Enhanced Temporal Knowledge Embeddings with Contextualized Language Representations
arxiv(2022)
摘要
World knowledge exists in both structured (tables, knowledge graphs) and unstructured forms (texts). Recently, there have been extensive research efforts in the integration of structured factual knowledge and unstructured textual knowledge. However, most studies focus on incorporating static factual knowledge into pre-trained language models, while there is less work on enhancing temporal knowledge graph embedding using textual knowledge. Existing integration approaches can not apply to temporal knowledge graphs (tKGs) since they often assume knowledge embedding is time-invariant. In fact, the entity embedding in tKG embedding models usually evolves over time, which poses the challenge of aligning temporally relevant textual information with entities. To this end, we propose Enhanced Temporal Knowledge Embeddings with Contextualized Language Representations (ECOLA), which uses tKG quadruple as an implicit measure to temporally align textual data and the time-evolving entity representations and uses a novel knowledge-text prediction task to inject textual information into temporal knowledge embedding. ECOLA jointly optimizes the knowledge-text prediction objective and the temporal knowledge embedding objective, and thus, can simultaneously take full advantage of textual and structured knowledge. Since existing datasets do not provide tKGs with aligned textual data, we introduce three new datasets for training and evaluating ECOLA. Experimental results on the temporal knowledge graph completion task show that ECOLA outperforms state-of-the-art tKG embedding models by a large margin.
更多查看译文
关键词
structured and unstructured knowledge integration,temporal knowledge embedding,pre-trained language model,temporal reasoning
AI 理解论文
溯源树
样例
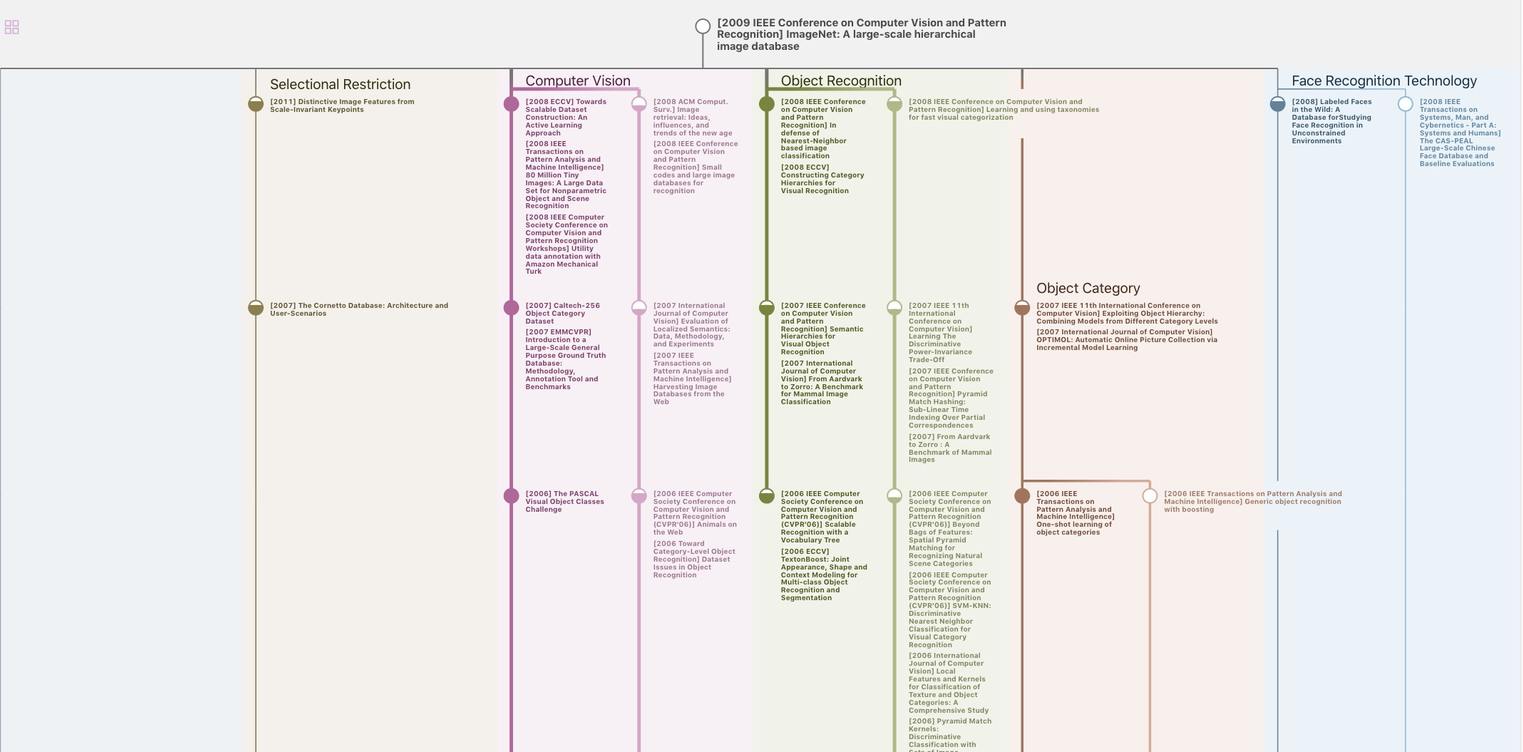
生成溯源树,研究论文发展脉络
Chat Paper
正在生成论文摘要