Breast Cancer Histopathological Image Classification Using Attention High‐order Deep Network
INTERNATIONAL JOURNAL OF IMAGING SYSTEMS AND TECHNOLOGY(2022)
摘要
Computer‐aided classification of pathological images is of the great significance for breast cancer diagnosis. In recent years, deep learning methods for breast cancer pathological image classification have made breakthrough progress, becoming the mainstream in this field. To capture more discriminant deep features for breast cancer pathological images, this work introduces a novel attention high‐order deep network (AHoNet) by simultaneously embedding attention mechanism and high‐order statistical representation into a residual convolutional network. AHoNet firstly employs an efficient channel attention module with non‐dimensionality reduction and local cross‐channel interaction to achieve local salient deep features of breast cancer pathological images. Then, their second‐order covariance statistics are further estimated through matrix power normalization, which provides a more robust global feature presentation of breast cancer pathological images. We extensively evaluate AHoNet on the public BreakHis and BACH breast cancer pathology datasets. Experimental results illustrate that AHoNet gains the optimal patient‐level classification accuracies of 99.29% and 85% on the BreakHis and BACH database, respectively, demonstrating the competitive performance with state‐of‐the‐art single models on this medical image application.
更多查看译文
关键词
breast cancer histopathological image classification,convolutional neural network,covariance pooling,efficient channel attention,second-order statistics
AI 理解论文
溯源树
样例
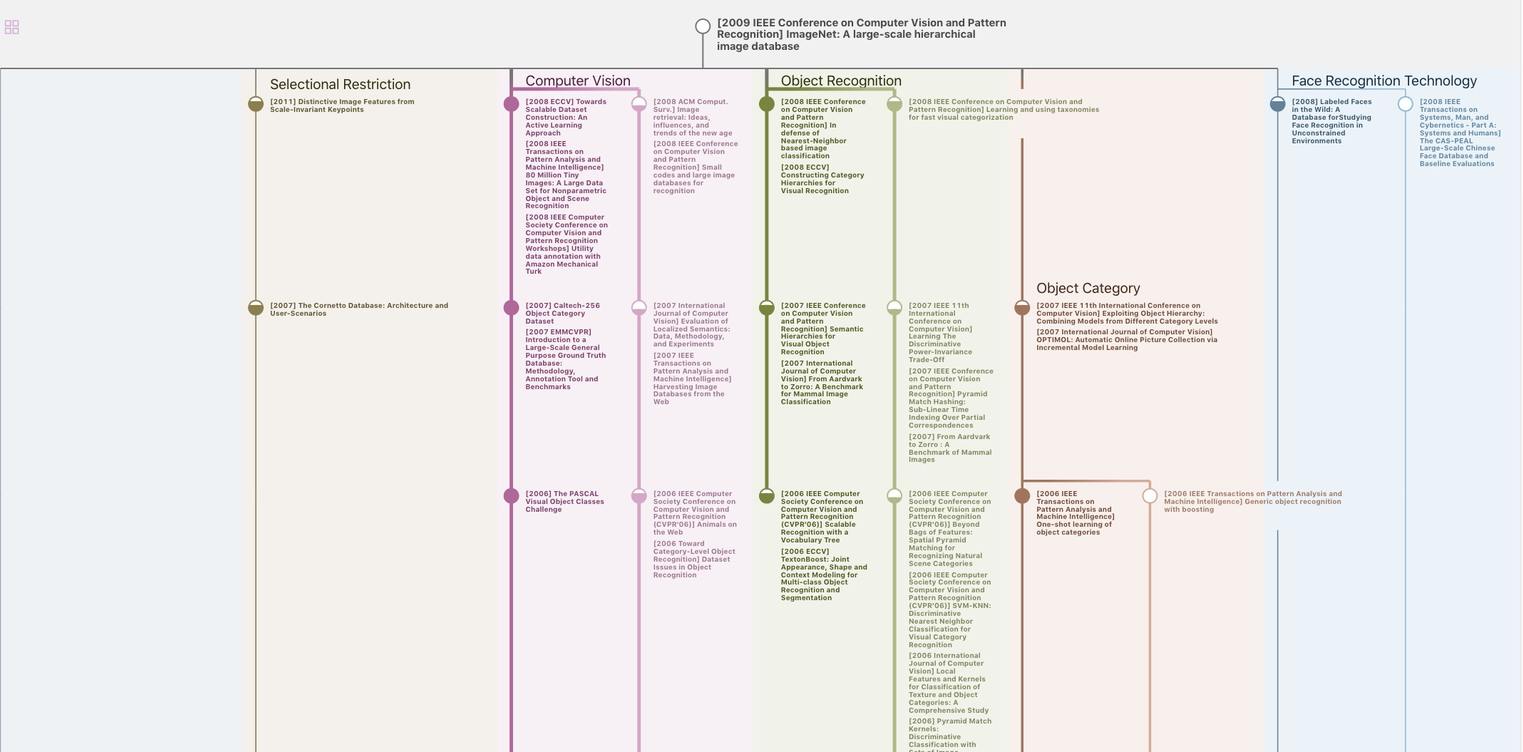
生成溯源树,研究论文发展脉络
Chat Paper
正在生成论文摘要