Quantifying Differences Between Affine and Nonlinear Spatial Normalization of FP-CIT Spect Images
INTERNATIONAL JOURNAL OF NEURAL SYSTEMS(2022)
摘要
Spatial normalization helps us to compare quantitatively two or more input brain scans. Although using an affine normalization approach preserves the anatomical structures, the neuroimaging field is more common to find works that make use of nonlinear transformations. The main reason is that they facilitate a voxel-wise comparison, not only when studying functional images but also when comparing MRI scans given that they fit better to a reference template. However, the amount of bias introduced by the nonlinear transformations can potentially alter the final outcome of a diagnosis especially when studying functional scans for neurological disorders like Parkinson's Disease. In this context, we have tried to quantify the bias introduced by the affine and the nonlinear spatial registration of FP-CIT SPECT volumes of healthy control subjects and patients with PD. For that purpose, we calculated the deformation fields of each participant and applied these deformation fields to a 3D-grid. As the space between the edges of small cubes comprising the grid change, we can quantify which parts from the brain have been enlarged, compressed or just remain the same. When the nonlinear approach is applied, scans from PD patients show a region near their striatum very similar in shape to that of healthy subjects. This artificially increases the interclass separation between patients with PD and healthy subjects as the local intensity is decreased in the latter region, and leads machine learning systems to biased results due to the artificial information introduced by these deformations.
更多查看译文
关键词
Neuroimaging, spatial registration, FP-CIT SPECT, Parkinson's disease, statistical maps
AI 理解论文
溯源树
样例
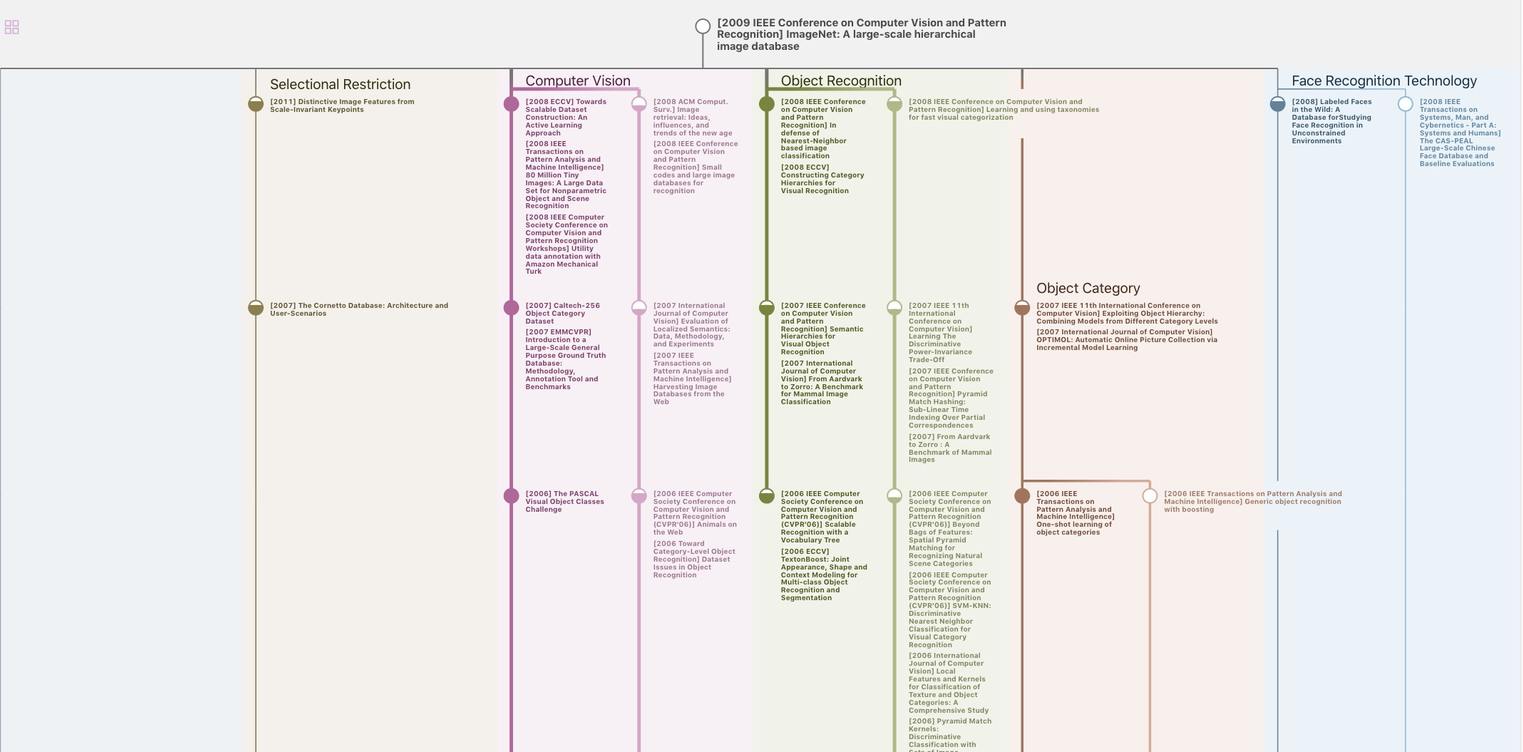
生成溯源树,研究论文发展脉络
Chat Paper
正在生成论文摘要