Unsupervised Domain Adaptation for Nighttime Aerial Tracking
2022 IEEE/CVF Conference on Computer Vision and Pattern Recognition (CVPR)(2022)
摘要
Previous advances in object tracking mostly reported on favorable illumination circumstances while neglecting performance at nighttime, which significantly impeded the development of related aerial robot applications. This work instead develops a novel unsupervised domain adaptation framework for nighttime aerial tracking (named UDAT). Specifically, a unique object discovery approach is provided to generate training patches from raw nighttime tracking videos. To tackle the domain discrepancy, we employ a Transformer-based bridging layer post to the feature extractor to align image features from both domains. With a Transformer day/night feature discriminator, the day-time tracking model is adversarially trained to track at night. Moreover, we construct a pioneering benchmark namely NAT2021 for unsupervised domain adaptive night-time tracking, which comprises a test set of 180 manually annotated tracking sequences and a train set of over 276k unlabelled nighttime tracking frames. Exhaustive experiments demonstrate the robustness and domain adaptability of the proposed framework in nighttime aerial tracking. The code and benchmark are available at https://github.com/vision4robotics/UDAT.
更多查看译文
关键词
Motion and tracking,Datasets and evaluation,Robot vision,Transfer/low-shot/long-tail learning
AI 理解论文
溯源树
样例
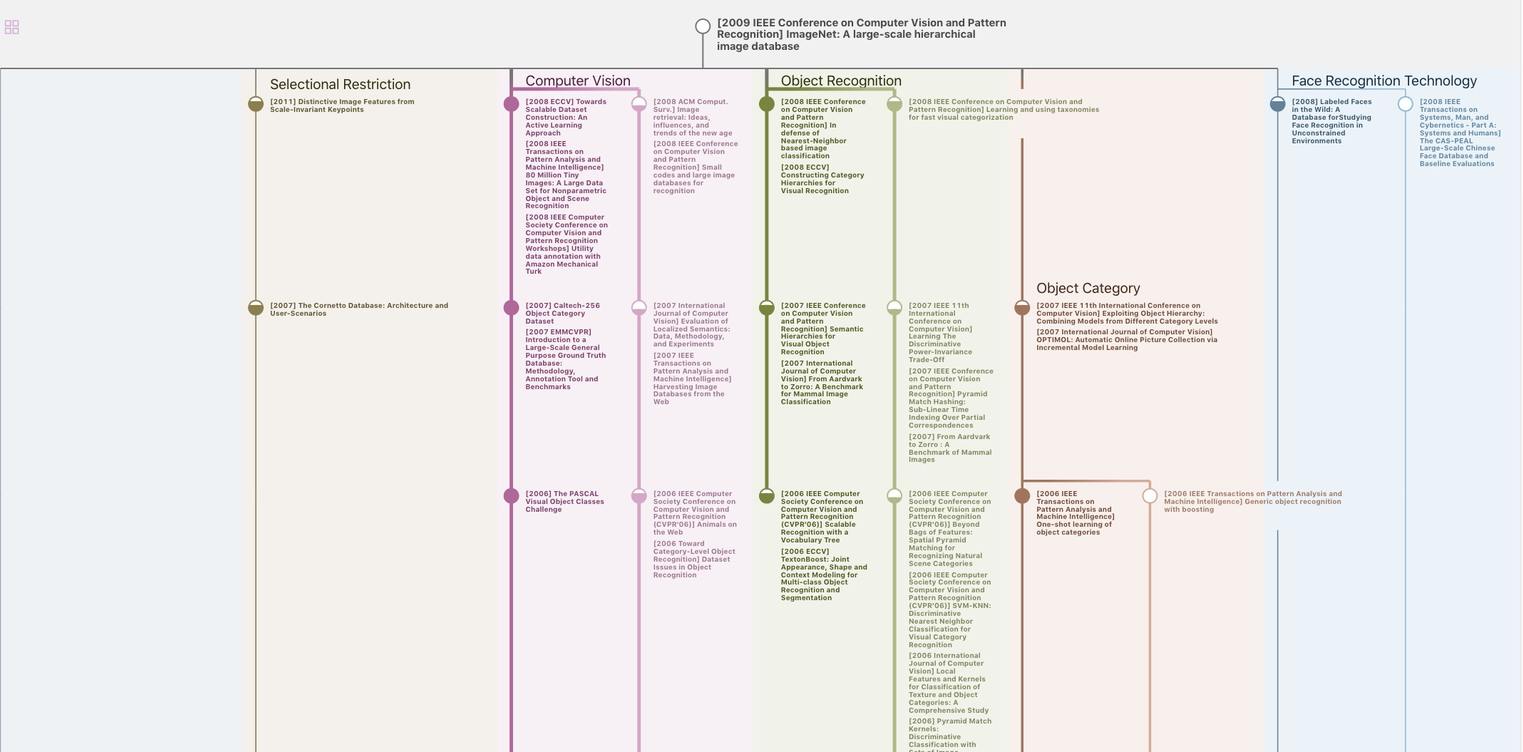
生成溯源树,研究论文发展脉络
Chat Paper
正在生成论文摘要