ANNA: Enhanced Language Representation for Question Answering
PROCEEDINGS OF THE 7TH WORKSHOP ON REPRESENTATION LEARNING FOR NLP(2022)
摘要
Pre-trained language models have brought significant improvements in performance in a variety of natural language processing tasks. Most existing models performing state-of-theart results have shown their approaches in the separate perspectives of data processing, pretraining tasks, neural network modeling, or fine-tuning. In this paper, we demonstrate how the approaches affect performance individually, and that the language model performs the best results on a specific question answering task when those approaches are jointly considered in pre-training models. In particular, we propose an extended pre-training task, and a new neighbor-aware mechanism that attends neighboring tokens more to capture the richness of context for pre-training language modeling. Our best model achieves new state-of-the-art results of 95.7% F1 and 90.6% EM on SQuAD 1.1 and also outperforms existing pre-trained language models such as RoBERTa, ALBERT, ELECTRA, and XLNet on the SQuAD 2.0 benchmark.
更多查看译文
关键词
enhanced language representation
AI 理解论文
溯源树
样例
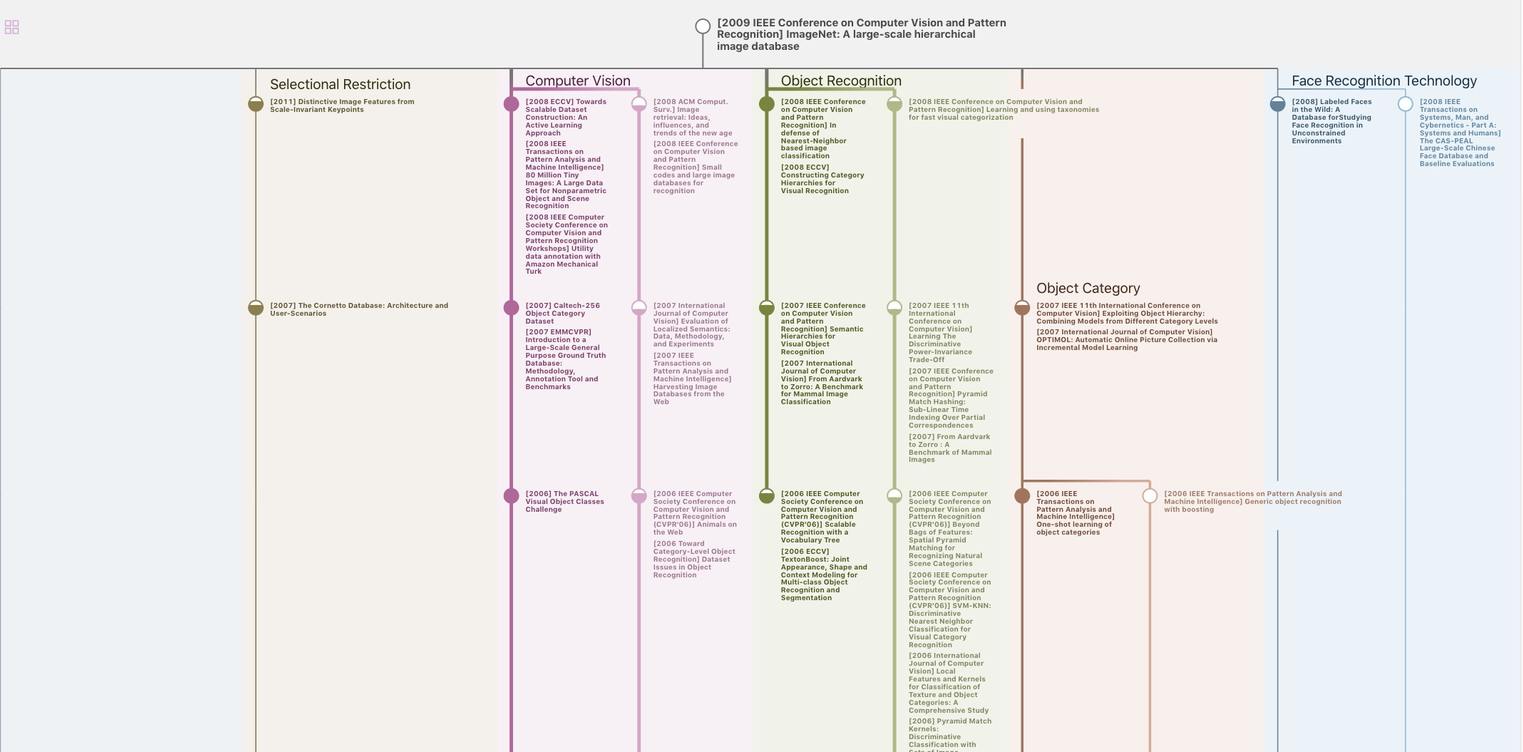
生成溯源树,研究论文发展脉络
Chat Paper
正在生成论文摘要