Data-driven Parameter Estimation Of Contaminated Damped Exponentials.
ACSCC(2021)
摘要
Damped exponentials appear naturally in a wide range of applications including structural health monitoring and electric machine fault detection. In this paper, given finite time-domain samples of composite, contaminated damped exponentials, we propose novel deep architectures to estimate the number of exponentials and recover the frequency and damping coefficient of each exponential. In our architecture, a damped exponential representation model maps time-domain samples to a frequency-damping spectrum representation, while a counting model then counts the number of exponentials. Combining the spectrum representation and the estimated number of exponentials, the frequencies and damping coefficients of the exponentials can be recovered automatically. Altogether, this yields an efficient learning-based method for parameter estimation of contaminated damped exponentials. Our experiments indicate that the proposed method is very effective and can robustly handle exponentials with close or even overlapping frequencies (resp. damping coefficients) as long as the damping coefficients (resp. frequencies) are sufficiently separated.
更多查看译文
关键词
Damped exponentials,deep learning,parameter estimation,signal decomposition
AI 理解论文
溯源树
样例
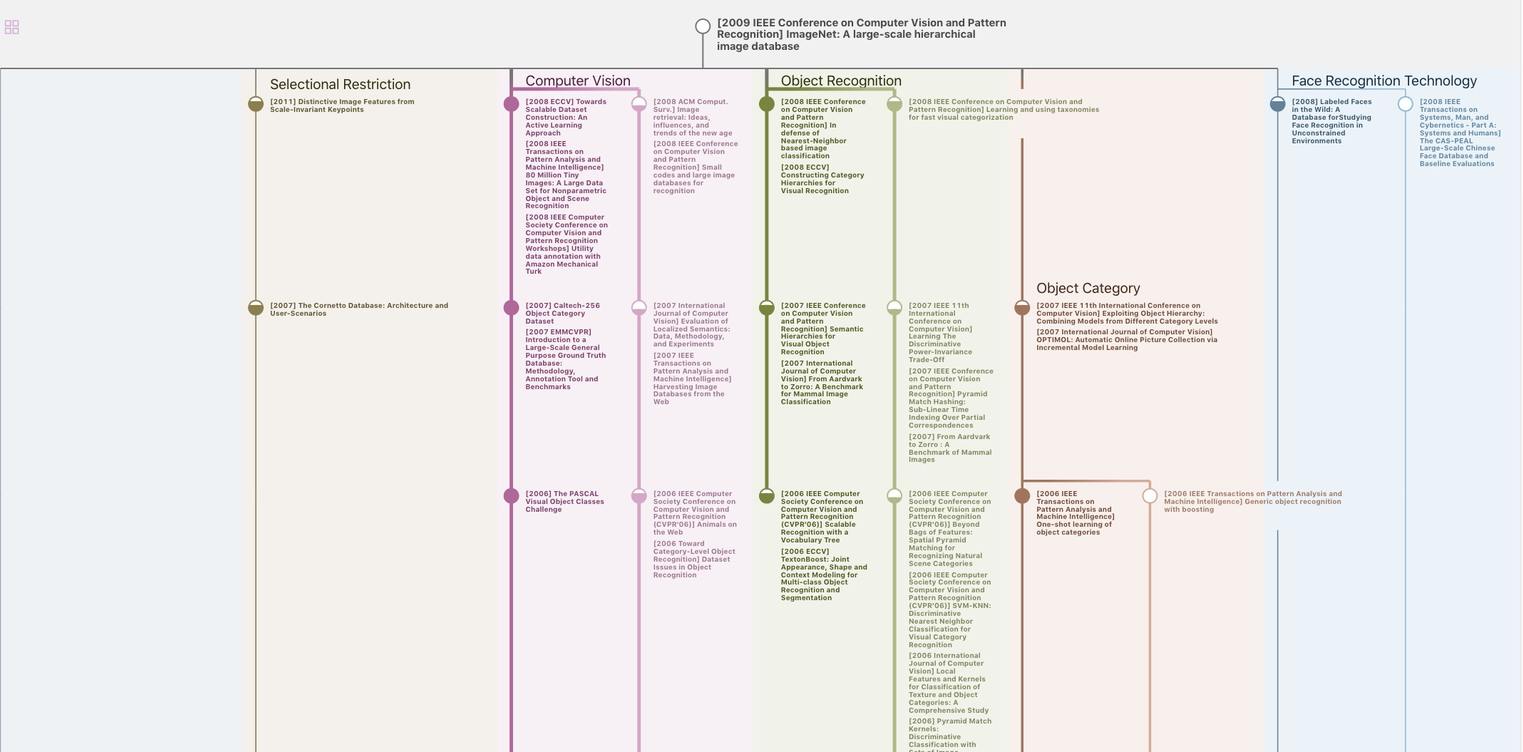
生成溯源树,研究论文发展脉络
Chat Paper
正在生成论文摘要