Fair Federated Learning via Bounded Group Loss
arxiv(2022)
摘要
Fair prediction across protected groups is an important constraint for many federated learning applications. However, prior work studying group fair federated learning lacks formal convergence or fairness guarantees. In this work we propose a general framework for provably fair federated learning. In particular, we explore and extend the notion of Bounded Group Loss as a theoretically-grounded approach for group fairness. Using this setup, we propose a scalable federated optimization method that optimizes the empirical risk under a number of group fairness constraints. We provide convergence guarantees for the method as well as fairness guarantees for the resulting solution. Empirically, we evaluate our method across common benchmarks from fair ML and federated learning, showing that it can provide both fairer and more accurate predictions than baseline approaches.
更多查看译文
关键词
Federated Learning,Group Fairness
AI 理解论文
溯源树
样例
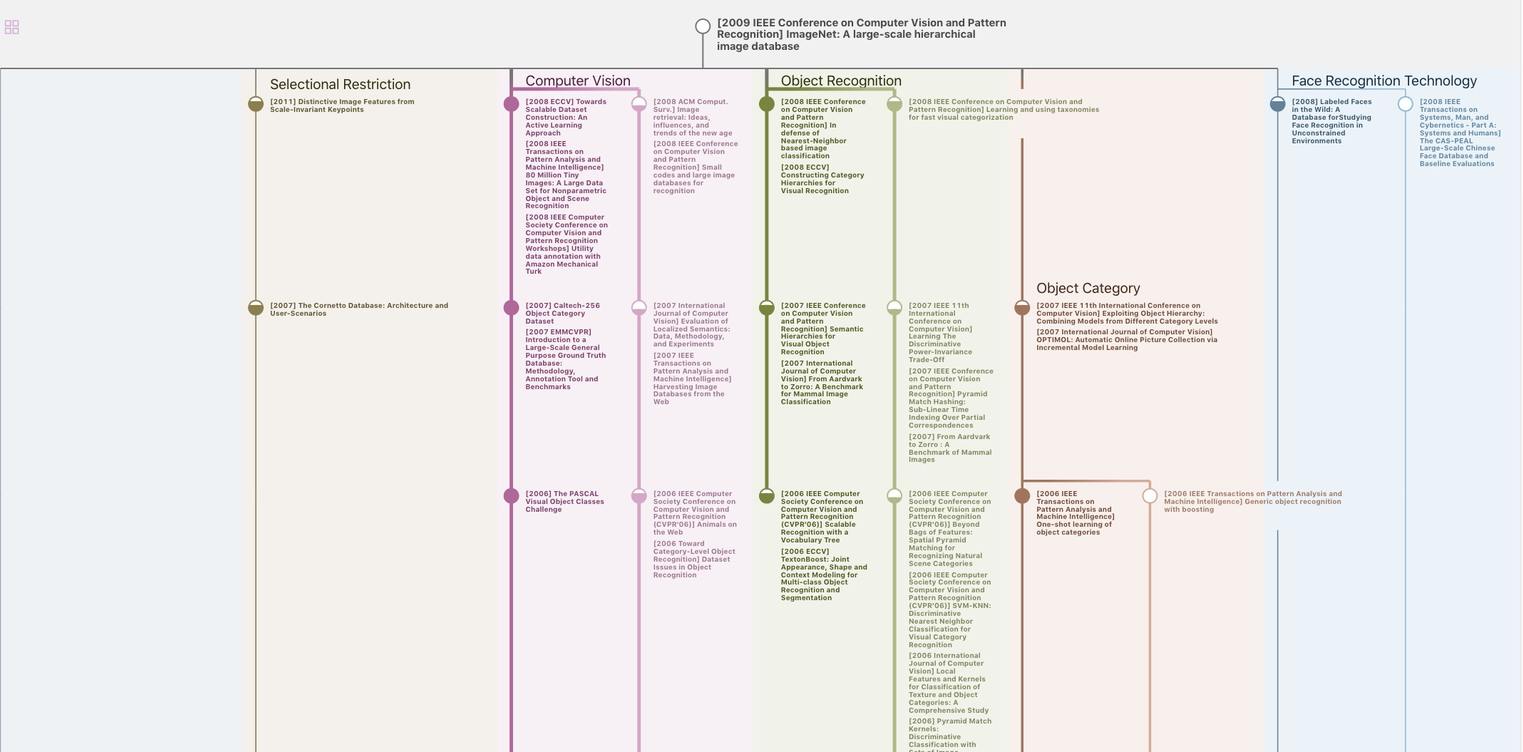
生成溯源树,研究论文发展脉络
Chat Paper
正在生成论文摘要