Learning the Dynamics of Autonomous Linear Systems From Multiple Trajectories.
ACC(2022)
摘要
We consider the problem of learning the dynamics of autonomous linear systems (i.e., systems that are not affected by external control inputs) from observations of multiple trajectories of those systems, with finite sample guarantees. Existing results on learning rate and consistency of autonomous linear system identification rely on observations of steady state behaviors from a single long trajectory, and are not applicable to unstable systems. In contrast, we consider the scenario of learning system dynamics based on multiple short trajectories, where there are no easily observed steady state behaviors. We provide a finite sample analysis, which shows that the dynamics can be learned at a rate $\mathcal{O}(\frac{1}{\sqrt{N}})$ for both stable and unstable systems, where $N$ is the number of trajectories, when the initial state of the system has zero mean (which is a common assumption in the existing literature). We further generalize our result to the case where the initial state has non-zero mean. We show that one can adjust the length of the trajectories to achieve a learning rate of $\mathcal{O}(\sqrt{\frac{\log{N}}{N})}$ for strictly stable systems and a learning rate of $\mathcal{O}(\frac{(\log{N})^d}{\sqrt{N}})$ for marginally stable systems, where $d$ is some constant.
更多查看译文
关键词
multiple trajectories,autonomous linear systems,dynamics,linear systems
AI 理解论文
溯源树
样例
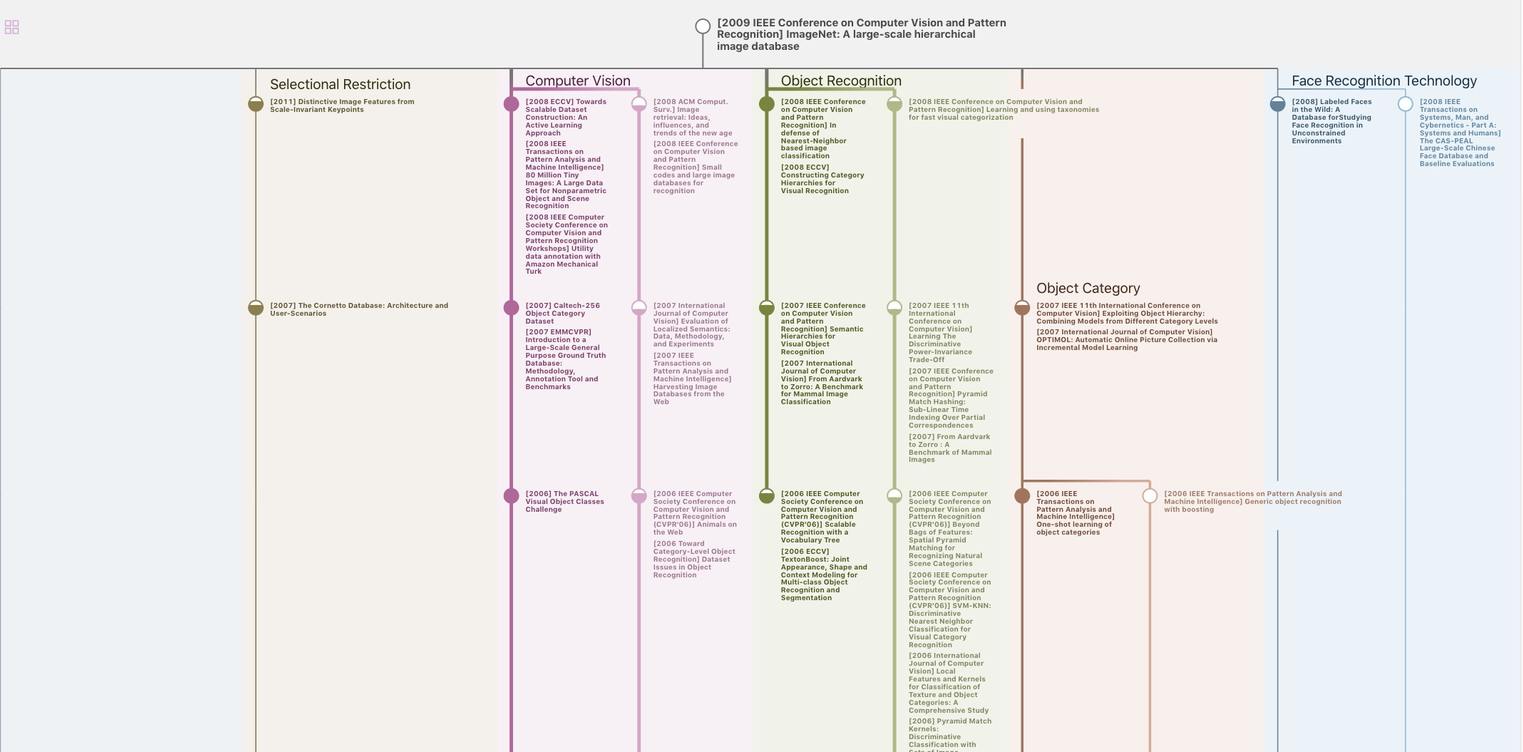
生成溯源树,研究论文发展脉络
Chat Paper
正在生成论文摘要