Longitudinal Self-Supervision for COVID-19 Pathology Quantification
arxiv(2022)
摘要
Quantifying COVID-19 infection over time is an important task to manage the hospitalization of patients during a global pandemic. Recently, deep learning-based approaches have been proposed to help radiologists automatically quantify COVID-19 pathologies on longitudinal CT scans. However, the learning process of deep learning methods demands extensive training data to learn the complex characteristics of infected regions over longitudinal scans. It is challenging to collect a large-scale dataset, especially for longitudinal training. In this study, we want to address this problem by proposing a new self-supervised learning method to effectively train longitudinal networks for the quantification of COVID-19 infections. For this purpose, longitudinal self-supervision schemes are explored on clinical longitudinal COVID-19 CT scans. Experimental results show that the proposed method is effective, helping the model better exploit the semantics of longitudinal data and improve two COVID-19 quantification tasks.
更多查看译文
关键词
pathology,self-supervision
AI 理解论文
溯源树
样例
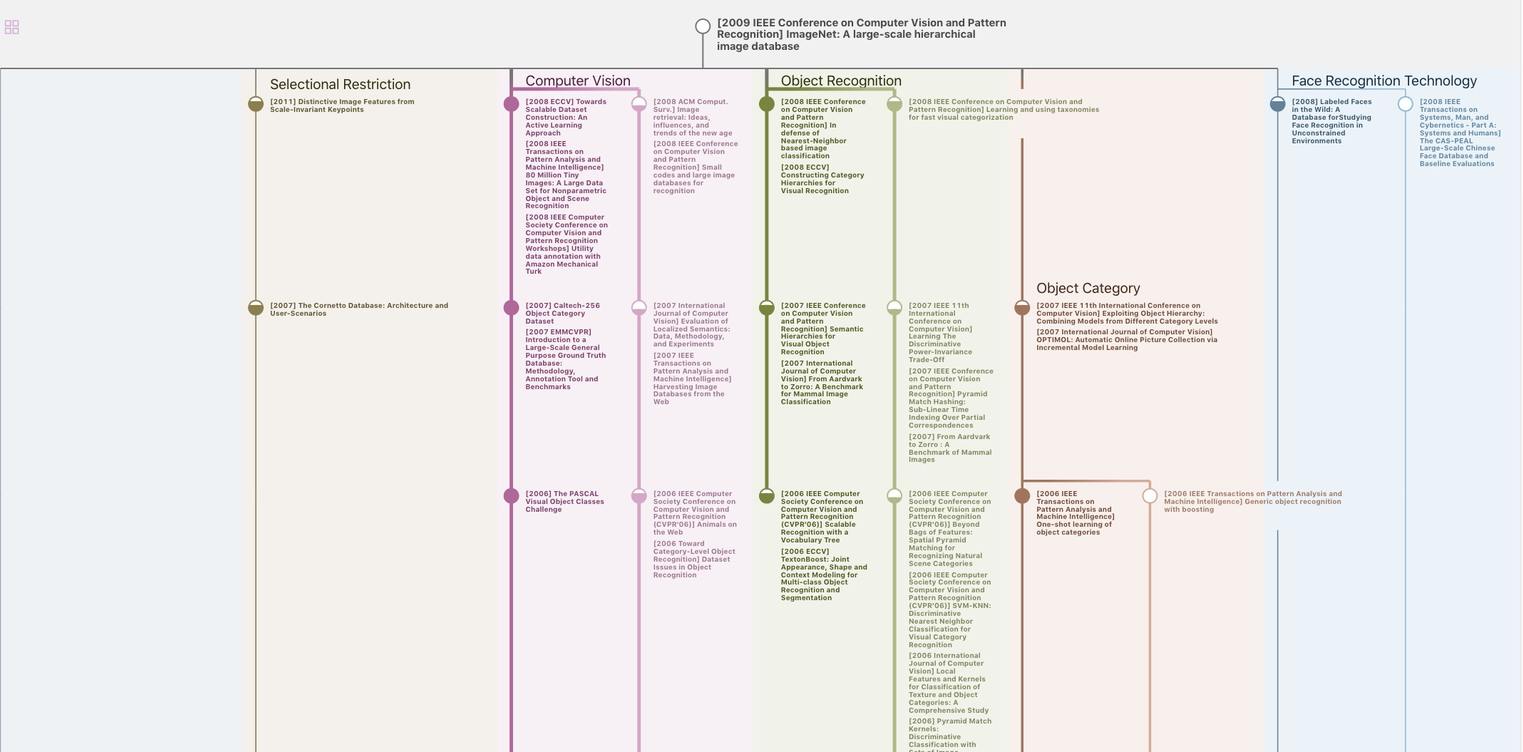
生成溯源树,研究论文发展脉络
Chat Paper
正在生成论文摘要