Identifying Predictors For All-Cause Mortality In Diabetic Patients In The Accord Trial Using Random Survival Forests
Circulation(2017)
摘要
Background: The ACCORD trial (NCT00000620) was terminated early due to an unexpected increase in mortality with intensive vs. standard glucose-lowering treatment (targeted HbA1c u003c6% vs. 7.0-7.9%) in type 2 diabetes (T2D) patients. We conducted exploratory analyses to identify factors predictive of mortality, using random survival forests (RSF), a machine-learning methodology. Methods: A total of 240 variables including, demographic, clinical and laboratory data, and their change from baseline during follow-up, were analyzed as potential predictors of mortality, using RSF in patients randomized to the intensive arm and standard arm. An RSF of 500 trees were constructed, with each tree constructed using a different bootstrap sample from the original data. The top 20 predictors, identified using the RSF method, were included in a multivariate analysis using a traditional Cox proportional hazards model with stepwise selection to validate the results. Results: During a 4.9 year median follow-up, 716/10251 pati...
更多查看译文
关键词
Mortality, Risk factors, Diabetes (Type II), Biomarkers, Outcomes
AI 理解论文
溯源树
样例
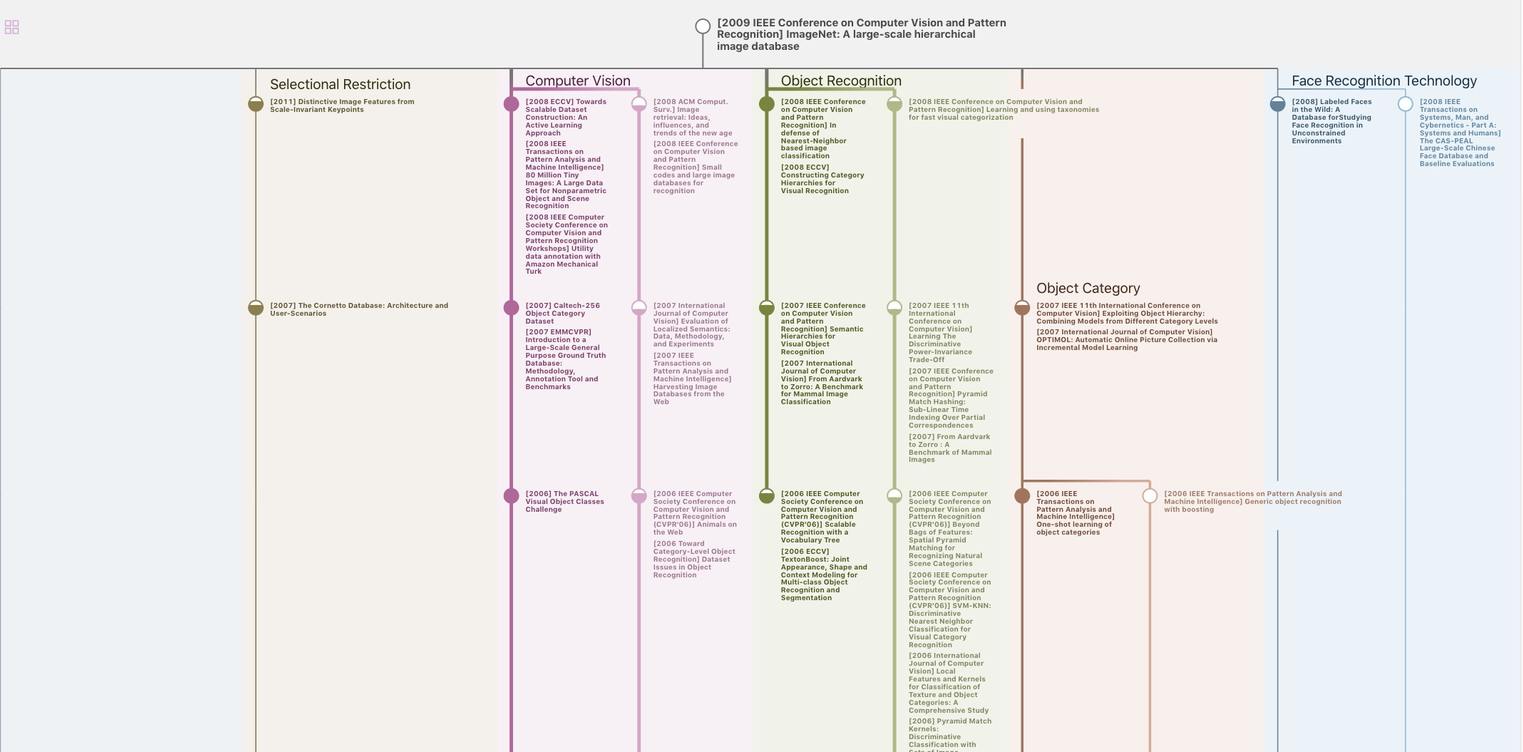
生成溯源树,研究论文发展脉络
Chat Paper
正在生成论文摘要