Brain-inspired Multilayer Perceptron with Spiking Neurons
2022 IEEE/CVF Conference on Computer Vision and Pattern Recognition (CVPR)(2022)
摘要
Recently, Multilayer Perceptron (MLP) becomes the hotspot in the field of computer vision tasks. Without in-ductive bias, MLPs perform well on feature extraction and achieve amazing results. However, due to the simplic-ity of their structures, the performance highly depends on the local features communication machenism. To further improve the performance of MLP, we introduce information communication mechanisms from brain-inspired neu-ral networks. Spiking Neural Network (SNN) is the most famous brain-inspired neural network, and achieve great success on dealing with sparse data. Leaky Integrate and Fire (LIF) neurons in SNNs are used to communicate be-tween different time steps. In this paper, we incorporate the machanism of LIF neurons into the MLP models, to achieve better accuracy without extra FLOPs. We pro-pose a full-precision LIF operation to communicate be-tween patches, including horizontal LIF and vertical LIF in different directions. We also propose to use group LIF to extract better local features. With LIF modules, our SNN-MLP model achieves 81.9%, 83.3% and 83.5% top-1 accuracy on ImageNet dataset with only 4.4G, 8.5G and 15.2G FLOPs, respectively, which are state-of-the-art re-sults as far as we know. The source code will be available at https://gitee.com/mindspore/models/tree/master/research/cv/snn_mlp.
更多查看译文
关键词
Deep learning architectures and techniques, Biometrics, Machine learning
AI 理解论文
溯源树
样例
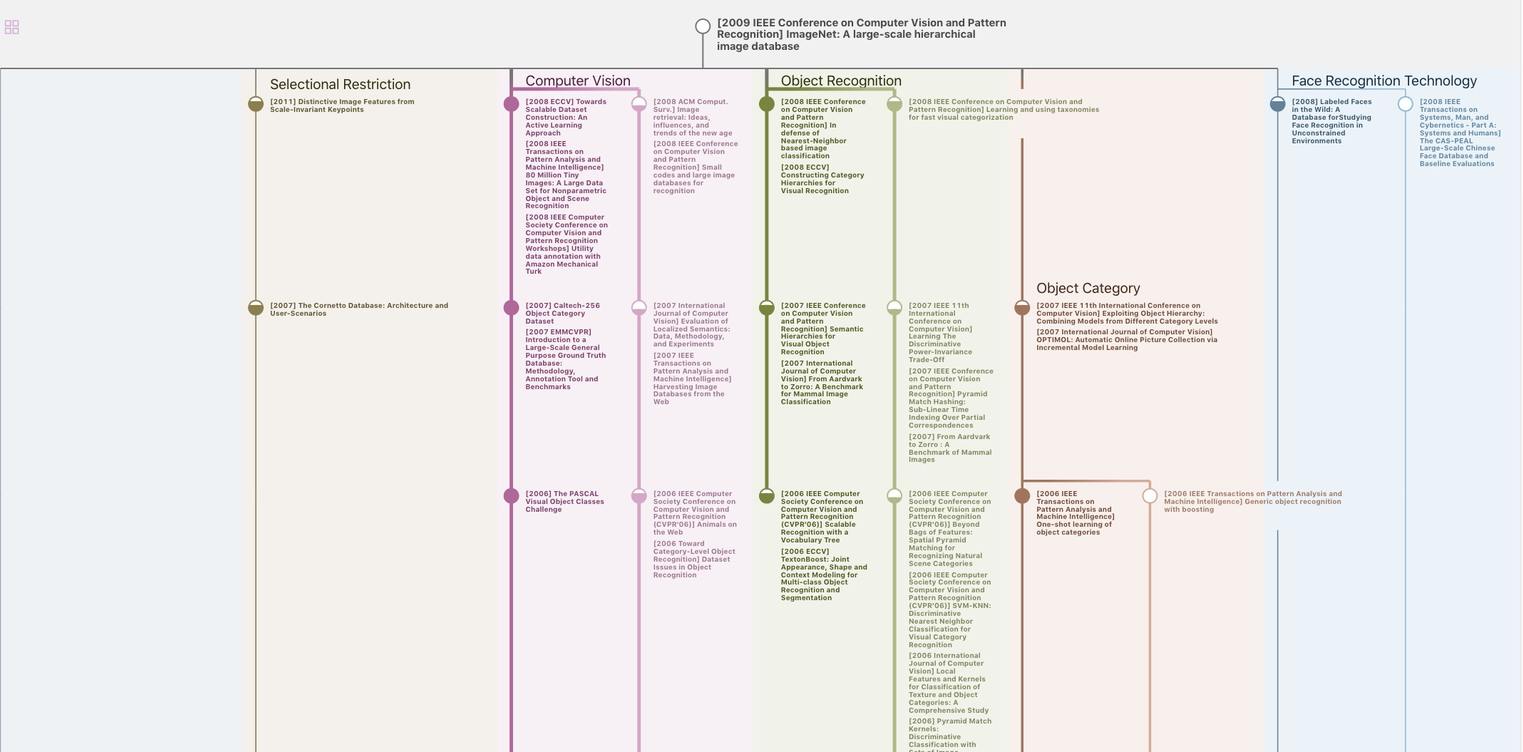
生成溯源树,研究论文发展脉络
Chat Paper
正在生成论文摘要