Quantification of Hepatocellular Mitoses in a Toxicological Study in Rats Using a Convolutional Neural Network
TOXICOLOGIC PATHOLOGY(2022)
摘要
Convolutional neural networks (CNNs) have been recognized as valuable tools for rapid quantitative analysis of morphological changes in toxicologic histopathology. We have assessed the performance of CNN-based (Halo-AI) mitotic figure detection in hepatocytes in comparison with detection by pathologists. In addition, we compared with Ki-67 and 5-bromodesoxyuridin (BrdU) immunohistochemistry labeling indices (LIs) obtained by image analysis. Tissues were from an exploratory toxicity study with a glycogen synthase kinase-3 (GSK-3) inhibitor. Our investigations revealed that (1) the CNN achieved similarly accurate but faster results than pathologists, (2) results of mitotic figure detection were comparable to Ki-67 and BrdU LIs, and (3) data from different methods were only moderately correlated. The latter is likely related to differences in the cell cycle component captured by each method. This highlights the importance of considering the differences of the available methods upon selection. Also, the pharmacology of our test item acting as a GSK-3 inhibitor potentially reduced the correlation. We conclude that hepatocyte cell proliferation assessment by CNNs can have several advantages when compared with the current gold standard: it relieves the pathologist of tedious routine tasks and contributes to standardization of results; the CNN algorithm can be shared and iteratively improved; it can be performed on routine histological slides; it does not require an additional animal experiment and in this way can contribute to animal welfare according to the 3R principles.
更多查看译文
关键词
convolutional neuronal networks, image analysis, liver, mitotic index, BrdU, Ki-67, glycogen synthase kinase-3 (GSK-3)
AI 理解论文
溯源树
样例
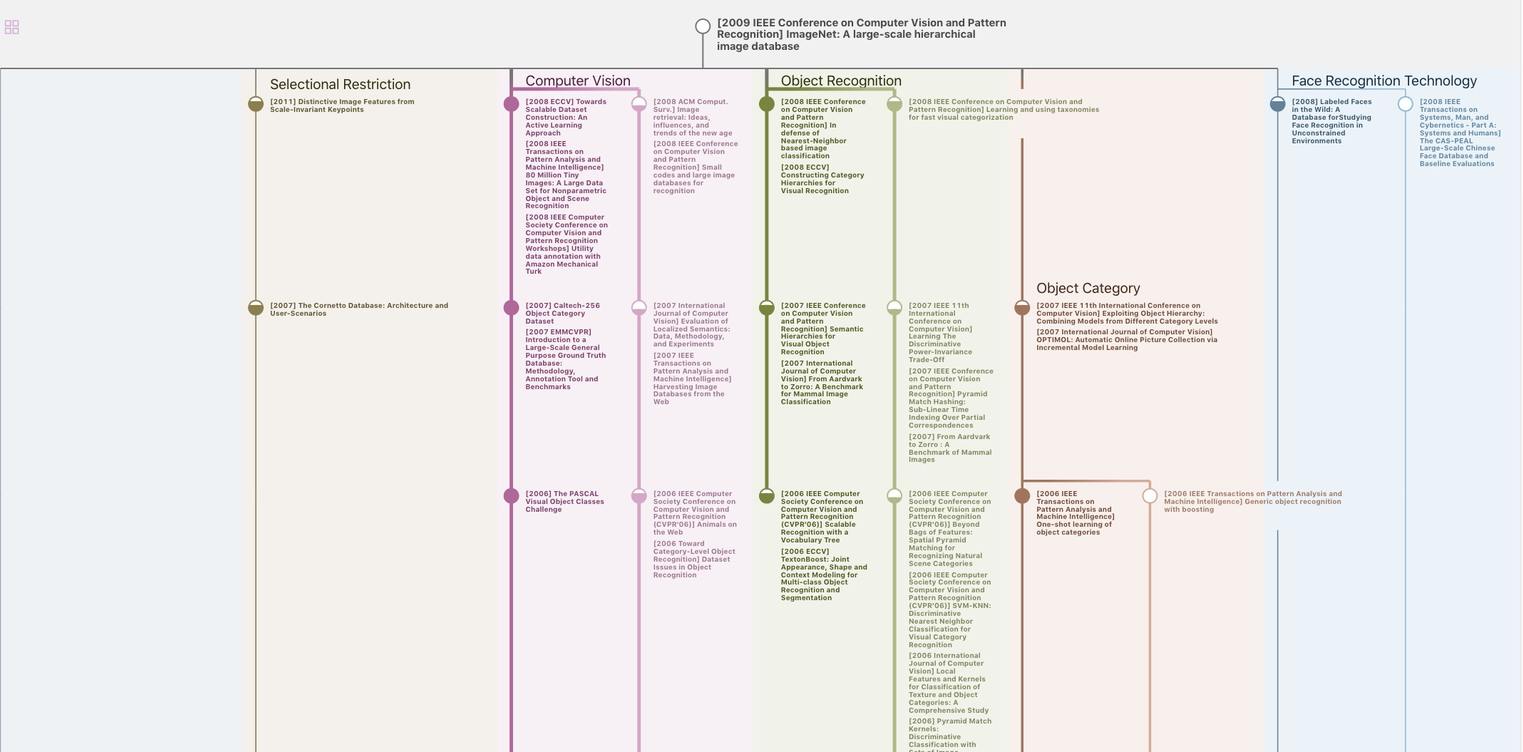
生成溯源树,研究论文发展脉络
Chat Paper
正在生成论文摘要