Reinforcement Guided Multi-Task Learning Framework for Low-Resource Stereotype Detection
PROCEEDINGS OF THE 60TH ANNUAL MEETING OF THE ASSOCIATION FOR COMPUTATIONAL LINGUISTICS (ACL 2022), VOL 1: (LONG PAPERS)(2022)
摘要
As large Pre-trained Language Models (PLMs) trained on large amounts of data in an unsupervised manner become more ubiquitous, identifying various types of bias in the text has come into sharp focus. Existing 'Stereotype Detection' datasets mainly adopt a diagnostic approach toward large PLMs. Blodgett et al. (2021a) show that there are significant reliability issues with the existing benchmark datasets. Annotating a reliable dataset requires a precise understanding of the subtle nuances of how stereotypes manifest in text. In this paper, we annotate a focused evaluation set for 'Stereotype Detection' that addresses those pitfalls by de-constructing various ways in which stereotypes manifest in text. Further, we present a multi-task model that leverages the abundance of data-rich neighboring tasks such as hate speech detection, offensive language detection, misogyny detection, etc., to improve the empirical performance on 'Stereotype Detection'. We then propose a reinforcement-learning agent that guides the multi-task learning model by learning to identify the training examples from the neighboring tasks that help the target task the most. We show that the proposed models achieve significant empirical gains over existing baselines on all the tasks.
更多查看译文
关键词
learning,multi-task,low-resource
AI 理解论文
溯源树
样例
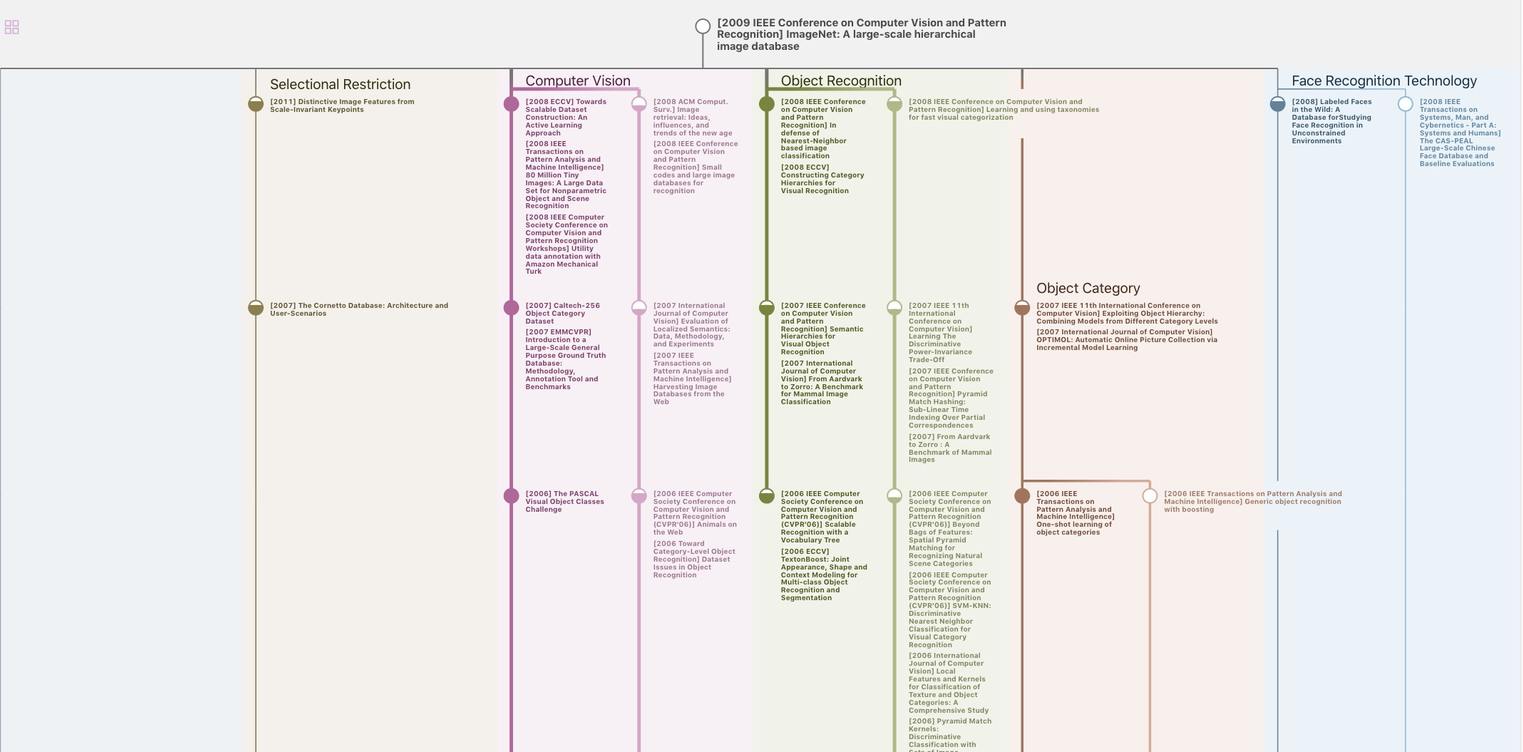
生成溯源树,研究论文发展脉络
Chat Paper
正在生成论文摘要