A Bayesian Hierarchical Spatial Model to Correct for Misreporting in Count Data: Application to State-Level COVID-19 Data in the United States
INTERNATIONAL JOURNAL OF ENVIRONMENTAL RESEARCH AND PUBLIC HEALTH(2022)
摘要
The COVID-19 pandemic that began at the end of 2019 has caused hundreds of millions of infections and millions of deaths worldwide. COVID-19 posed a threat to human health and profoundly impacted the global economy and people's lifestyles. The United States is one of the countries severely affected by the disease. Evidence shows that the spread of COVID-19 was significantly underestimated in the early stages, which prevented governments from adopting effective interventions promptly to curb the spread of the disease. This paper adopts a Bayesian hierarchical model to study the under-reporting of COVID-19 at the state level in the United States as of the end of April 2020. The model examines the effects of different covariates on the under-reporting and accurate incidence rates and considers spatial dependency. In addition to under-reporting (false negatives), we also explore the impact of over-reporting (false positives). Adjusting for misclassification requires adding additional parameters that are not directly identified by the observed data. Informative priors are required. We discuss prior elicitation and include R functions that convert expert information into the appropriate prior distribution.
更多查看译文
关键词
COVID-19, under-reporting, Bayesian, spatial, over-reporting
AI 理解论文
溯源树
样例
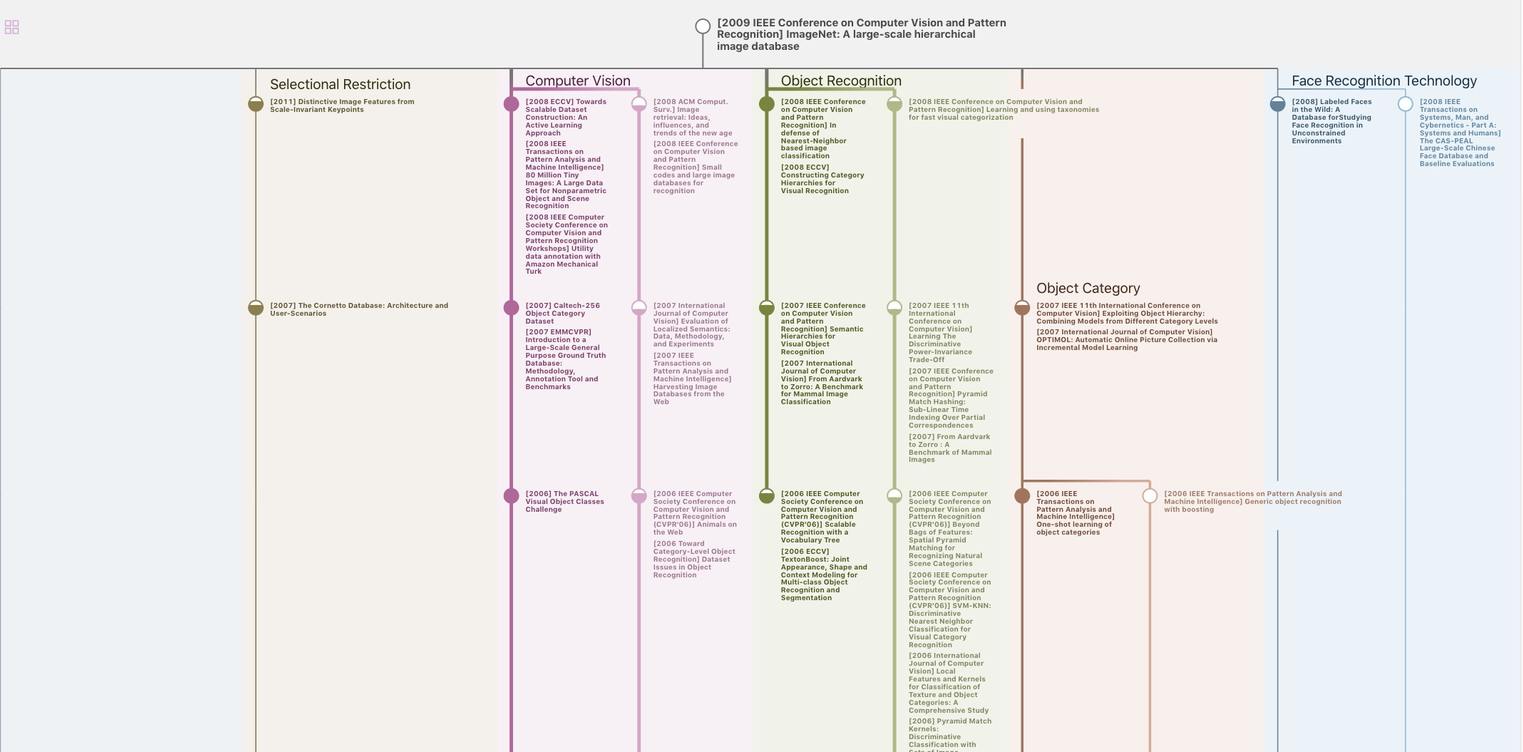
生成溯源树,研究论文发展脉络
Chat Paper
正在生成论文摘要