Chaos is a Ladder: A New Theoretical Understanding of Contrastive Learning via Augmentation Overlap
International Conference on Learning Representations (ICLR)(2022)
摘要
Recently, contrastive learning has risen to be a promising approach for large-scale self-supervised learning. However, theoretical understanding of how it works is still unclear. In this paper, we propose a new guarantee on the downstream performance without resorting to the conditional independence assumption that is widely adopted in previous work but hardly holds in practice. Our new theory hinges on the insight that the support of different intra-class samples will become more overlapped under aggressive data augmentations, thus simply aligning the positive samples (augmented views of the same sample) could make contrastive learning cluster intra-class samples together. Based on this augmentation overlap perspective, theoretically, we obtain asymptotically closed bounds for downstream performance under weaker assumptions, and empirically, we propose an unsupervised model selection metric ARC that aligns well with downstream accuracy. Our theory suggests an alternative understanding of contrastive learning: the role of aligning positive samples is more like a surrogate task than an ultimate goal, and the overlapped augmented views (i.e., the chaos) create a ladder for contrastive learning to gradually learn class-separated representations. The code for computing ARC is available at https://github.com/zhangq327/ARC.
更多查看译文
关键词
Contrastive Learning,Representation Learning,Self-supervised Learning
AI 理解论文
溯源树
样例
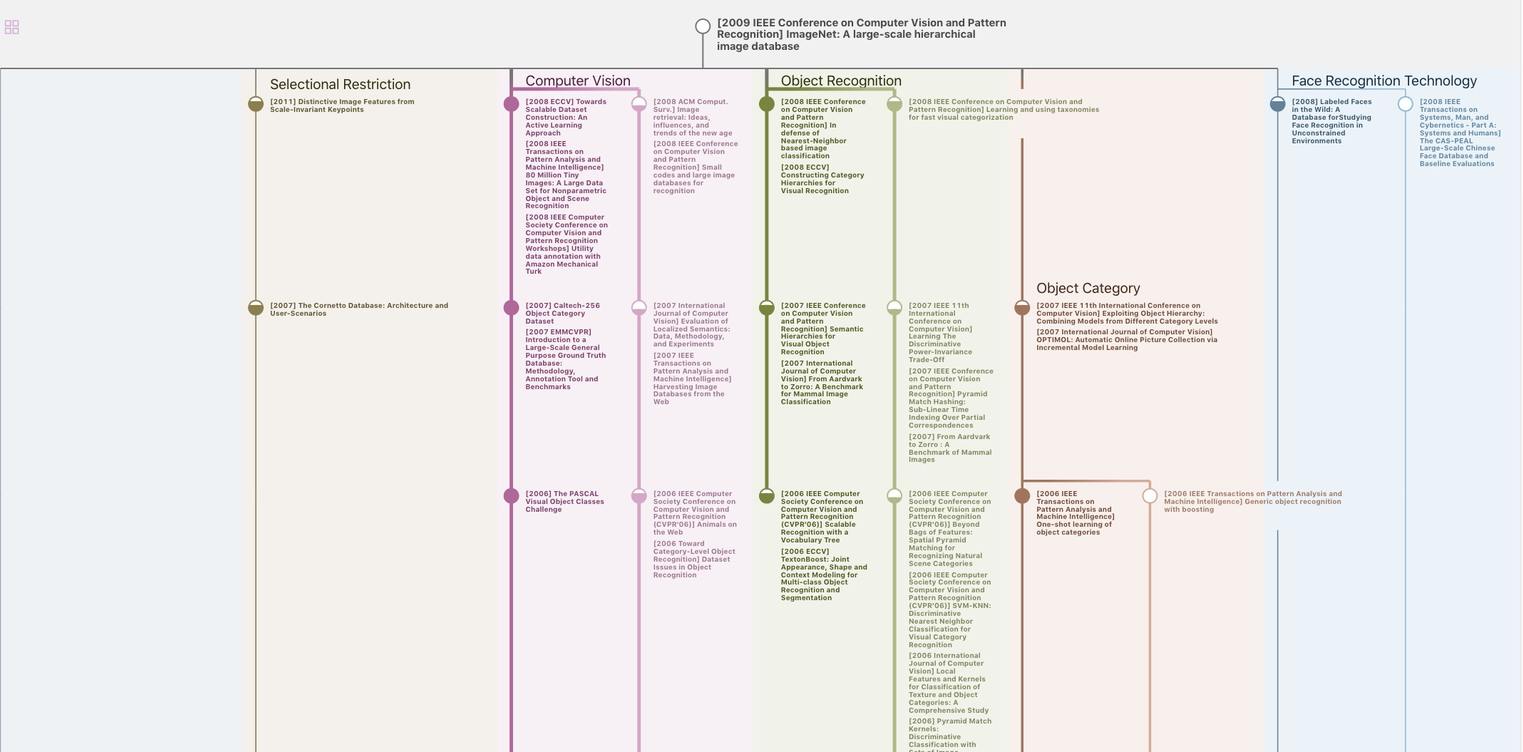
生成溯源树,研究论文发展脉络
Chat Paper
正在生成论文摘要