GAM(L)A: An econometric model for interpretable Machine Learning
arxiv(2022)
摘要
Despite their high predictive performance, random forest and gradient boosting are often considered as black boxes or uninterpretable models which has raised concerns from practitioners and regulators. As an alternative, we propose in this paper to use partial linear models that are inherently interpretable. Specifically, this article introduces GAM-lasso (GAMLA) and GAM-autometrics (GAMA), denoted as GAM(L)A in short. GAM(L)A combines parametric and non-parametric functions to accurately capture linearities and non-linearities prevailing between dependent and explanatory variables, and a variable selection procedure to control for overfitting issues. Estimation relies on a two-step procedure building upon the double residual method. We illustrate the predictive performance and interpretability of GAM(L)A on a regression and a classification problem. The results show that GAM(L)A outperforms parametric models augmented by quadratic, cubic and interaction effects. Moreover, the results also suggest that the performance of GAM(L)A is not significantly different from that of random forest and gradient boosting.
更多查看译文
关键词
interpretable machine learning,machine learning,econometric model
AI 理解论文
溯源树
样例
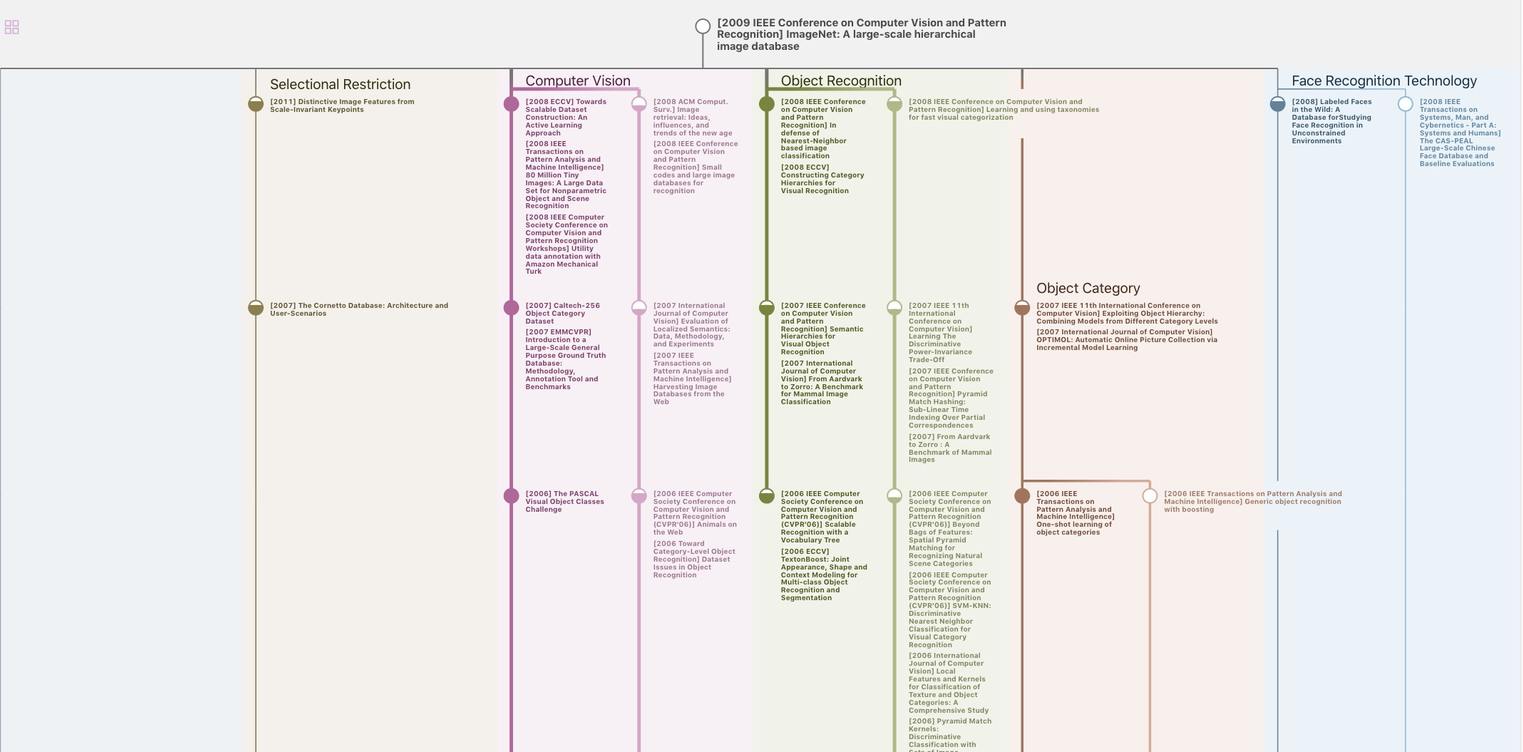
生成溯源树,研究论文发展脉络
Chat Paper
正在生成论文摘要