Evaluating the Impact of Integrating Similar Translations into Neural Machine Translation.
Inf.(2022)
摘要
Previous research has shown that simple methods of augmenting machine translation training data and input sentences with translations of similar sentences (or fuzzy matches), retrieved from a translation memory or bilingual corpus, lead to considerable improvements in translation quality, as assessed by a limited set of automatic evaluation metrics. In this study, we extend this evaluation by calculating a wider range of automated quality metrics that tap into different aspects of translation quality and by performing manual MT error analysis. Moreover, we investigate in more detail how fuzzy matches influence translations and where potential quality improvements could still be made by carrying out a series of quantitative analyses that focus on different characteristics of the retrieved fuzzy matches. The automated evaluation shows that the quality of NFR translations is higher than the NMT baseline in terms of all metrics. However, the manual error analysis did not reveal a difference between the two systems in terms of total number of translation errors; yet, different profiles emerged when considering the types of errors made. Finally, in our analysis of how fuzzy matches influence NFR translations, we identified a number of features that could be used to improve the selection of fuzzy matches for NFR data augmentation.
更多查看译文
关键词
neural machine translation,translation memory,evaluation
AI 理解论文
溯源树
样例
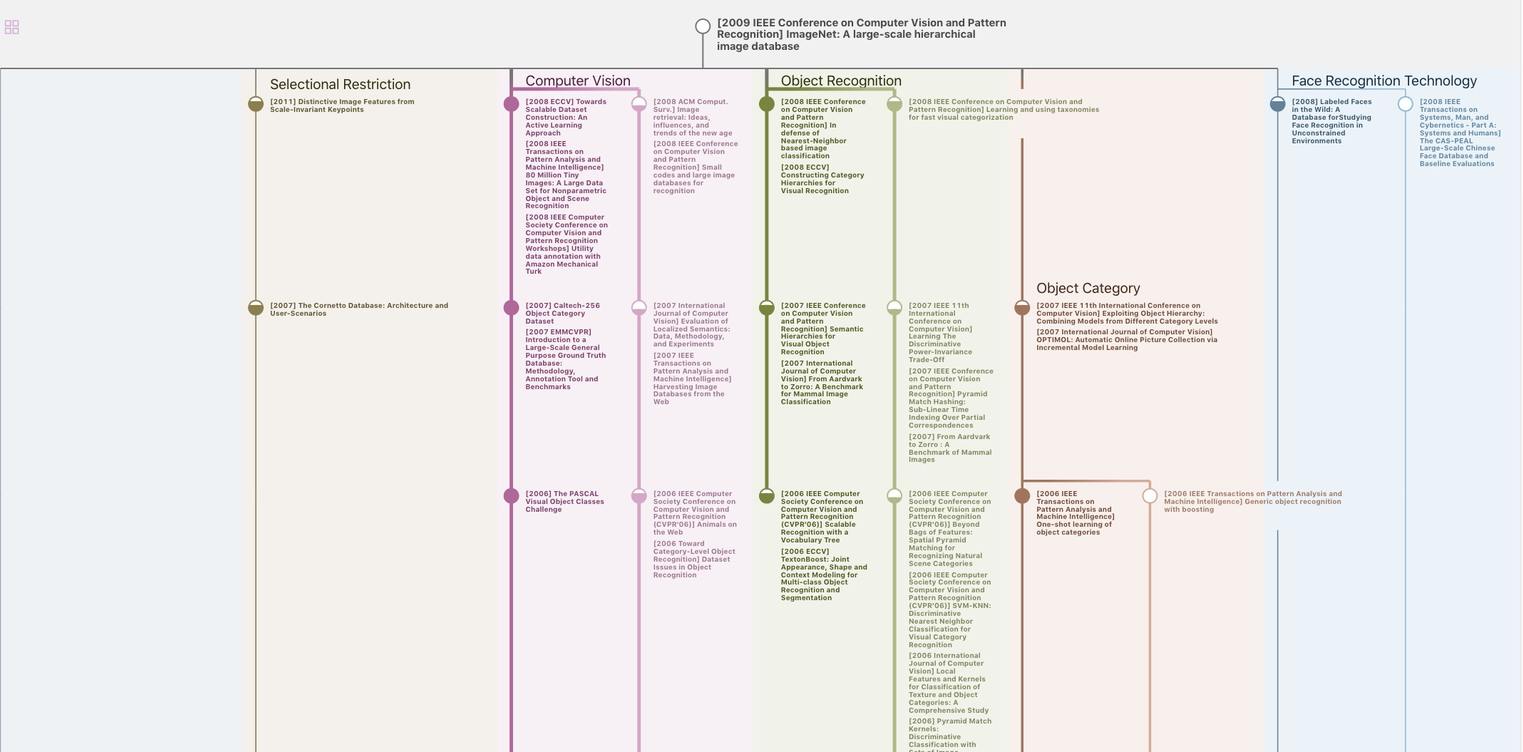
生成溯源树,研究论文发展脉络
Chat Paper
正在生成论文摘要