Towards a New Qualitative Screening Assay for Synthetic Cannabinoids Using Metabolomics and Machine Learning
CLINICAL CHEMISTRY(2022)
摘要
Background Synthetic cannabinoids (SCs) are steadily emerging on the drug market. To remain competitive in clinical or forensic toxicology, new screening strategies including high-resolution mass spectrometry (HRMS) are required. Machine learning algorithms can detect and learn chemical signatures in complex datasets and use them as a proxy to predict new samples. We propose a new screening tool based on a SC-specific change of the metabolome and a machine learning algorithm. Methods Authentic human urine samples (n = 474), positive or negative for SCs, were used. These samples were measured with an untargeted metabolomics liquid chromatography (LC)-quadrupole time-of-flight-HRMS method. Progenesis QI software was used to preprocess the raw data. Following feature engineering, a random forest (RF) model was optimized in R using a 10-fold cross-validation method and a training set (n = 369). The performance of the model was assessed with a test (n = 50) and a verification (n = 55) set. Results During RF optimization, 49 features, 200 trees, and 7 variables at each branching node were determined as most predictive. The optimized model accuracy, clinical sensitivity, clinical specificity, positive predictive value, and negative predictive value were 88.1%, 83.0%, 92.7%, 91.3%, and 85.6%, respectively. The test set was predicted with an accuracy of 88.0%, and the verification set provided evidence that the model was able to detect cannabinoid-specific changes in the metabolome. Conclusions An RF approach combined with metabolomics enables a novel screening strategy for responding effectively to the challenge of new SCs. Biomarkers identified by this approach may also be integrated in routine screening methods.
更多查看译文
关键词
synthetic cannabinoids,metabolomics,random forests,urine screening
AI 理解论文
溯源树
样例
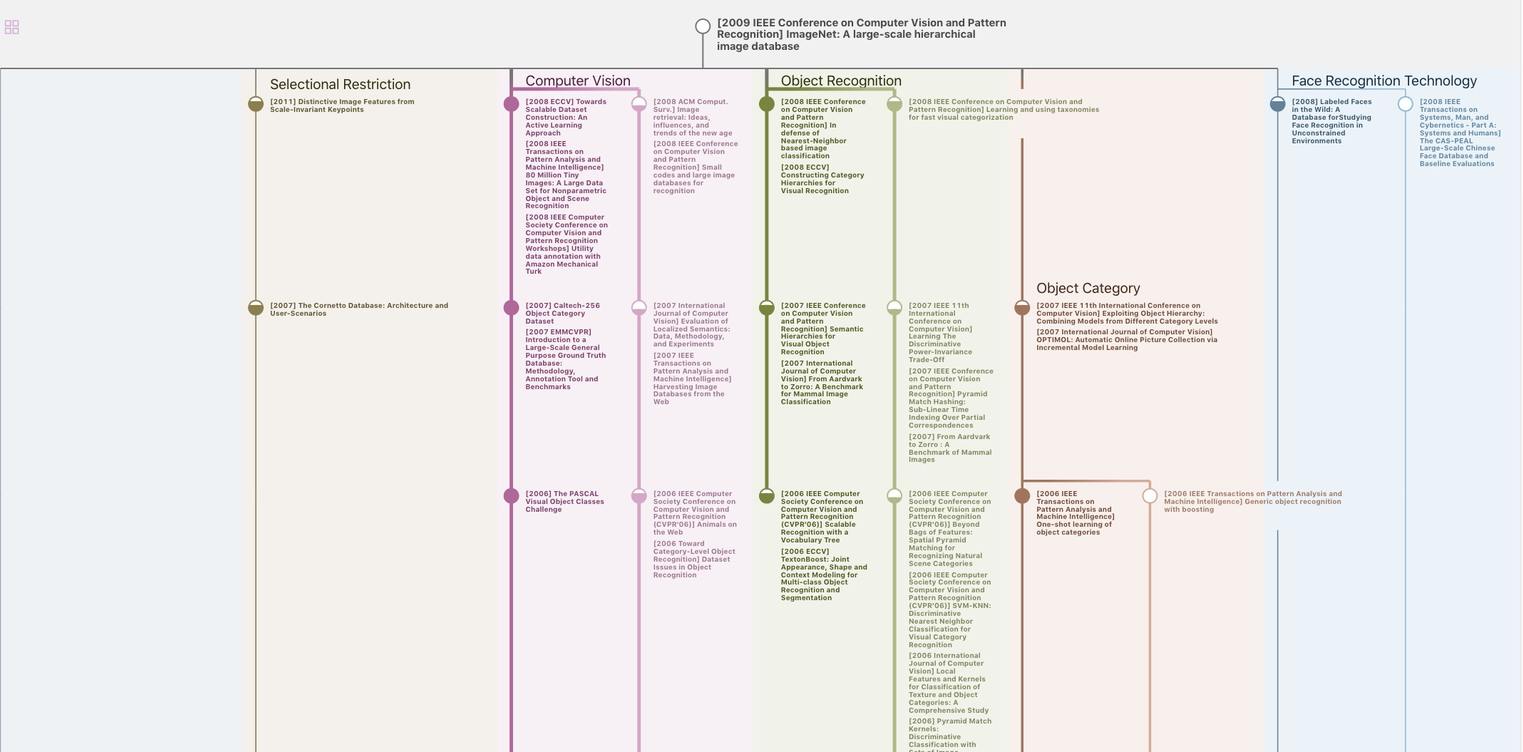
生成溯源树,研究论文发展脉络
Chat Paper
正在生成论文摘要