FedAGCN: A traffic flow prediction framework based on federated learning and Asynchronous Graph Convolutional Network
Appl. Soft Comput.(2023)
摘要
Accurate and real-time traffic flow prediction is an essential component of the Intelligent Transportation System (ITS). Balancing the prediction accuracy and time cost of prediction models is a challenging topic. This paper proposes a deep learning framework (FedAGCN) based on federated learning and asynchronous graph convolutional networks to predict traffic flow accurately in real time. FedAGCN applies asynchronous spatial-temporal graph convolution to model the spatial-temporal dependence in traffic data. In order to reduce the time cost of the deep learning model, we propose a graph federated learning strategy GraphFed to train the model. Experiments were conducted on two public traffic datasets, and the results showed that FedAGCN effectively reduced the training and inference time of the model while maintaining considerable prediction accuracy.& COPY; 2023 Elsevier B.V. All rights reserved.
更多查看译文
关键词
Traffic flow prediction,Graph convolutional network,Asynchronous spatial-temporal correlation,Federated learning
AI 理解论文
溯源树
样例
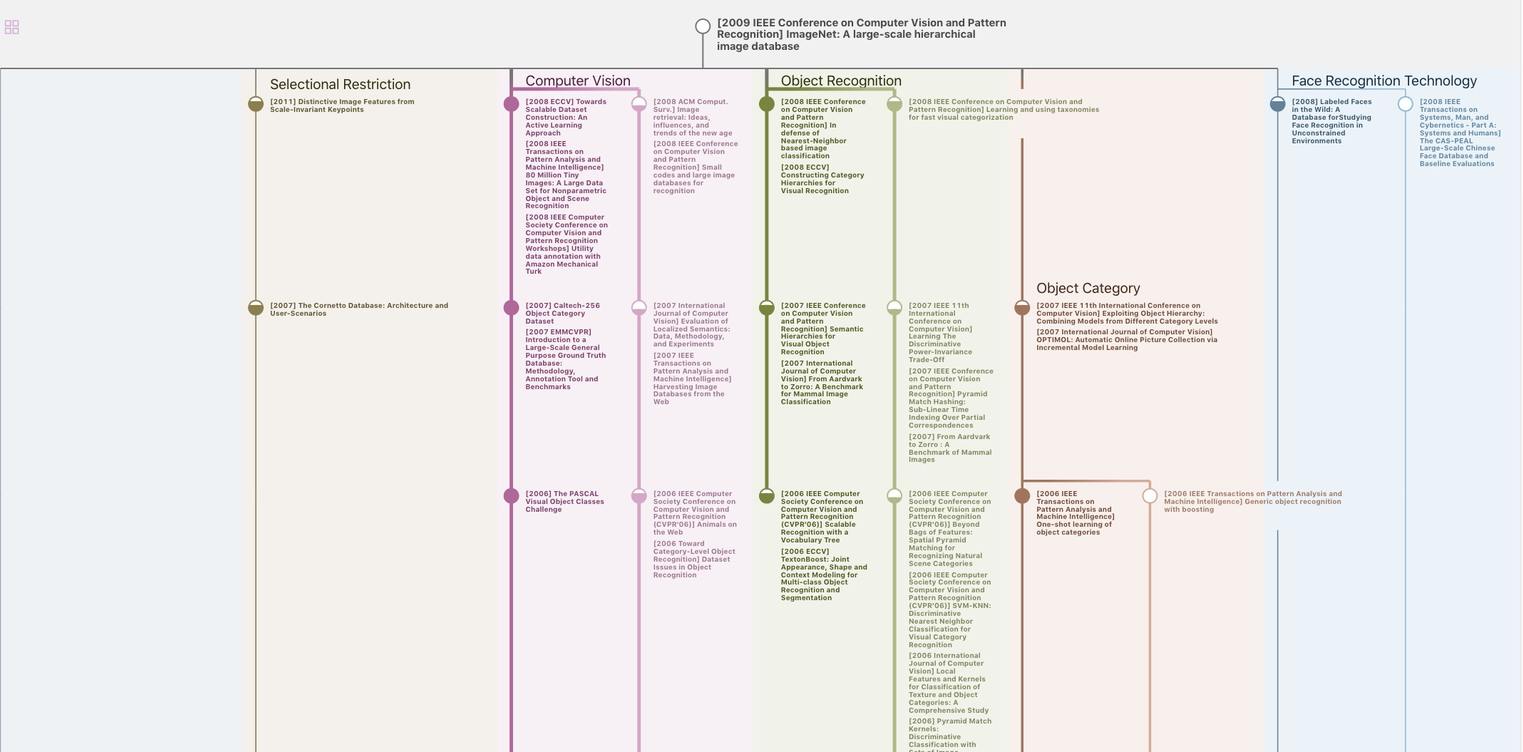
生成溯源树,研究论文发展脉络
Chat Paper
正在生成论文摘要