A Model-Based Reinforcement Learning Protocol for Routing in Vehicular Ad hoc Network
WIRELESS PERSONAL COMMUNICATIONS(2021)
摘要
Todays by equipping vehicles with wireless technologies, Vehicular Ad Hoc Network (VANET) has been emerged. This type of network can be utilized in many fields such as emergency, safety or entertainment. It is also considered as a main component of intelligent transportation system. However, due to the nodes velocity (vehicles velocity), varying density, obstacles and lack of fixed infrastructure, finding and maintaining a route between nodes are always challenging in VANET. Any routing protocol can be effective only if the nodes can learn and adapt themselves with such a dynamic environment. One way to achieve this adaptation is using machine learning techniques. In this paper we try to reach this goal by applying Multi-Agent Reinforcement Learning (MARL) that enables agents to solve routing optimization problems in a distributed way. Although model-free Reinforcement Learning (RL) schemes are introduced for this purpose, such techniques learn using a trial and error scheme in a real environment so they cannot reach an optimal policy in a short time. To deal with such a problem, we have proposed a mode-based RL based routing scheme. We have also developed a Fuzzy Logic (FL) system to evaluate the quality of links between neighbor nodes based on parameters such as velocity and connection quality. Outputs of this fuzzy system have been used to form the state transition model, needed in MARL. Results of evaluations have shown that our approach can improve some routing metrics like delivery ratio, end-to-end delay and traffic overhead.
更多查看译文
关键词
VANET, Routing, Reinforcement Learning, Fuzzy Logic
AI 理解论文
溯源树
样例
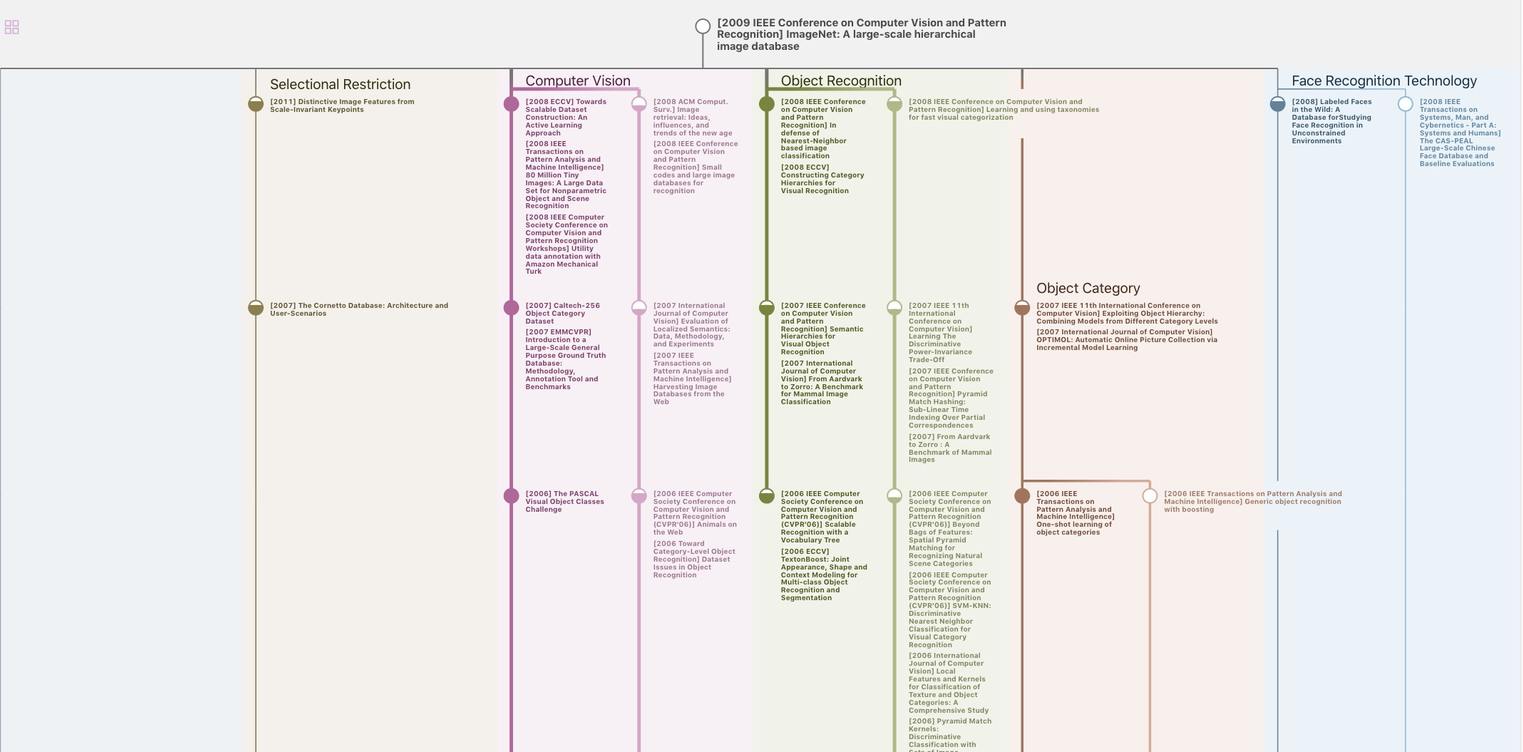
生成溯源树,研究论文发展脉络
Chat Paper
正在生成论文摘要