Incremental neighborhood entropy-based feature selection for mixed-type data under the variation of feature set
Applied Intelligence(2021)
摘要
Feature selection is to find relevant features and delete redundant features, which provides a basis for classification problems. In many real-world applications, mixed-type data including missing, numerical, and categorical features are ubiquitous in medical treatment, intrusion detection, traffic analysis and so on. Feature selection from mixed-type data has attracted considerable research attention. The neighborhood rough set model has attracted much attention to select a feature subset when handling with mixed-type data. In this study, we focus on the feature selection process for mixed-type data under the variation of feature set by the utilization of neighborhood rough sets. At first, the hybrid relation is given to define the similarity between objects for the mixed-type data without resorting to the discretization process. On this basis, the neighborhood entropy is given to evaluate the uncertainty of the mixed-type data. When new features may appear while old features are deleted, the updated neighborhood entropy is computed incrementally to reflect the significance of mixed-type features, which is an important step in the dynamic feature selection process. Finally, an efficient incremental feature selection algorithm for selecting a new feature subset is developed when deleting and adding a feature set simultaneously. Experimental results over different real-life data sets have verified the feasibility and efficiency of the proposed algorithm from the perspective of the runtime.
更多查看译文
关键词
Feature selection, Incremental algorithm, Dynamic mixed-type data, Neighborhood rough sets
AI 理解论文
溯源树
样例
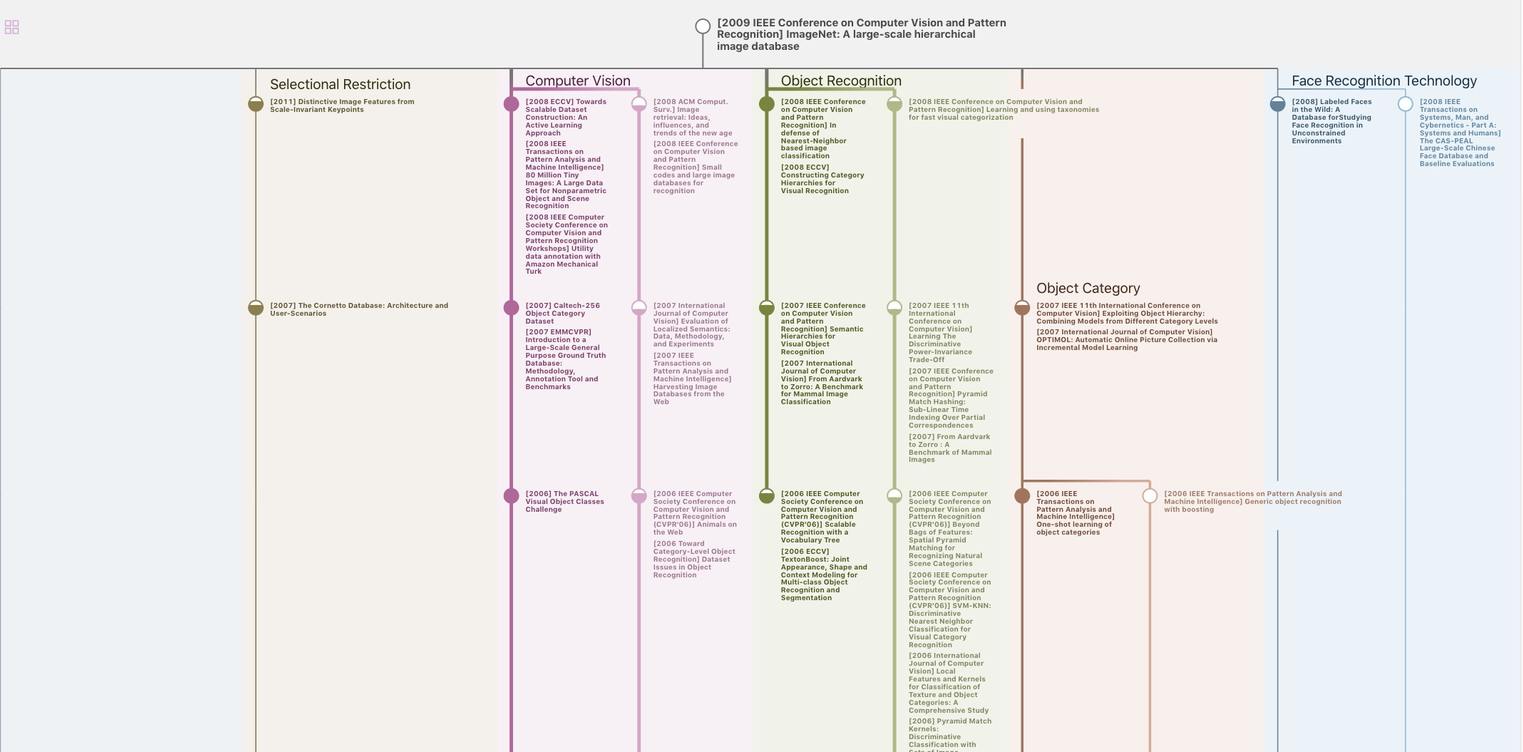
生成溯源树,研究论文发展脉络
Chat Paper
正在生成论文摘要