Learning Deep Implicit Functions for 3D Shapes with Dynamic Code Clouds
2022 IEEE/CVF Conference on Computer Vision and Pattern Recognition (CVPR)(2022)
摘要
Deep Implicit Function (DIF) has gained popularity as an efficient 3D shape representation. To capture geometry details, current methods usually learn DIF using local latent codes, which discretize the space into a regular 3D grid (or octree) and store local codes in grid points (or octree nodes). Given a query point, the local feature is computed by interpolating its neighboring local codes with their positions. However, the local codes are constrained at discrete and regular positions like grid points, which makes the code positions difficult to be optimized and limits their representation ability. To solve this problem, we propose to learn DIF with Dynamic Code Cloud, named DCC-DIF. Our method explicitly associates local codes with learnable position vectors, and the position vectors are continuous and can be dynamically optimized, which improves the representation ability. In addition, we propose a novel code position loss to optimize the code positions, which heuristically guides more local codes to be distributed around complex geometric details. In contrast to previous methods, our DCC-DIF represents 3D shapes more efficiently with a small amount of local codes, and improves the reconstruction quality. Experiments demonstrate that DCC-DIF achieves better performance over previous methods. Code and data are available at https://github.com/lity20/DCCDIF.
更多查看译文
关键词
3D from multi-view and sensors
AI 理解论文
溯源树
样例
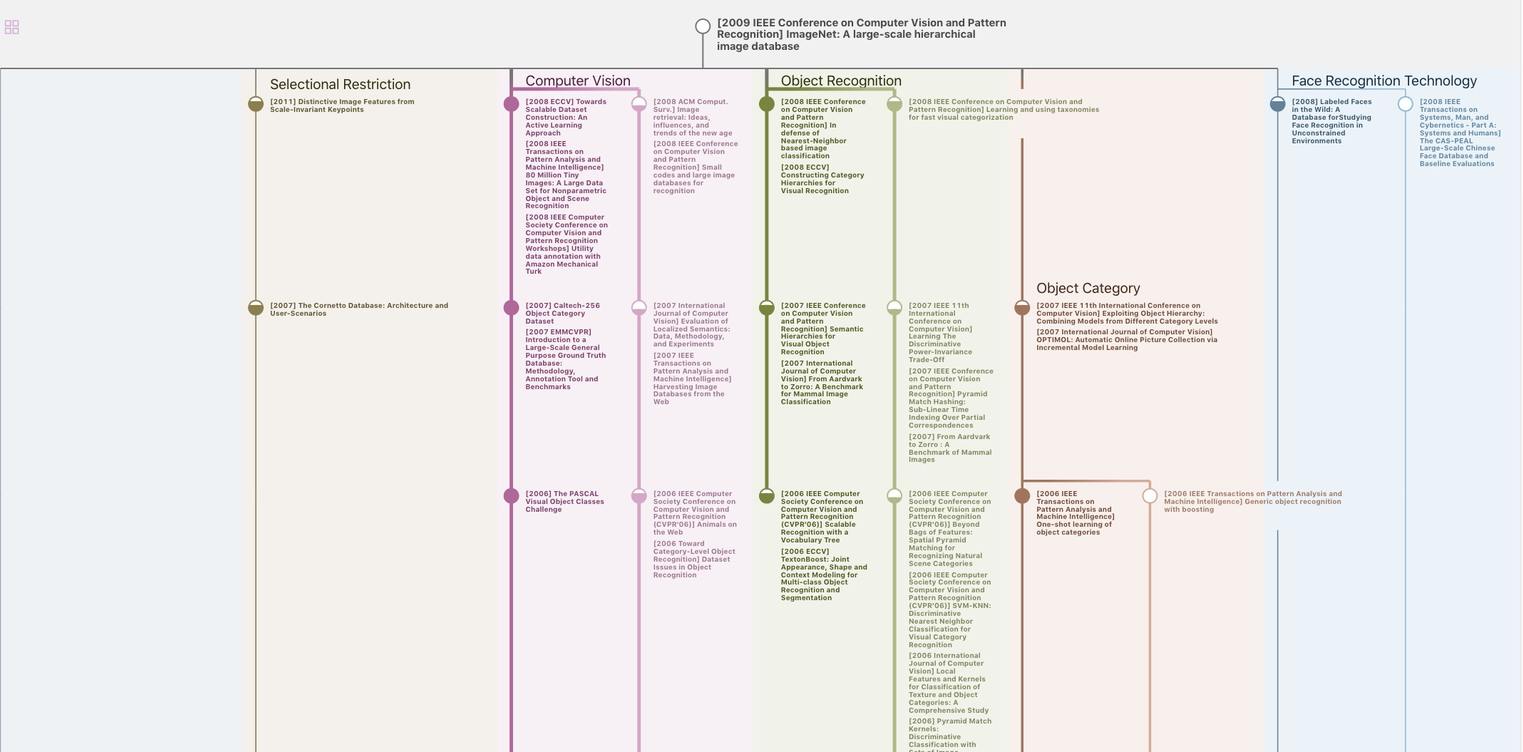
生成溯源树,研究论文发展脉络
Chat Paper
正在生成论文摘要