Machine Learning-Based Mortality Prediction of Patients at Risk During Hospital Admission
JOURNAL OF PATIENT SAFETY(2022)
摘要
Objectives The ability to predict in-hospital mortality from data available at hospital admission would identify patients at risk and thereby assist hospital-wide patient safety initiatives. Our aim was to use modern machine learning tools to predict in-hospital mortality from standardized data sets available at hospital admission. Methods This was a retrospective, observational study in 3 adult tertiary care hospitals in Western Australia between January 2008 and June 2017. Primary outcome measures were the area under the curve for the receiver operating characteristics curve, the F-1 score, and the average precision of the 4 machine learning algorithms used: logistic regression, neural networks, random forests, and gradient boosting trees. Results Using our 4 predictive models, in-hospital mortality could be predicted satisfactorily (areas under the curve for neural networks, logistic regression, random forests, and gradient boosting trees: 0.932, 0.936, 0.935, and 0.935, respectively), with moderate F-1 scores: 0.378, 0.367, 0.380, and 0.380, respectively. Average precision values were 0.312, 0.321, 0.334, and 0.323, respectively. It remains unknown whether additional features might improve our models; however, this would result in additional efforts for data acquisition in daily clinical practice. Conclusions This study demonstrates that using only a limited, standardized data set in-hospital mortality can be predicted satisfactorily at the time point of hospital admission. More parameters describing patient's health are likely needed to improve our model.
更多查看译文
关键词
prediction, in-hospital mortality, patient safety, machine learning
AI 理解论文
溯源树
样例
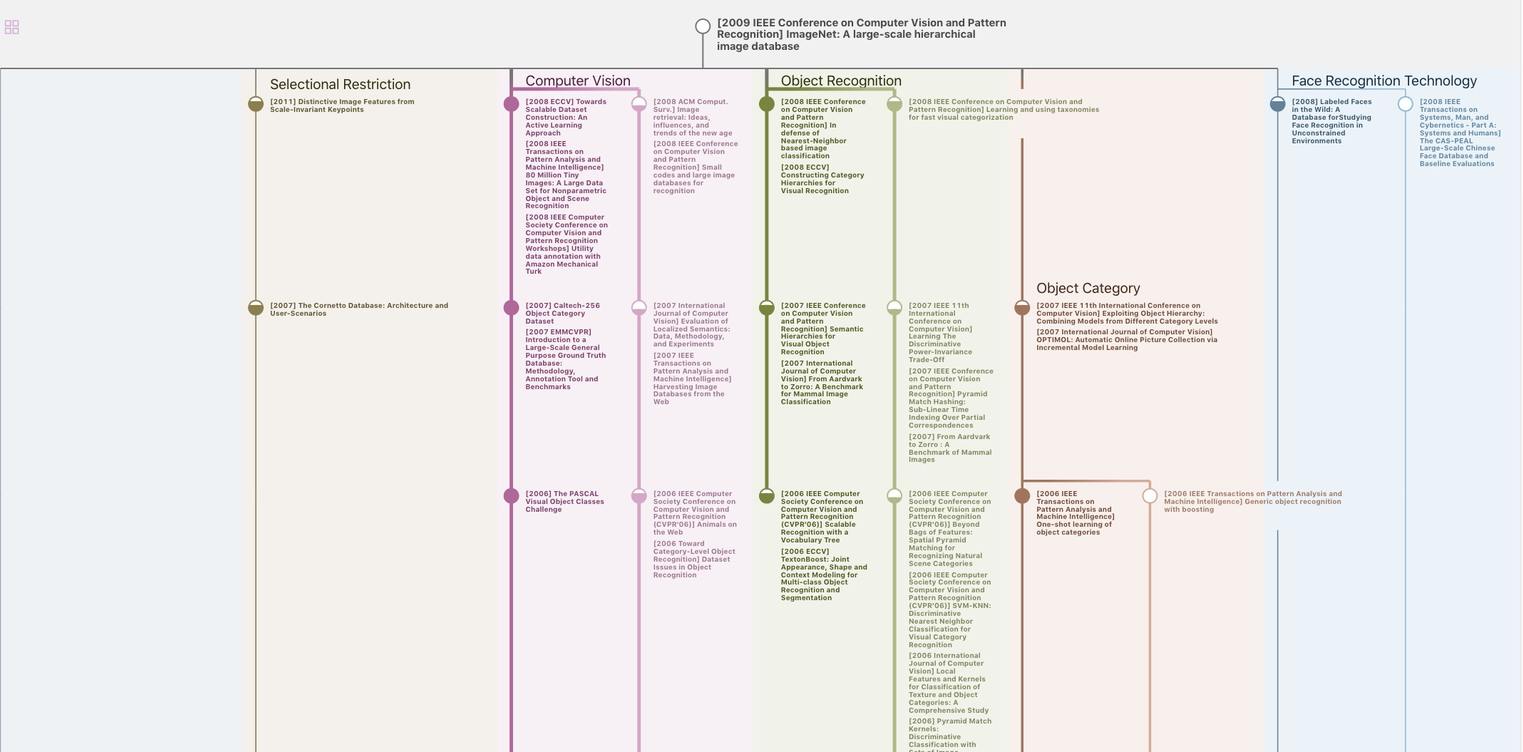
生成溯源树,研究论文发展脉络
Chat Paper
正在生成论文摘要