Weakly-Supervised Learning for Fine-Grained Emotion Recognition Using Physiological Signals
user-5e9d449e4c775e765d44d7c9(2023)
摘要
Instead of predicting just one emotion for one activity (e.g., video watching), fine-grained emotion recognition enables more temporally precise recognition. Previous works on fine-grained emotion recognition require segment-by-segment, fine-grained emotion labels to train the recognition algorithm. However, experiments to collect these labels are costly and time-consuming compared with only collecting one emotion label after the user watched that stimulus (i.e., the post-stimuli emotion labels). To recognize emotions at a finer granularity level when trained with only post-stimuli labels, we propose an emotion recognition algorithm based on Deep Multiple Instance Learning (EDMIL) using physiological signals. EDMIL recognizes fine-grained valence and arousal (V-A) labels by identifying which instances represent the post-stimuli V-A annotated by users after watching the videos. Instead of fully-supervised training, the instances are weakly-supervised by the post-stimuli labels in the training stage. The V-A of instances are estimated by the instance gains, which indicate the probability of instances to predict the post-stimuli labels. We tested EDMIL on three different datasets, CASE, MERCA and CEAP-360VR, collected in three different environments: desktop, mobile and HMD-based Virtual Reality, respectively. Recognition results validated with the fine-grained V-A self-reports show that for subject-independent 3-class classification (high/neutral/low), EDMIL obtains promising recognition accuracies: 75.63% and 79.73% for V-A on CASE, 70.51% and 67.62% for V-A on MERCA and 65.04% and 67.05% for V-A on CEAP-360VR. Our ablation study shows that all components of EDMIL contribute to both the classification and regression tasks. Our experiments also show that (1) compared with fully-supervised learning, weakly-supervised learning can reduce the problem of overfitting caused by the temporal mismatch between fine-grained annotations and physiological signals, (2) instance segment lengths between 1-2 s result in the highest recognition accuracies and (3) EDMIL performs best if post-stimuli annotations consist of less than 30% or more than 60% of the entire video watching.
更多查看译文
关键词
Deep multiple instance learning,emotion recognition,physiological signals,temporal ambiguity,weakly-supervised learning
AI 理解论文
溯源树
样例
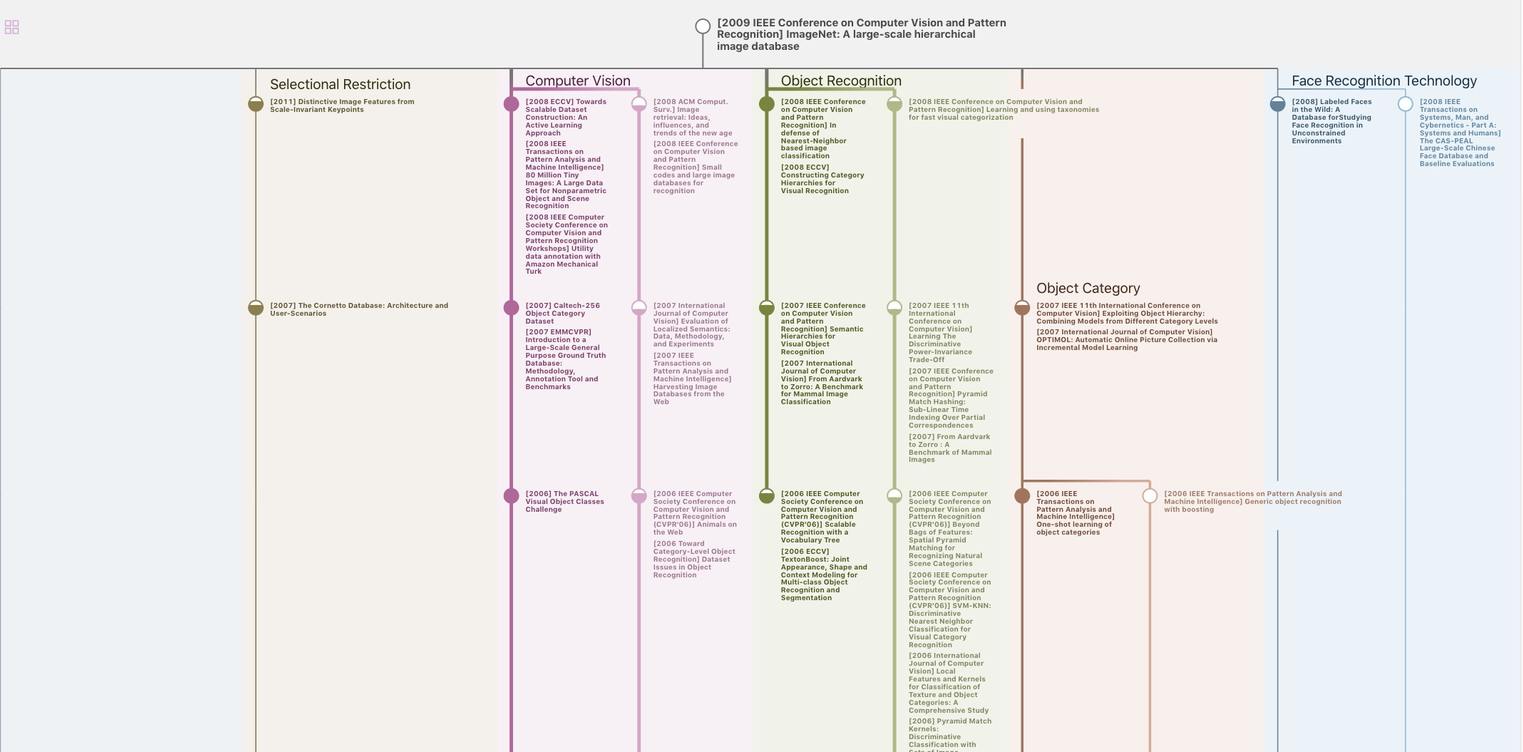
生成溯源树,研究论文发展脉络
Chat Paper
正在生成论文摘要