Automated Extraction of Pain Symptoms: A Natural Language Approach using Electronic Health Records.
Pain physician(2022)
摘要
BACKGROUND:Pain costs more than $600 billion annually and affects more than 100 million Americans, but is still a poorly understood problem and one for which there is very often limited effective treatment. Electronic health records (EHRs) are the only databases with a high volume of granular pain information that allows for documentation of detailed clinical notes on a patient's subjective experience.
OBJECTIVES:This study applied natural language processing (NLP) technology to an EHR dataset as part of a pilot study to capture pain information from clinical notes and prove its feasibility as an efficient method.
STUDY DESIGN:Retrospective study.
SETTING:All data were from UConn Health John Dempsey Hospital (JDH) in Farmington, CT.
METHODS:The JDH EHR dataset contains 611,355 clinical narratives from 359,854 patients from diverse demographic backgrounds from 2010 through 2019. These data were processed through a customized NLP pipeline. A training set of 100 notes was annotated based on focus group-generated ontology and used to generate and evaluate an NLP model that was later tested on the remaining notes. Validation of the model was evaluated externally and performance was analyzed.
RESULTS:The model identified back pain as the most common location of experienced pain with 40,369 term frequencies. Patients most commonly experienced decreased mobility with their pain with 7,375 term frequencies. Pain was most commonly found to be radiating with 26,967 term frequencies and patients most commonly rated their pain as 8/10 with 2,375 term frequencies. All parameters studied had statistical F-scores greater than 0.85.
LIMITATIONS:A single-center, pilot study subject to reporting bias, recording bias, and missing patient data.
CONCLUSIONS:Our customized NLP model demonstrated good and successful performance in extracting granular pain information from clinical notes in electronic health records.
更多查看译文
AI 理解论文
溯源树
样例
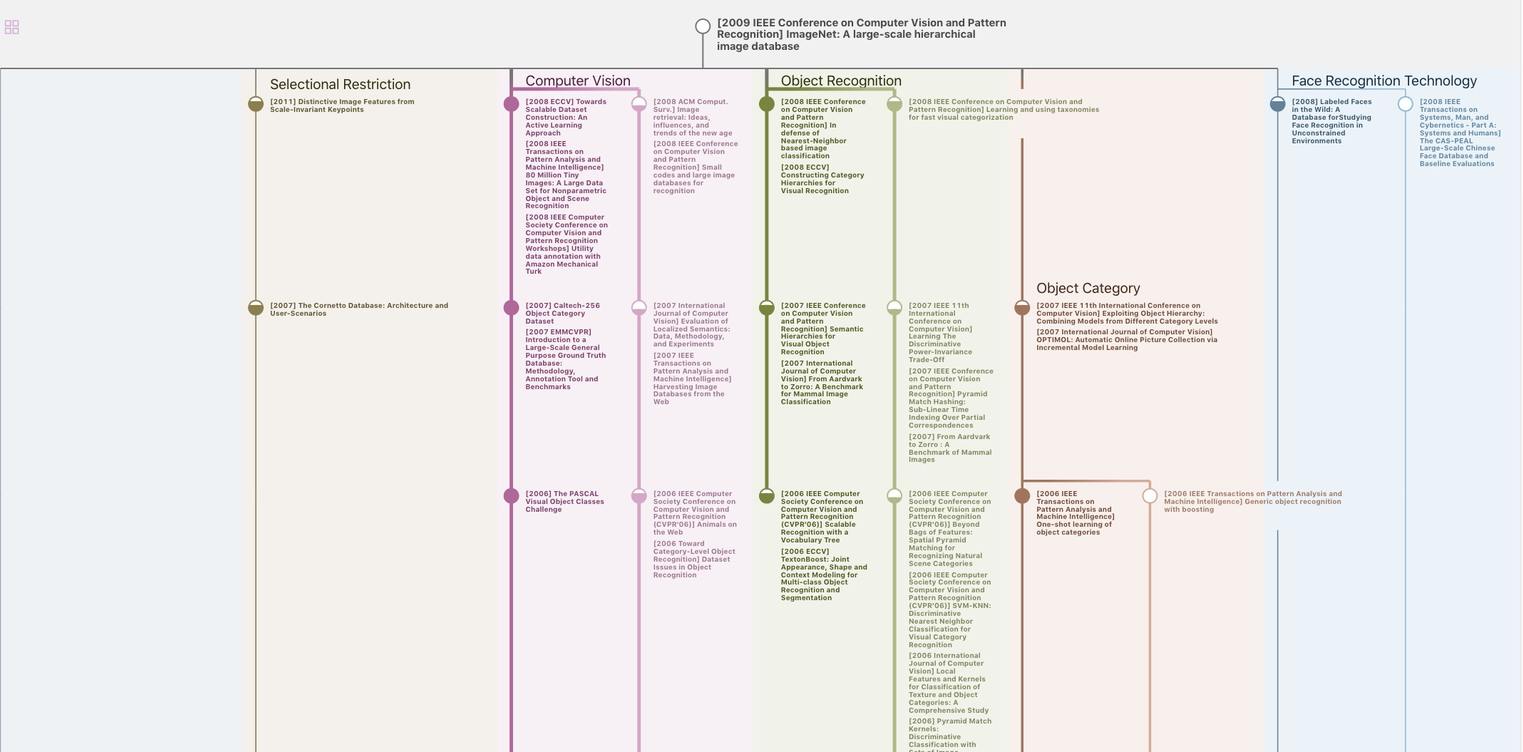
生成溯源树,研究论文发展脉络
Chat Paper
正在生成论文摘要