Machine Learning to Predict Executive Function in Adolescents with Repaired d-Transposition of the Great Arteries, Tetralogy of Fallot, and Fontan Palliation
The Journal of Pediatrics(2022)
摘要
Objective To identify predictors of impaired executive function in adolescents after surgical repair of critical congenital heart disease (CHD). Study design We analyzed patient factors, medical and surgical history, and family social class from 3 single-center studies of adolescents with d-transposition of the great arteries (d-TGA), tetralogy of Fallot (TOF), and Fontan repair. Machine learning models were developed using recursive partitioning to predict an executive function composite score based on five subtests (population mean 10, SD 3) of the Delis-Kaplan Executive Function System. Results The sample included 386 patients (139 d-TGA, 91 TOF, 156 Fontan) of age 15.1 +/- 2.1 (mean +/- SD) years and an executive function composite score of 8.6 +/- 2.4. Family social class emerged as the most important predictive factor. The lowest (worst) mean executive function score (5.3) occurred in patients with low to medium social class (Hollingshead index < 56) with one or more neurologic events and a diagnosis of TOF. The highest (best) mean score (9.7) occurred in subjects with high social class (Hollingshead index >= 56) and shorter duration of deep hypothermic circulatory arrest. Other factors predicting lower executive function scores included low birth weight and a greater number of catheterizations. Conclusions In regression tree modeling, family social class was the strongest predictor of executive function in adolescents with critical CHD, even in the presence of medical risk factors. Additional predictors included CHD diagnosis, birth weight, neurologic events, and number of procedures. These data highlight the importance of social class in mitigating risks of executive dysfunction in CHD.
更多查看译文
关键词
executive function,machine learning,congenital heart disease
AI 理解论文
溯源树
样例
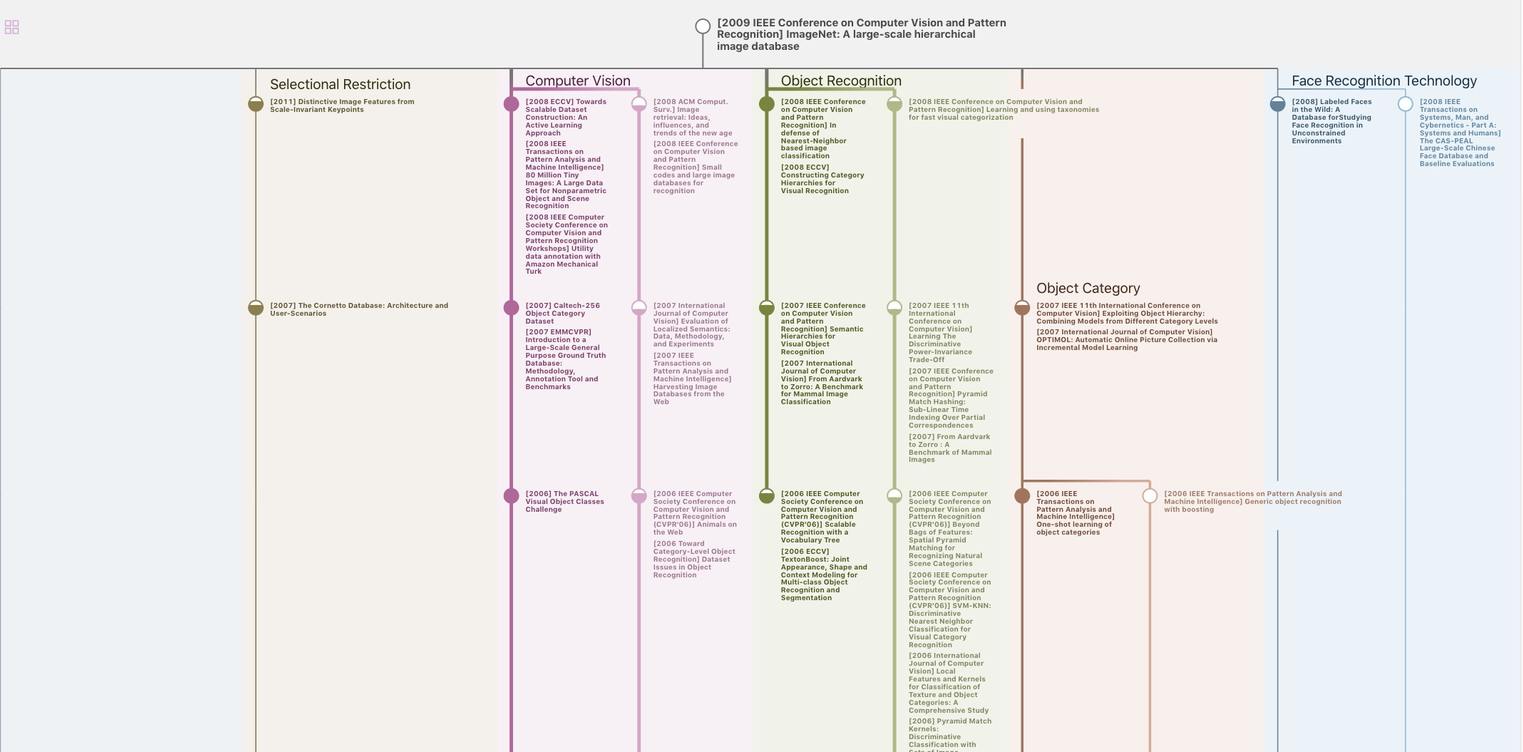
生成溯源树,研究论文发展脉络
Chat Paper
正在生成论文摘要