An End-to-End Dialect Identification System with Transfer Learning from a Multilingual Automatic Speech Recognition Model.
Interspeech(2021)
摘要
In this paper, we propose an end-to-end (E2E) dialect identification system trained using transfer learning from a multilingual automatic speech recognition (ASR) model. This is also an extension of our submitted system to the Oriental Language Recognition Challenge 2020 (AP20-OLR). We verified its applicability using the dialect identification (DID) task of the AP20-OLR. First, we trained a robust conformer-based joint connectionist temporal classification (CTC) /attention multilingual E2E ASR model using the training corpora of eight languages, independent of the target dialects. Second, we initialized the E2E-based classifier with the ASR model's shared encoder using a transfer learning approach. Finally, we trained the classifier on the target dialect corpus. We obtained the final classifier by selecting the best model from the following: (1) the averaged model in term of the loss values; and (2) the averaged model in term of classification accuracy. Our experiments on the DID test-set of the AP20-OLR demonstrated that significant identification improvements were achieved for three Chinese dialects. The performances of our system outperforms the winning team of the AP20-OLR, with the largest relative reductions of 19.5% in C-av(g) and 25.2% in EER.
更多查看译文
关键词
dialect identification,end-to-end network,multilingual ASR,transfer learning
AI 理解论文
溯源树
样例
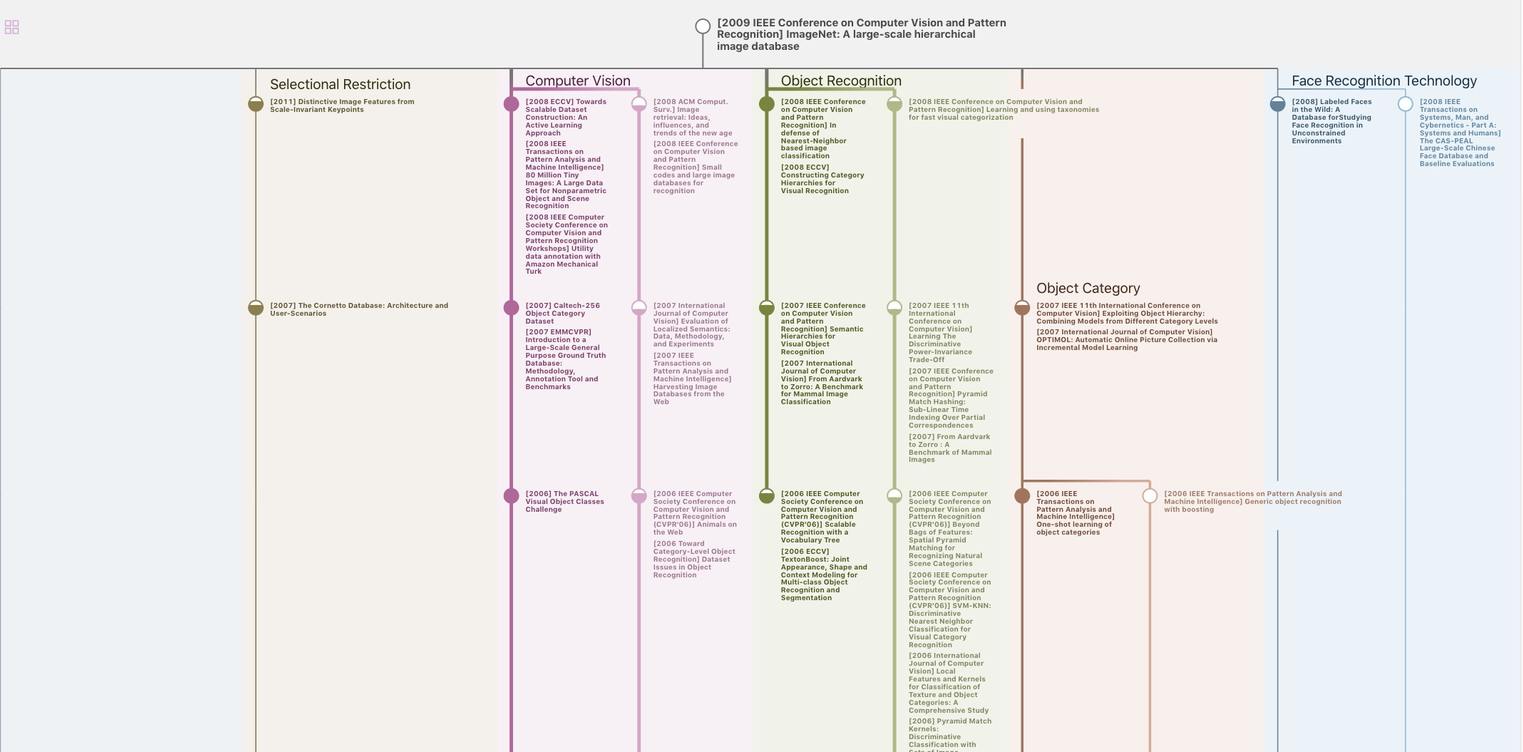
生成溯源树,研究论文发展脉络
Chat Paper
正在生成论文摘要