Performance-Robustness Tradeoffs in Adversarially Robust Linear-Quadratic Control
arxiv(2022)
摘要
While ${\mathcal{H}_\infty }$ methods can introduce robustness against worst-case perturbations, their nominal performance under conventional stochastic disturbances is often drastically reduced. Though this fundamental tradeoff between nominal performance and robustness is known to exist, it has not been quantitatively characterized. Toward addressing this issue, we borrow from the increasingly ubiquitous notion of adversarial training from machine learning to construct a class of controllers which are optimized for disturbances consisting of mixed stochastic and worst-case components. We find that this problem admits a stationary optimal controller that has a simple analytic form closely related to suboptimal ${\mathcal{H}_\infty }$ solutions. We then provide a quantitative performance-robustness tradeoff analysis, in which system-theoretic properties such as controllability and stability explicitly manifest in an interpretable manner. This provides practitioners with general guidance for determining how much robustness to incorporate based on a priori system knowledge. We empirically validate our results by comparing the performance of our controller against standard baselines, and plotting tradeoff curves.
更多查看译文
关键词
control,performance-robustness,linear-quadratic
AI 理解论文
溯源树
样例
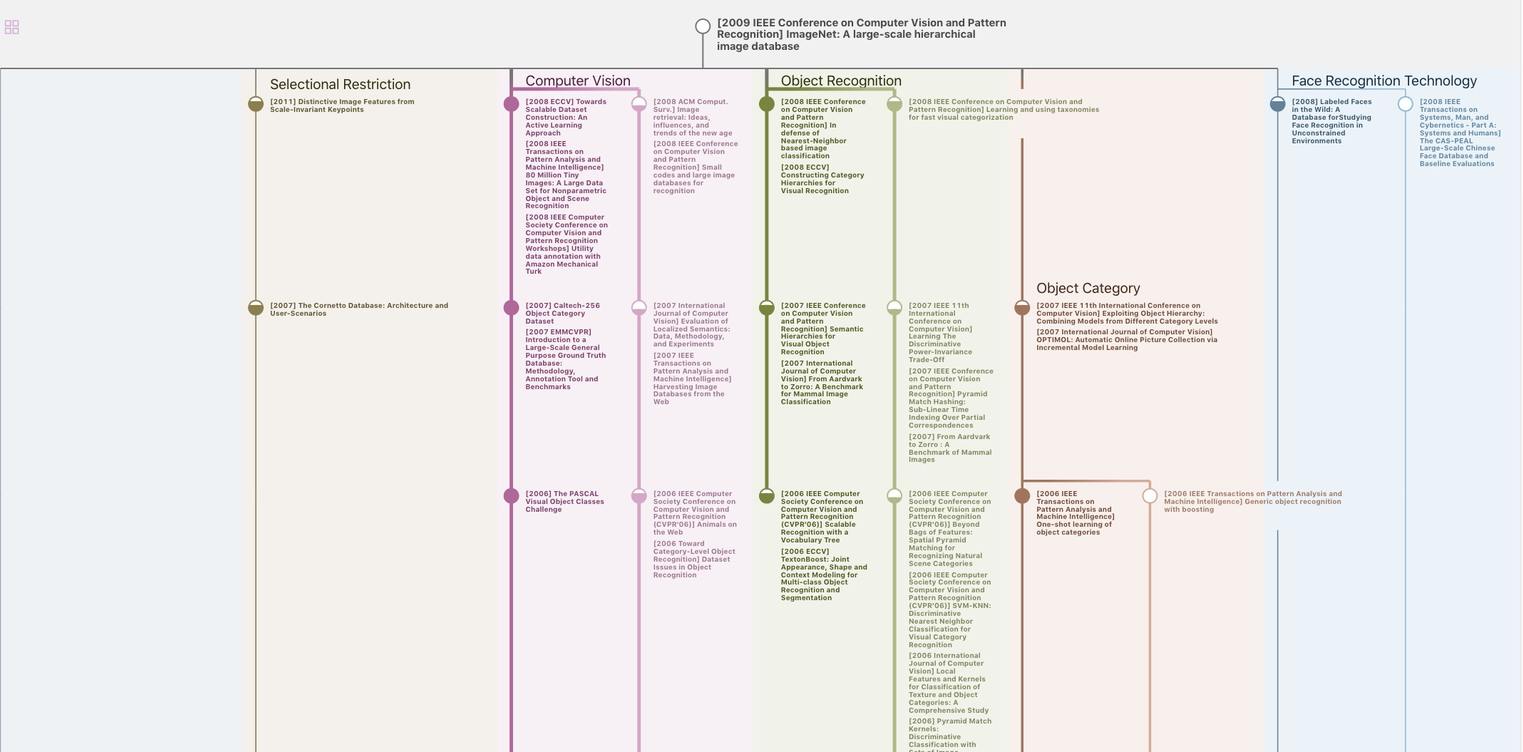
生成溯源树,研究论文发展脉络
Chat Paper
正在生成论文摘要