A Prompting-based Approach for Adversarial Example Generation and Robustness Enhancement.
CoRR(2022)
摘要
Recent years have seen the wide application of natural language processing (NLP) models in crucial areas such as finance, medical treatment, and news media, raising concerns about the model robustness and vulnerabilities. We find that prompt paradigm can probe special robust defects of pre-trained language models. Malicious prompt texts are first constructed for inputs and a pre-trained language model can generate adversarial examples for victim models via mask-filling. Experimental results show that prompt paradigm can efficiently generate more diverse adversarial examples besides synonym substitution. Then, we propose a novel robust training approach based on prompt paradigm which incorporates prompt texts as the alternatives to adversarial examples and enhances robustness under a lightweight minimax-style optimization framework. Experiments on three real-world tasks and two deep neural models show that our approach can significantly improve the robustness of models to resist adversarial attacks.
更多查看译文
AI 理解论文
溯源树
样例
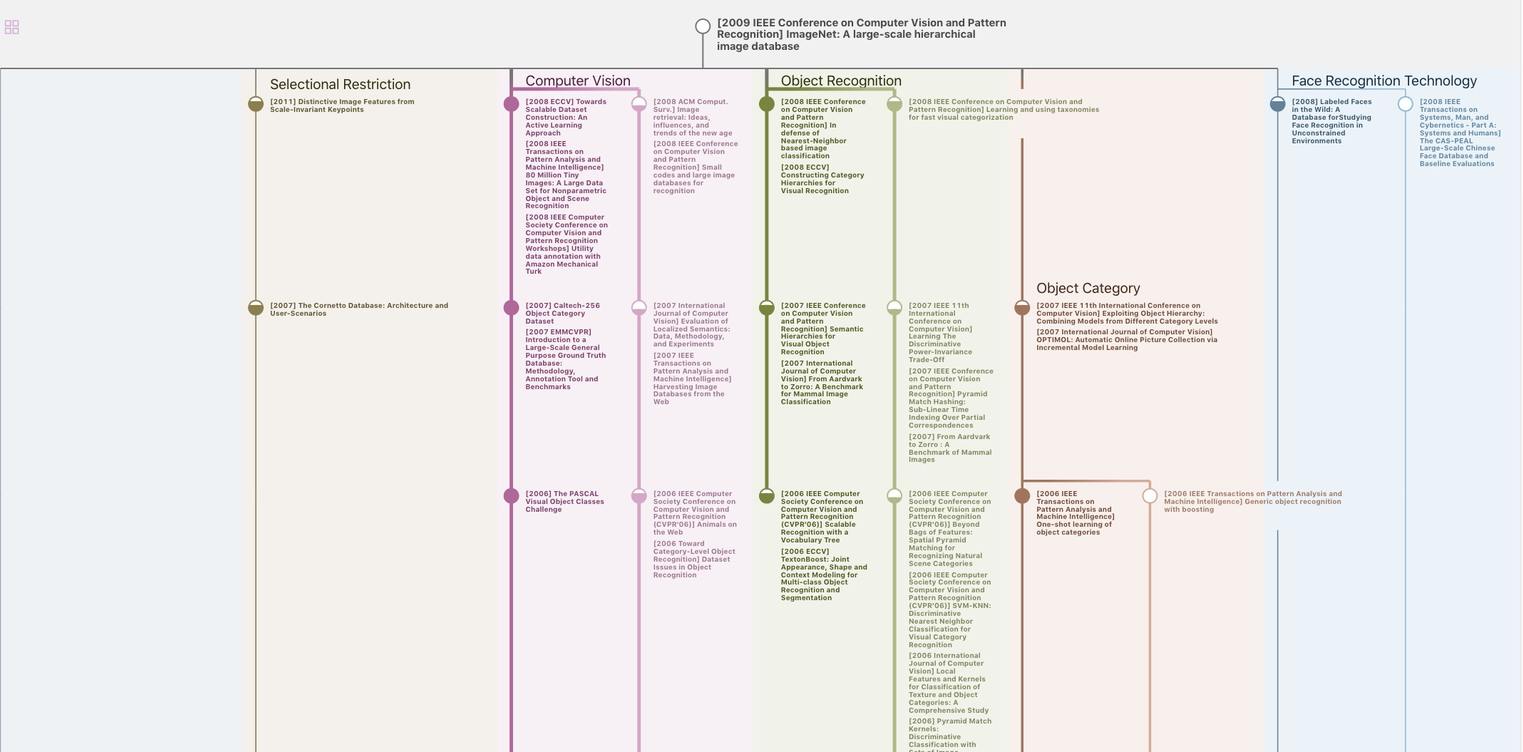
生成溯源树,研究论文发展脉络
Chat Paper
正在生成论文摘要