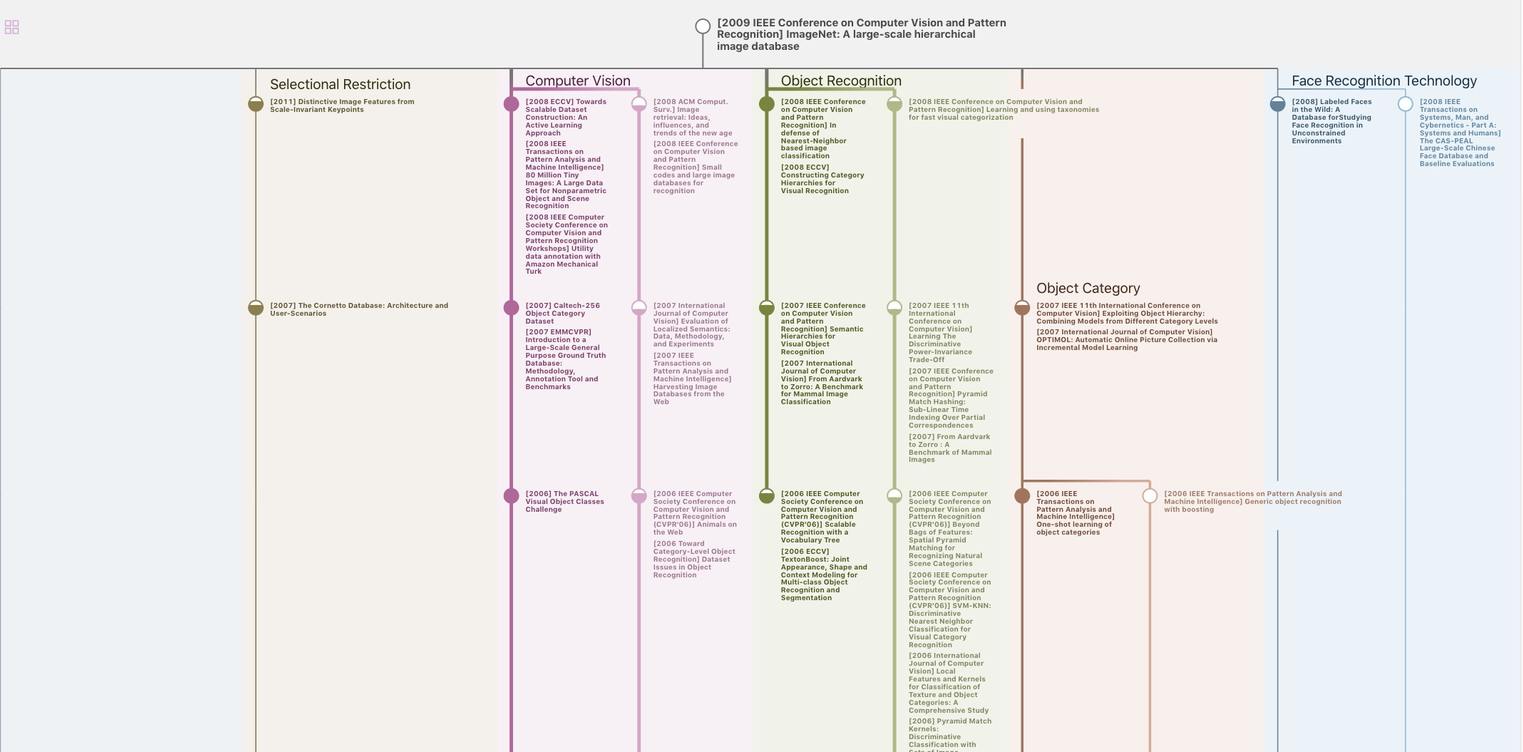
生成溯源树,研究论文发展脉络
Chat Paper
正在生成论文摘要