Light-weight shadow detection via GCN-based annotation strategy and knowledge distillation
Computer vision and image understanding(2022)
摘要
This paper discusses shadow detection problem, and proposes a light-weight network to achieve both accurate detection results and high computation efficiency. Firstly, we begin by presenting a compact network for realtime shadow detection. Secondly, to improve the performance of our light-weight networks, we propose two complementary and necessary strategies, i.e., the use of extra training data and knowledge distillation. Note that collecting a large amount of extra data will lead to the following challenge: shadow scenes is various, while annotating for those complex scenarios is time-consuming and expensive, sometimes even need expert help. To solve it, in the first step, we introduce a novel shadow annotation strategy based on graph convolutional networks, namely Anno-GCN, to provide extra training pairs, which obtains a complete shadow mask via only several annotation scribbles. In the second step, we can combine knowledge distillation with these sufficient GCN-labeled training data to further improve the performance of the light-weight network. Extensive experiments demonstrate that our method can achieve a state-of-the-art inference accuracy, computational efficiency, and generalizability with only about 2.97 M parameters.
更多查看译文
关键词
Light-weight,Image processing,Graph convolutional network,Semi-supervised learning,Shadow detection
AI 理解论文
溯源树
样例
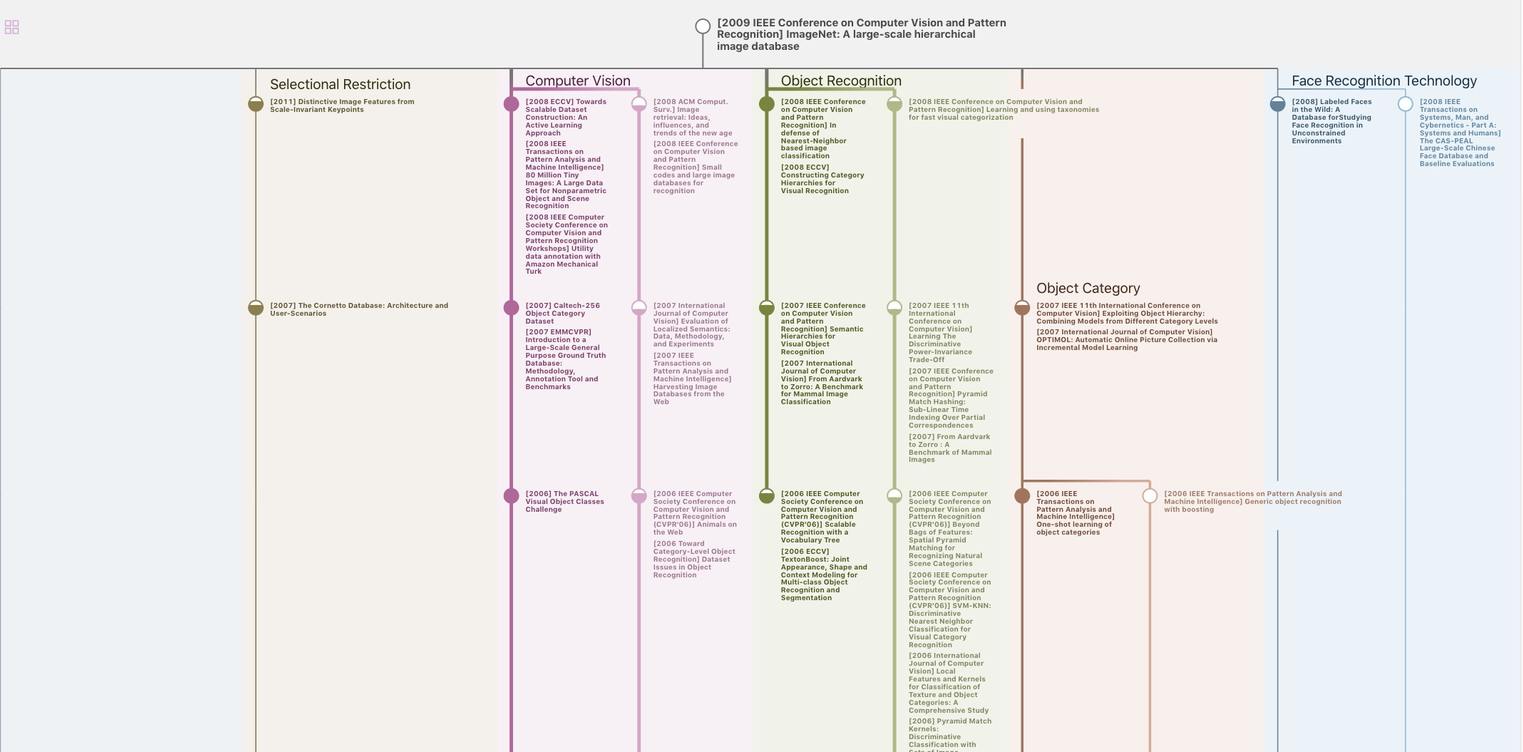
生成溯源树,研究论文发展脉络
Chat Paper
正在生成论文摘要