Hierarchical Reinforcement Learning of Locomotion Policies in Response to Approaching Objects: A Preliminary Study
arxiv(2022)
摘要
Animals such as rabbits and birds can instantly generate locomotion behavior in reaction to a dynamic, approaching object, such as a person or a rock, despite having possibly never seen the object before and having limited perception of the object's properties. Recently, deep reinforcement learning has enabled complex kinematic systems such as humanoid robots to successfully move from point A to point B. Inspired by the observation of the innate reactive behavior of animals in nature, we hope to extend this progress in robot locomotion to settings where external, dynamic objects are involved whose properties are partially observable to the robot. As a first step toward this goal, we build a simulation environment in MuJoCo where a legged robot must avoid getting hit by a ball moving toward it. We explore whether prior locomotion experiences that animals typically possess benefit the learning of a reactive control policy under a proposed hierarchical reinforcement learning framework. Preliminary results support the claim that the learning becomes more efficient using this hierarchical reinforcement learning method, even when partial observability (radius-based object visibility) is taken into account.
更多查看译文
关键词
hierarchical reinforcement learning,locomotion policies,approaching objects
AI 理解论文
溯源树
样例
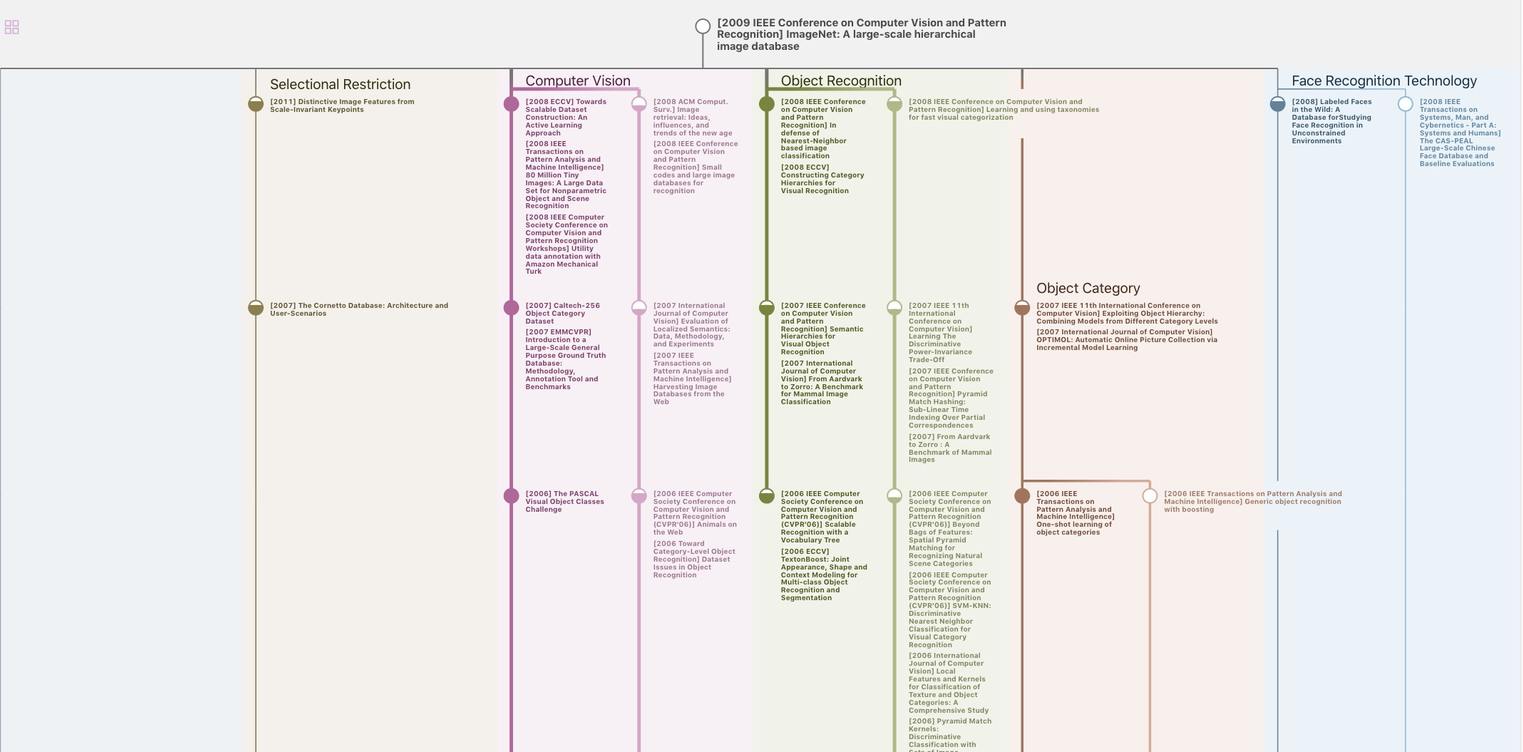
生成溯源树,研究论文发展脉络
Chat Paper
正在生成论文摘要