Pathways: Asynchronous Distributed Dataflow for ML.
Conference on Machine Learning and Systems (MLSys)(2022)
摘要
We present the design of a new large scale orchestration layer for accelerators. Our system, Pathways, is explicitly designed to enable exploration of new systems and ML research ideas, while retaining state of the art performance for current models. Pathways uses a sharded dataflow graph of asynchronous operators that consume and produce futures, and efficiently gang-schedules heterogeneous parallel computations on thousands of accelerators while coordinating data transfers over their dedicated interconnects. Pathways makes use of a novel asynchronous distributed dataflow design that lets the control plane execute in parallel despite dependencies in the data plane. This design, with careful engineering, allows Pathways to adopt a single-controller model that makes it easier to express complex new parallelism patterns. We demonstrate that Pathways can achieve performance parity (~100% accelerator utilization) with state-of-the-art systems when running SPMD computations over 2048 TPUs, while also delivering throughput comparable to the SPMD case for Transformer models that are pipelined across 16 stages, or sharded across two islands of accelerators connected over a data center network.
更多查看译文
关键词
dataflow,asynchronous,pathways,ml
AI 理解论文
溯源树
样例
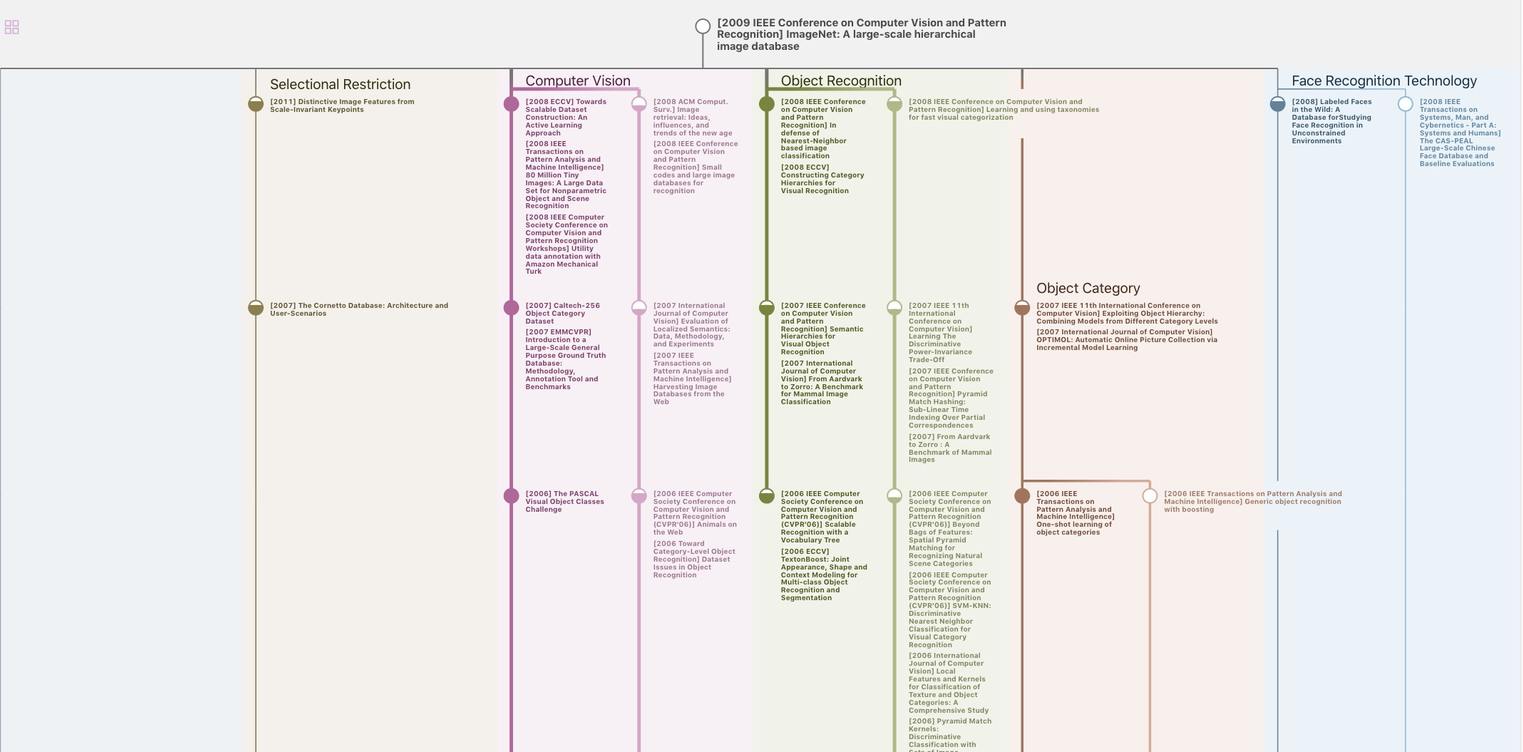
生成溯源树,研究论文发展脉络
Chat Paper
正在生成论文摘要