Solving Inverse Electrocardiographic Mapping Using Machine Learning and Deep Learning Frameworks
Sensors(2022)
摘要
Electrocardiographic imaging (ECGi) reconstructs electrograms at the heart’s surface using the potentials recorded at the body’s surface. This is called the inverse problem of electrocardiography. This study aimed to improve on the current solution methods using machine learning and deep learning frameworks. Electrocardiograms were simultaneously recorded from pigs’ ventricles and their body surfaces. The Fully Connected Neural network (FCN), Long Short-term Memory (LSTM), Convolutional Neural Network (CNN) methods were used for constructing the model. A method is developed to align the data across different pigs. We evaluated the method using leave-one-out cross-validation. For the best result, the overall median of the correlation coefficient of the predicted ECG wave was 0.74. This study demonstrated that a neural network can be used to solve the inverse problem of ECGi with relatively small datasets, with an accuracy compatible with current standard methods.
更多查看译文
关键词
electrocardiographic imaging (ECGi),deep learning,machine learning,inverse problem,Fully Connected Neural network (FCN),Long Short-term Memory (LSTM),Convolutional Neural Network (CNN)
AI 理解论文
溯源树
样例
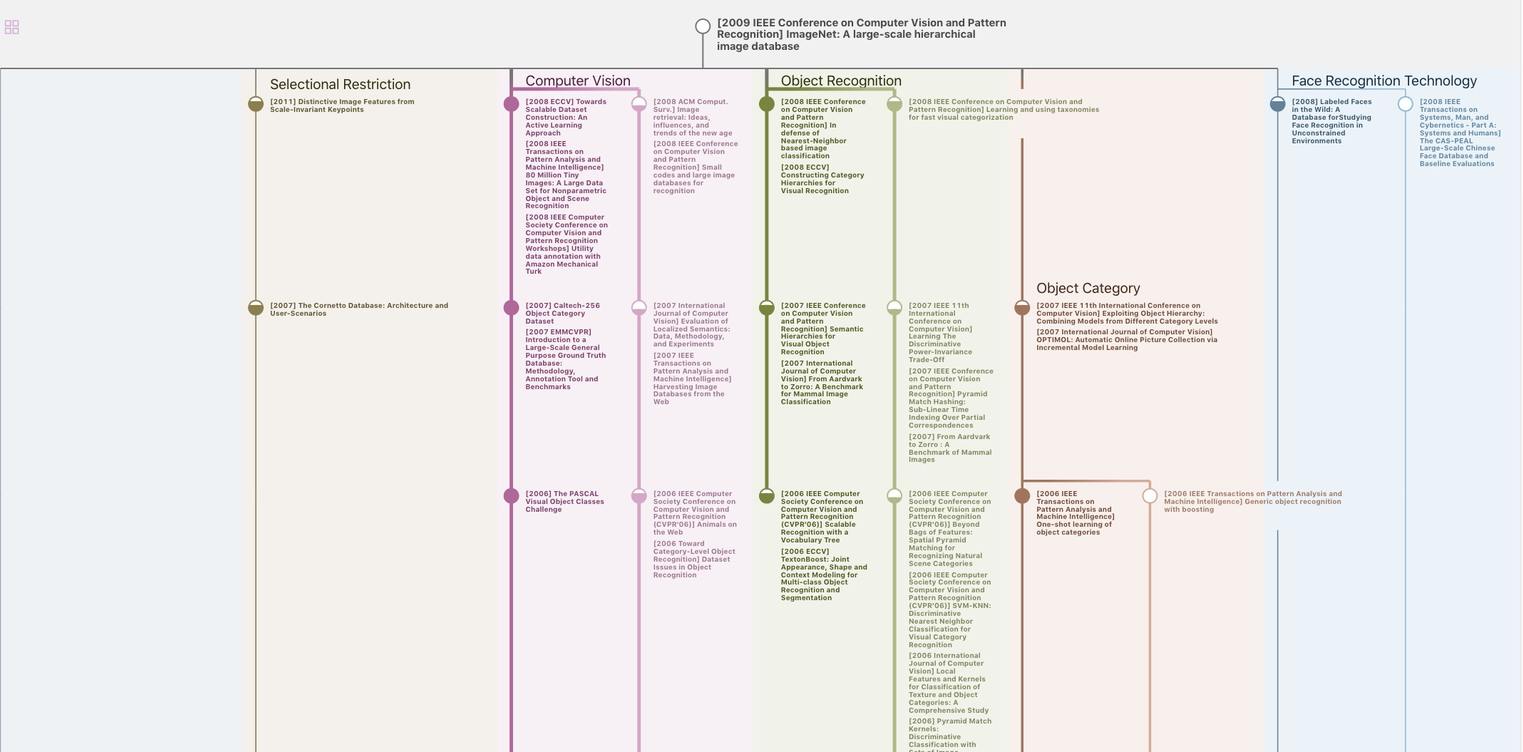
生成溯源树,研究论文发展脉络
Chat Paper
正在生成论文摘要