MSR-RCNN: A Multi-Class Crop Pest Detection Network Based on a Multi-Scale Super-Resolution Feature Enhancement Module
FRONTIERS IN PLANT SCIENCE(2022)
摘要
Pest disaster severely reduces crop yield and recognizing them remains a challenging research topic. Existing methods have not fully considered the pest disaster characteristics including object distribution and position requirement, leading to unsatisfactory performance. To address this issue, we propose a robust pest detection network by two customized core designs: multi-scale super-resolution (MSR) feature enhancement module and Soft-IoU (SI) mechanism. The MSR (a plug-and-play module) is employed to improve the detection performance of small-size, multi-scale, and high-similarity pests. It enhances the feature expression ability by using a super-resolution component, a feature fusion mechanism, and a feature weighting mechanism. The SI aims to emphasize the position-based detection requirement by distinguishing the performance of different predictions with the same Intersection over Union (IoU). In addition, to prosper the development of agricultural pest detection, we contribute a large-scale light-trap pest dataset (named LLPD-26), which contains 26-class pests and 18,585 images with high-quality pest detection and classification annotations. Extensive experimental results over multi-class pests demonstrate that our proposed method achieves the best performance by 67.4% of mAP on the LLPD-26 while being 15.0 and 2.7% gain than state-of-the-art pest detection AF-RCNN and HGLA respectively. Ablation studies verify the effectiveness of the proposed components.
更多查看译文
关键词
agricultural pest detection, convolutional neural network, feature enhancement, Soft-IoU, wisdom agriculture
AI 理解论文
溯源树
样例
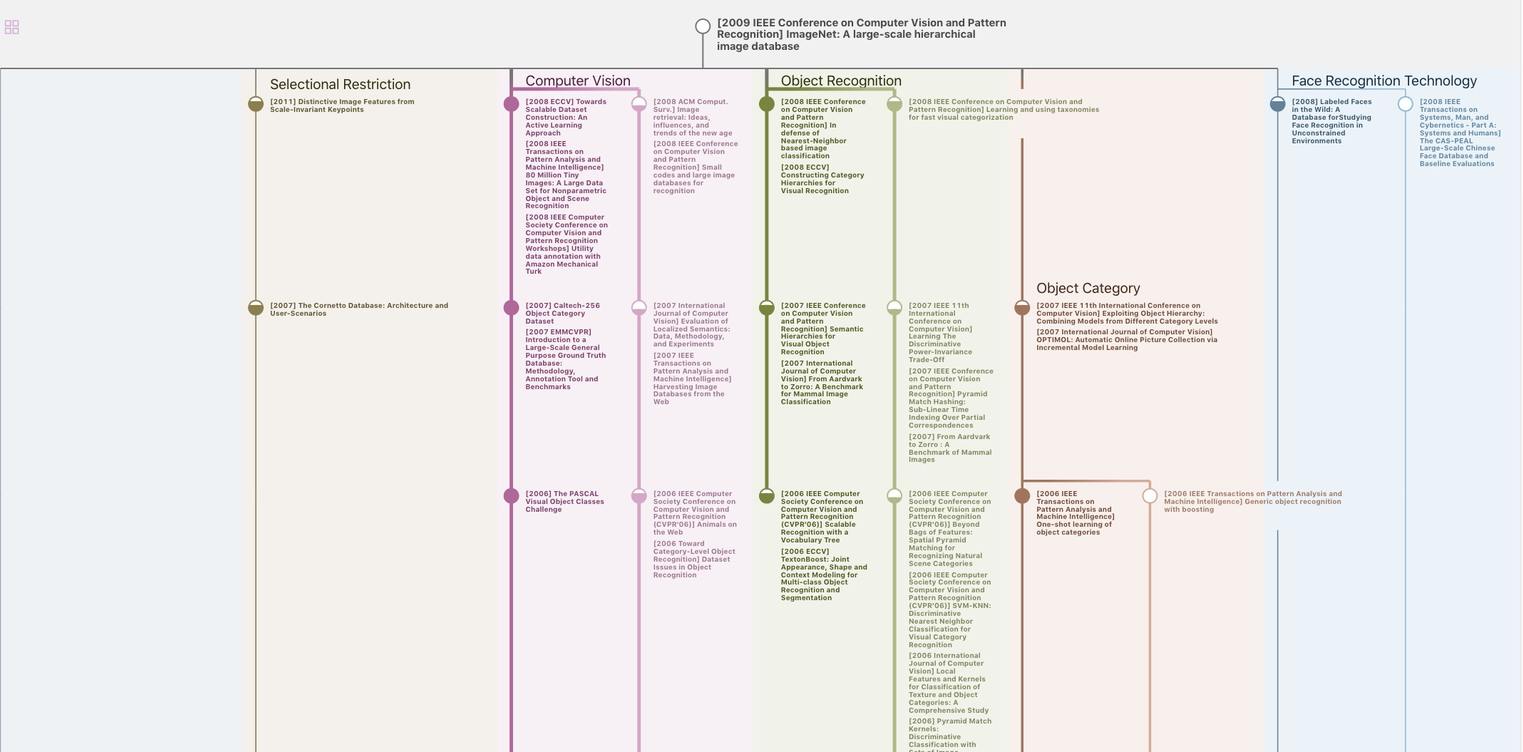
生成溯源树,研究论文发展脉络
Chat Paper
正在生成论文摘要