Towards Large-Scale Interpretable Knowledge Graph Reasoning for Dialogue Systems
FINDINGS OF THE ASSOCIATION FOR COMPUTATIONAL LINGUISTICS (ACL 2022)(2022)
摘要
Users interacting with voice assistants today need to phrase their requests in a very specific manner to elicit an appropriate response. This limits the user experience, and is partly due to the lack of reasoning capabilities of dialogue platforms and the hand-crafted rules that require extensive labor. One possible way to improve user experience and relieve the manual efforts of designers is to build an end-to-end dialogue system that can do reasoning itself while perceiving user's utterances. In this work, we propose a novel method to incorporate the knowledge reasoning capability into dialogue systems in a more scalable and generalizable manner. Our proposed method allows a single transformer model to directly walk on a large-scale knowledge graph to generate responses. To the best of our knowledge, this is the first work to have transformer models generate responses by reasoning over differentiable knowledge graphs. We investigate the reasoning abilities of the proposed method on both task-oriented and domain-specific chitchat dialogues. Empirical results show that this method can effectively and efficiently incorporate a knowledge graph into a dialogue system with fully-interpretable reasoning paths.
更多查看译文
关键词
dialogue
AI 理解论文
溯源树
样例
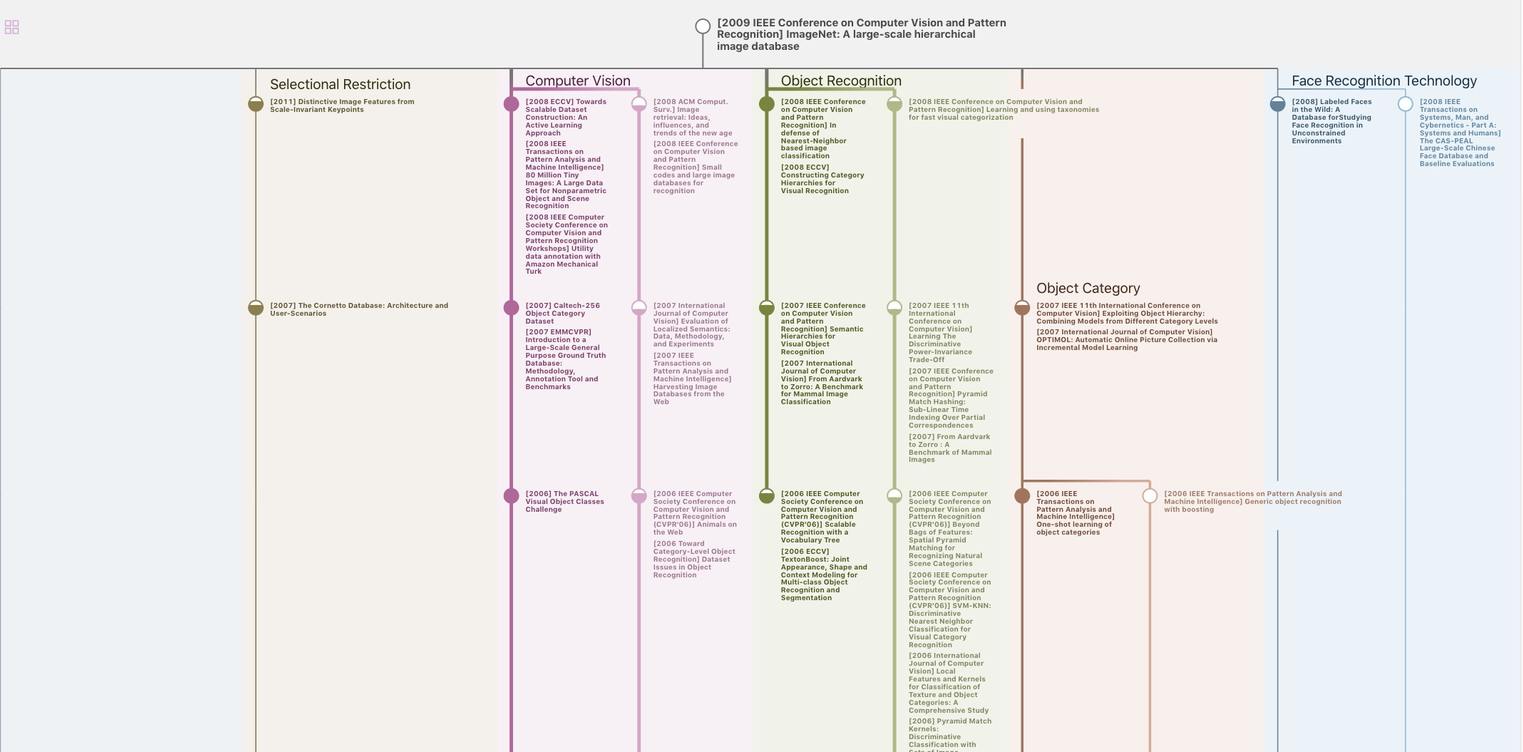
生成溯源树,研究论文发展脉络
Chat Paper
正在生成论文摘要