Modeling Intensification for Sign Language Generation: A Computational Approach
FINDINGS OF THE ASSOCIATION FOR COMPUTATIONAL LINGUISTICS (ACL 2022)(2022)
摘要
End-to-end sign language generation models do not accurately represent the prosody in sign language. A lack of temporal and spatial variations leads to poor-quality generated presentations that confuse human interpreters. In this paper, we aim to improve the prosody in generated sign languages by modeling intensification in a data-driven manner. We present different strategies grounded in linguistics of sign language that inform how intensity modifiers can be represented in gloss annotations. To employ our strategies, we first annotate a subset of the benchmark PHOENIX-14T, a German Sign Language dataset, with different levels of intensification. We then use a supervised intensity tagger to extend the annotated dataset and obtain labels for the remaining portion of it. This enhanced dataset is then used to train state-of-the-art transformer models for sign language generation. We find that our efforts in intensification modeling yield better results when evaluated with automatic metrics. Human evaluation also indicates a higher preference of the videos generated using our model.
更多查看译文
关键词
sign language generation,intensification,modeling,computational approach
AI 理解论文
溯源树
样例
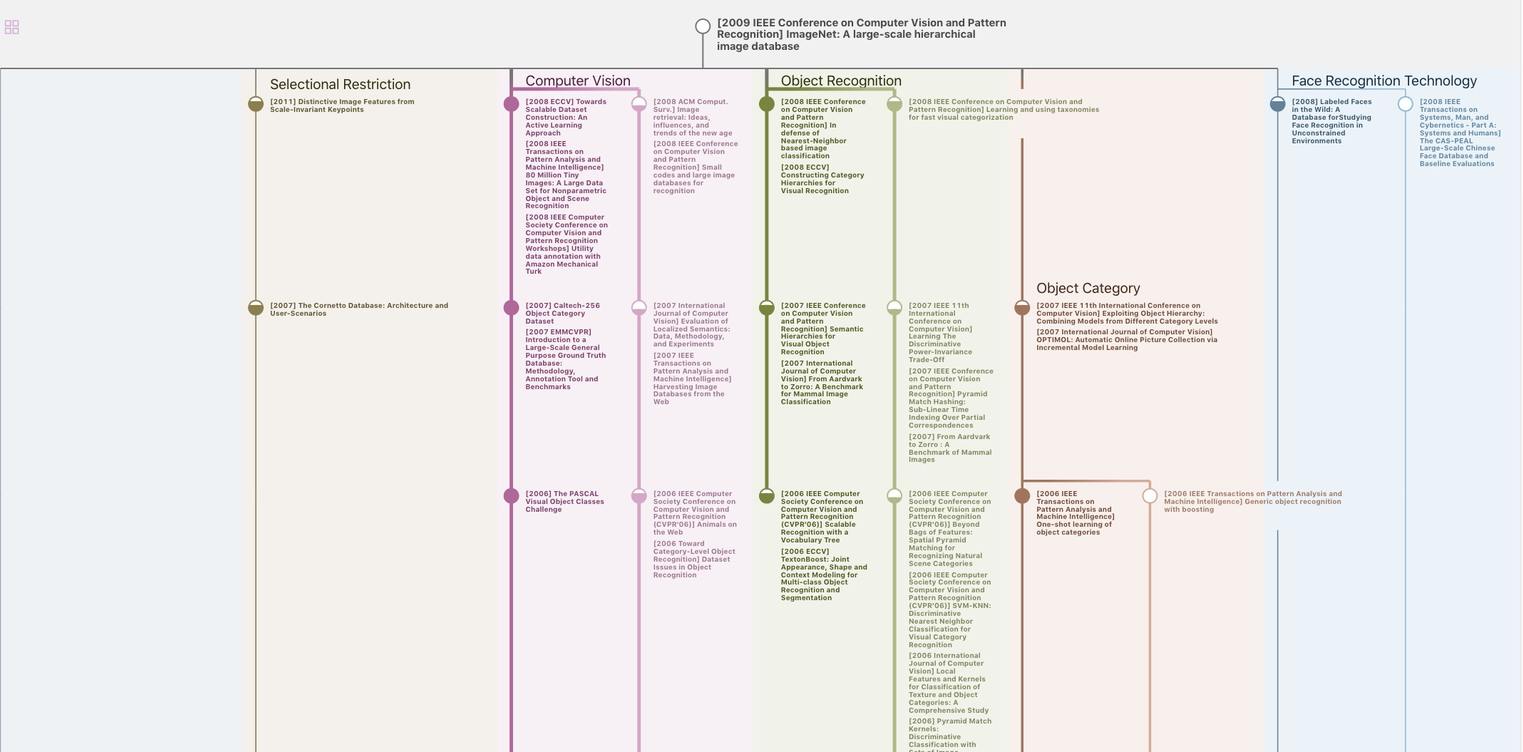
生成溯源树,研究论文发展脉络
Chat Paper
正在生成论文摘要