Implicit data crimes: Machine learning bias arising from misuse of public data
PROCEEDINGS OF THE NATIONAL ACADEMY OF SCIENCES OF THE UNITED STATES OF AMERICA(2022)
摘要
Although open databases are an important resource in the current deep learning (DL) era, they are sometimes used "off label": Data published for one task are used to train algorithms for a different one. This work aims to highlight that this common practice may lead to biased, overly optimistic results. We demonstrate this phenomenon for inverse problem solvers and show how their biased performance stems from hidden data-processing pipelines. We describe two processing pipelines typical of open-access databases and study their effects on three well-established algorithms developed for MRI reconstruction: compressed sensing, dictionary learning, and DL. Our results demonstrate that all these algorithms yield systematically biased results when they are naively trained on seemingly appropriate data: The normalized rms error improves consistently with the extent of data processing, showing an artificial improvement of 25 to 48% in some cases. Because this phenomenon is not widely known, biased results sometimes are published as state of the art; we refer to that as implicit "data crimes." This work hence aims to raise awareness regarding naive off-label usage of big data and reveal the vulnerability of modern inverse problem solvers to the resulting bias.
更多查看译文
关键词
data crimes, inverse problem, big data, MRI, bias
AI 理解论文
溯源树
样例
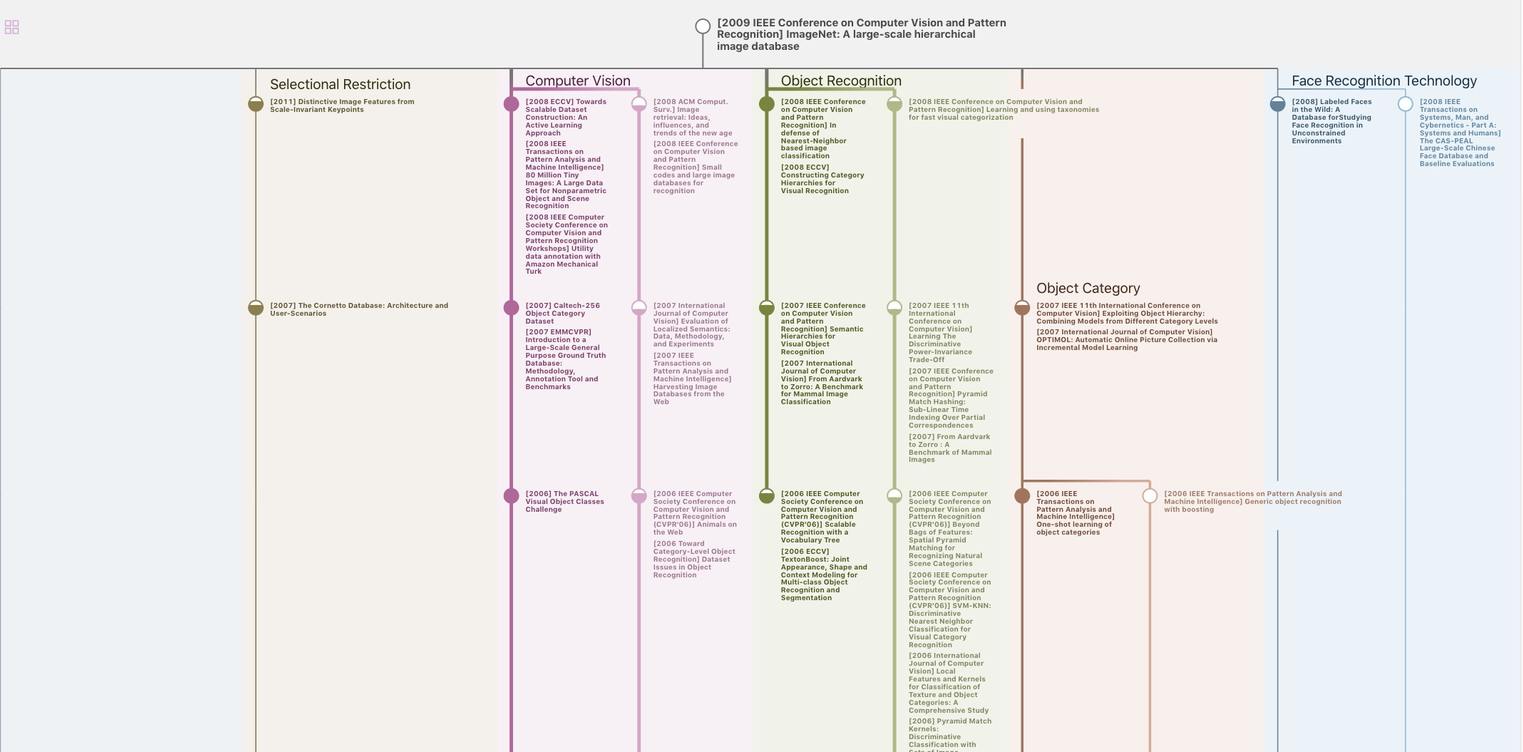
生成溯源树,研究论文发展脉络
Chat Paper
正在生成论文摘要